2022-07-25 更新
On Higher Adversarial Susceptibility of Contrastive Self-Supervised Learning
Authors:Rohit Gupta, Naveed Akhtar, Ajmal Mian, Mubarak Shah
Contrastive self-supervised learning (CSL) has managed to match or surpass the performance of supervised learning in image and video classification. However, it is still largely unknown if the nature of the representation induced by the two learning paradigms is similar. We investigate this under the lens of adversarial robustness. Our analytical treatment of the problem reveals intrinsic higher sensitivity of CSL over supervised learning. It identifies the uniform distribution of data representation over a unit hypersphere in the CSL representation space as the key contributor to this phenomenon. We establish that this increases model sensitivity to input perturbations in the presence of false negatives in the training data. Our finding is supported by extensive experiments for image and video classification using adversarial perturbations and other input corruptions. Building on the insights, we devise strategies that are simple, yet effective in improving model robustness with CSL training. We demonstrate up to 68% reduction in the performance gap between adversarially attacked CSL and its supervised counterpart. Finally, we contribute to robust CSL paradigm by incorporating our findings in adversarial self-supervised learning. We demonstrate an average gain of about 5% over two different state-of-the-art methods in this domain.
PDF
点此查看论文截图
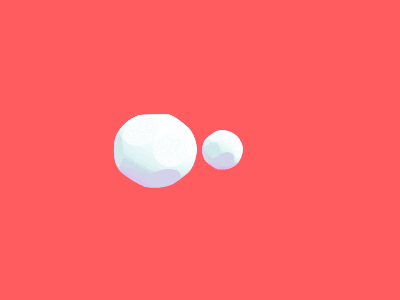
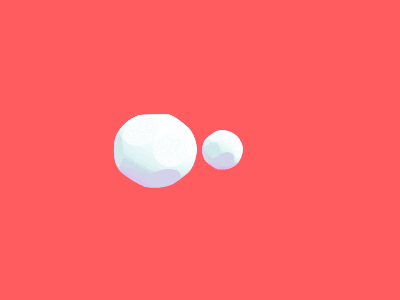
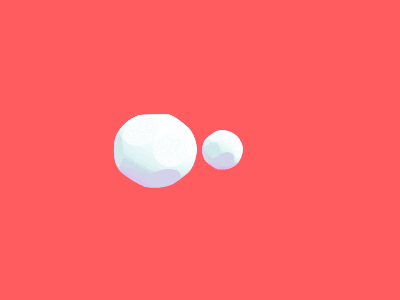
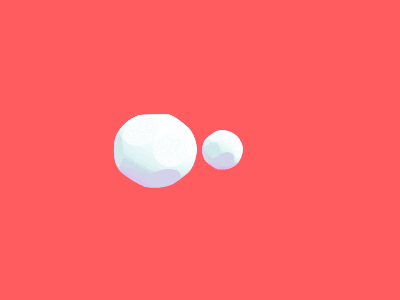