2022-07-25 更新
Bi-level Feature Alignment for Versatile Image Translation and Manipulation
Authors:Fangneng Zhan, Yingchen Yu, Rongliang Wu, Jiahui Zhang, Kaiwen Cui, Aoran Xiao, Shijian Lu, Chunyan Miao
Generative adversarial networks (GANs) have achieved great success in image translation and manipulation. However, high-fidelity image generation with faithful style control remains a grand challenge in computer vision. This paper presents a versatile image translation and manipulation framework that achieves accurate semantic and style guidance in image generation by explicitly building a correspondence. To handle the quadratic complexity incurred by building the dense correspondences, we introduce a bi-level feature alignment strategy that adopts a top-$k$ operation to rank block-wise features followed by dense attention between block features which reduces memory cost substantially. As the top-$k$ operation involves index swapping which precludes the gradient propagation, we approximate the non-differentiable top-$k$ operation with a regularized earth mover’s problem so that its gradient can be effectively back-propagated. In addition, we design a novel semantic position encoding mechanism that builds up coordinate for each individual semantic region to preserve texture structures while building correspondences. Further, we design a novel confidence feature injection module which mitigates mismatch problem by fusing features adaptively according to the reliability of built correspondences. Extensive experiments show that our method achieves superior performance qualitatively and quantitatively as compared with the state-of-the-art.
PDF Accepted to ECCV 2022
点此查看论文截图
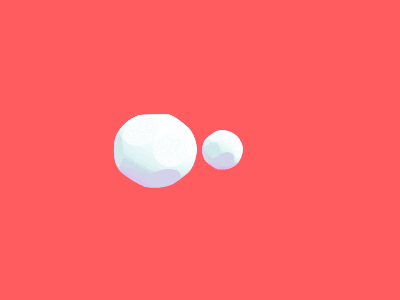
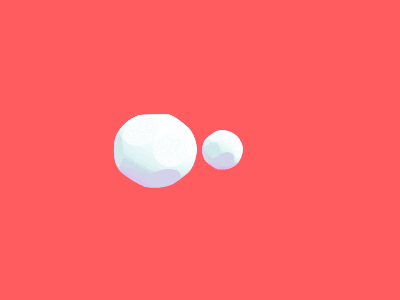
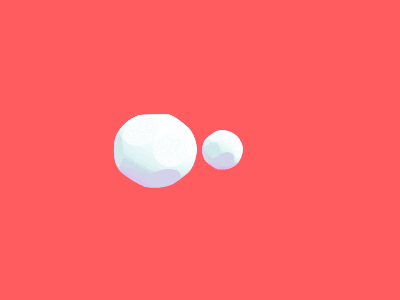
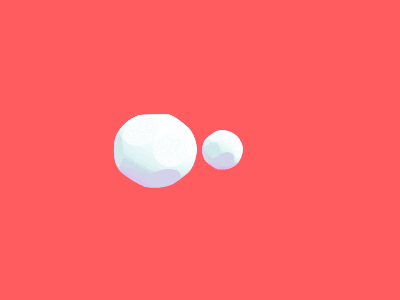
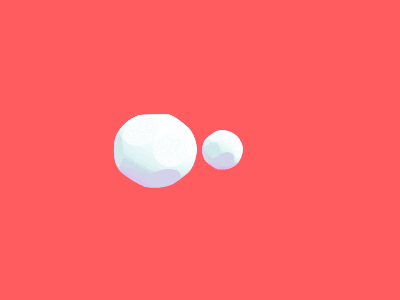
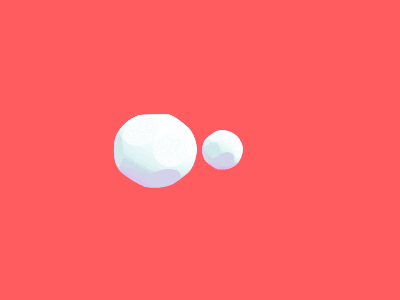