2022-07-30 更新
High-fidelity GAN Inversion with Padding Space
Authors:Qingyan Bai, Yinghao Xu, Jiapeng Zhu, Weihao Xia, Yujiu Yang, Yujun Shen
Inverting a Generative Adversarial Network (GAN) facilitates a wide range of image editing tasks using pre-trained generators. Existing methods typically employ the latent space of GANs as the inversion space yet observe the insufficient recovery of spatial details. In this work, we propose to involve the padding space of the generator to complement the latent space with spatial information. Concretely, we replace the constant padding (e.g., usually zeros) used in convolution layers with some instance-aware coefficients. In this way, the inductive bias assumed in the pre-trained model can be appropriately adapted to fit each individual image. Through learning a carefully designed encoder, we manage to improve the inversion quality both qualitatively and quantitatively, outperforming existing alternatives. We then demonstrate that such a space extension barely affects the native GAN manifold, hence we can still reuse the prior knowledge learned by GANs for various downstream applications. Beyond the editing tasks explored in prior arts, our approach allows a more flexible image manipulation, such as the separate control of face contour and facial details, and enables a novel editing manner where users can customize their own manipulations highly efficiently.
PDF ECCV 2022 camera-ready; Project page: https://ezioby.github.io/padinv/; Code: https://github.com/EzioBy/padinv
点此查看论文截图
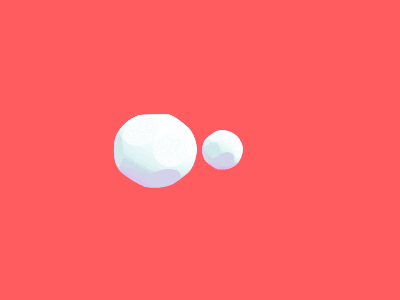
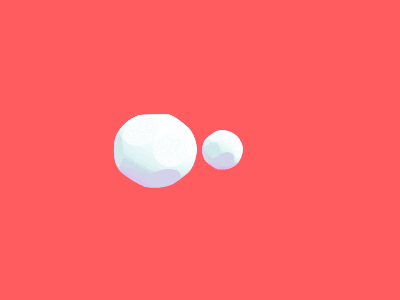
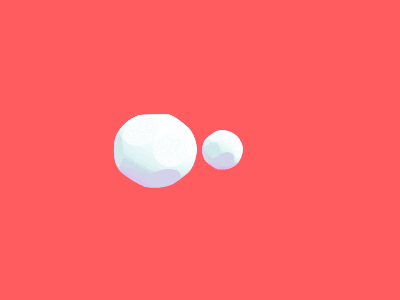
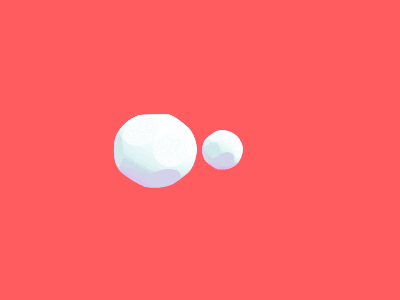
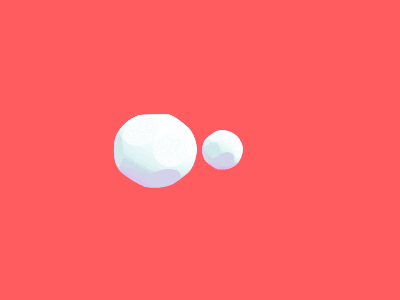
Stable Parallel Training of Wasserstein Conditional Generative Adversarial Neural Networks
Authors:Massimiliano Lupo Pasini, Junqi Yin
We propose a stable, parallel approach to train Wasserstein Conditional Generative Adversarial Neural Networks (W-CGANs) under the constraint of a fixed computational budget. Differently from previous distributed GANs training techniques, our approach avoids inter-process communications, reduces the risk of mode collapse and enhances scalability by using multiple generators, each one of them concurrently trained on a single data label. The use of the Wasserstein metric also reduces the risk of cycling by stabilizing the training of each generator. We illustrate the approach on the CIFAR10, CIFAR100, and ImageNet1k datasets, three standard benchmark image datasets, maintaining the original resolution of the images for each dataset. Performance is assessed in terms of scalability and final accuracy within a limited fixed computational time and computational resources. To measure accuracy, we use the inception score, the Frechet inception distance, and image quality. An improvement in inception score and Frechet inception distance is shown in comparison to previous results obtained by performing the parallel approach on deep convolutional conditional generative adversarial neural networks (DC-CGANs) as well as an improvement of image quality of the new images created by the GANs approach. Weak scaling is attained on both datasets using up to 2,000 NVIDIA V100 GPUs on the OLCF supercomputer Summit.
PDF 22 pages; 9 figures
点此查看论文截图
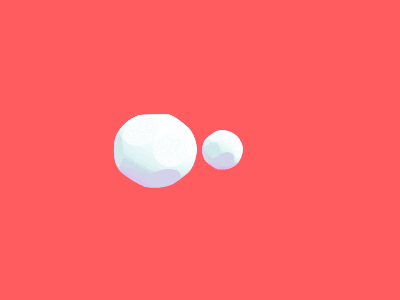
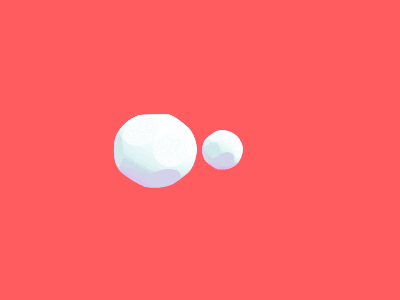
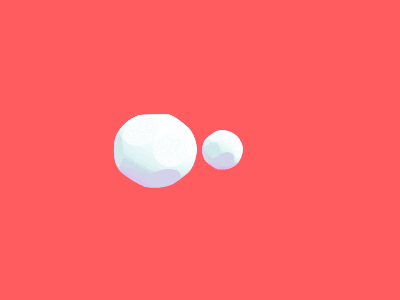
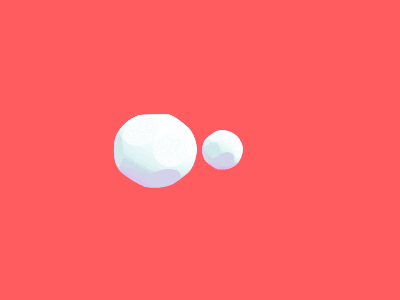
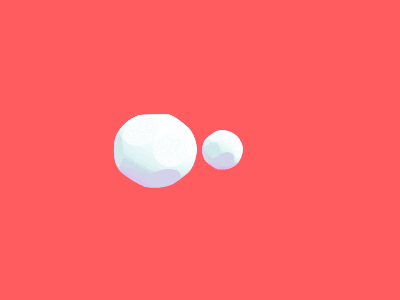