2022-07-29 更新
Look Closer to Your Enemy: Learning to Attack via Teacher-student Mimicking
Authors:Mingejie Wang, Zhiqing Tang, Sirui Li, Dingwen Xiao
This paper aims to generate realistic attack samples of person re-identification, ReID, by reading the enemy’s mind (VM). In this paper, we propose a novel inconspicuous and controllable ReID attack baseline, LCYE, to generate adversarial query images. Concretely, LCYE first distills VM’s knowledge via teacher-student memory mimicking in the proxy task. Then this knowledge prior acts as an explicit cipher conveying what is essential and realistic, believed by VM, for accurate adversarial misleading. Besides, benefiting from the multiple opposing task framework of LCYE, we further investigate the interpretability and generalization of ReID models from the view of the adversarial attack, including cross-domain adaption, cross-model consensus, and online learning process. Extensive experiments on four ReID benchmarks show that our method outperforms other state-of-the-art attackers with a large margin in white-box, black-box, and target attacks. Our code is now available at https://gitfront.io/r/user-3704489/mKXusqDT4ffr/LCYE/.
PDF 13 pages, 8 figures, NDSS
点此查看论文截图
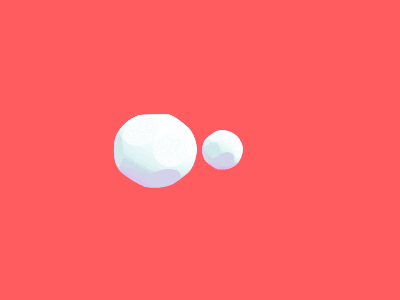
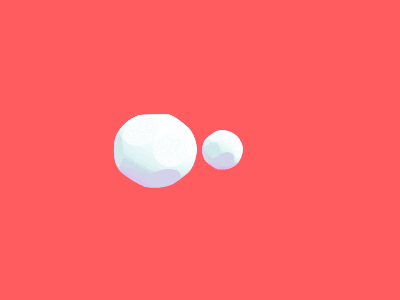
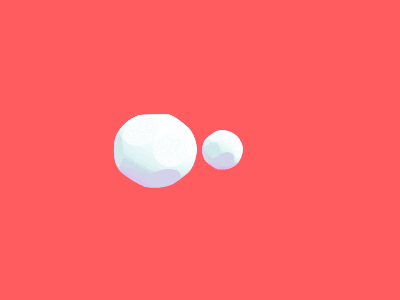
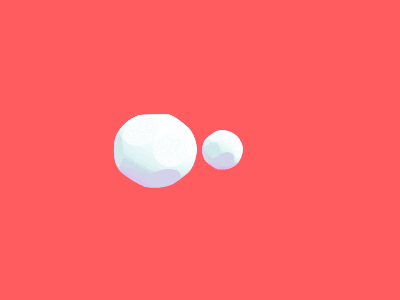
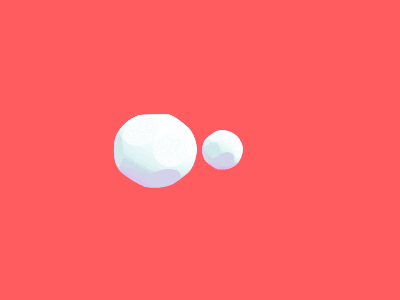
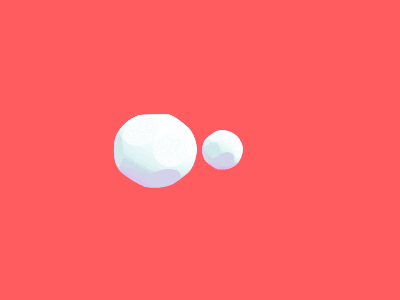
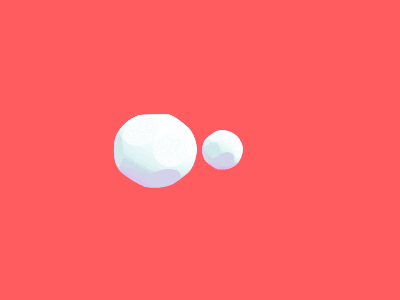
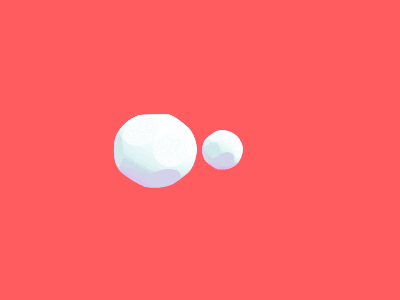
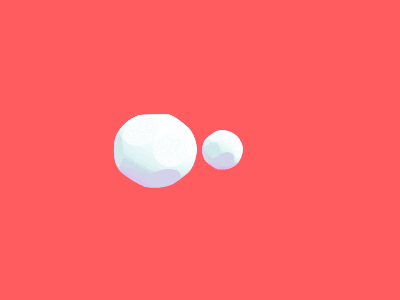
TnT Attacks! Universal Naturalistic Adversarial Patches Against Deep Neural Network Systems
Authors:Bao Gia Doan, Minhui Xue, Shiqing Ma, Ehsan Abbasnejad, Damith C. Ranasinghe
Deep neural networks are vulnerable to attacks from adversarial inputs and, more recently, Trojans to misguide or hijack the model’s decision. We expose the existence of an intriguing class of spatially bounded, physically realizable, adversarial examples — Universal NaTuralistic adversarial paTches — we call TnTs, by exploring the superset of the spatially bounded adversarial example space and the natural input space within generative adversarial networks. Now, an adversary can arm themselves with a patch that is naturalistic, less malicious-looking, physically realizable, highly effective achieving high attack success rates, and universal. A TnT is universal because any input image captured with a TnT in the scene will: i) misguide a network (untargeted attack); or ii) force the network to make a malicious decision (targeted attack). Interestingly, now, an adversarial patch attacker has the potential to exert a greater level of control — the ability to choose a location-independent, natural-looking patch as a trigger in contrast to being constrained to noisy perturbations — an ability is thus far shown to be only possible with Trojan attack methods needing to interfere with the model building processes to embed a backdoor at the risk discovery; but, still realize a patch deployable in the physical world. Through extensive experiments on the large-scale visual classification task, ImageNet with evaluations across its entire validation set of 50,000 images, we demonstrate the realistic threat from TnTs and the robustness of the attack. We show a generalization of the attack to create patches achieving higher attack success rates than existing state-of-the-art methods. Our results show the generalizability of the attack to different visual classification tasks (CIFAR-10, GTSRB, PubFig) and multiple state-of-the-art deep neural networks such as WideResnet50, Inception-V3 and VGG-16.
PDF Accepted for publication in the IEEE Transactions on Information Forensics & Security (TIFS)
点此查看论文截图
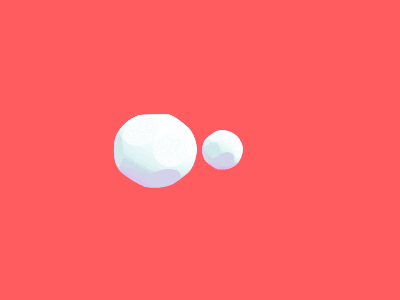
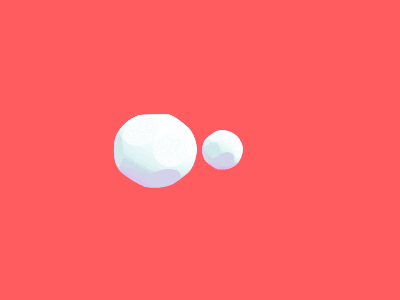
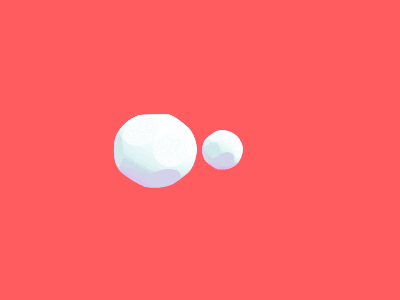
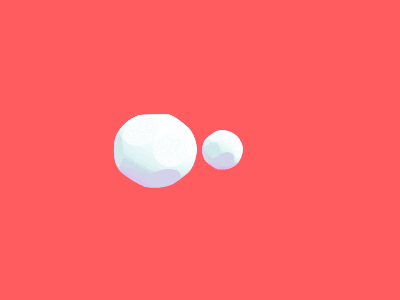
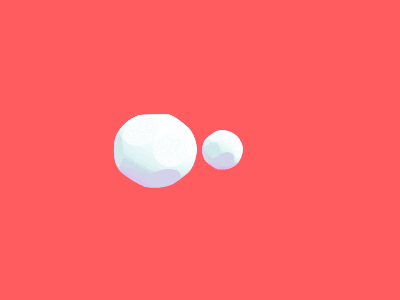
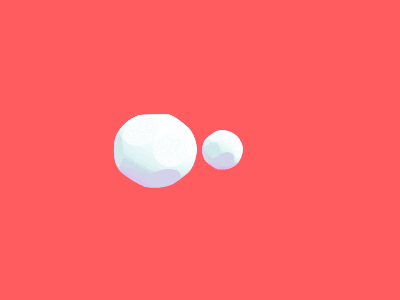
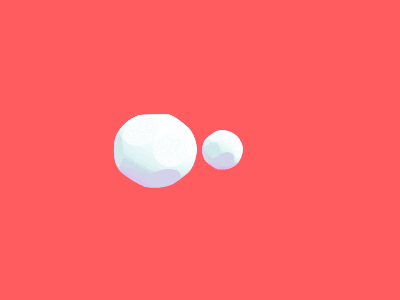
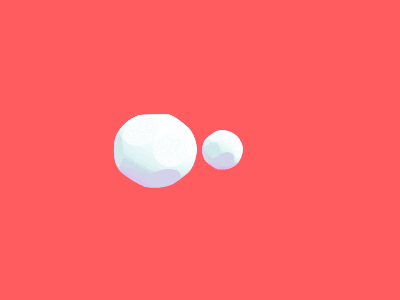