2022-07-09 更新
Learning Multi-View Aggregation In the Wild for Large-Scale 3D Semantic Segmentation
Authors:Damien Robert, Bruno Vallet, Loic Landrieu
Recent works on 3D semantic segmentation propose to exploit the synergy between images and point clouds by processing each modality with a dedicated network and projecting learned 2D features onto 3D points. Merging large-scale point clouds and images raises several challenges, such as constructing a mapping between points and pixels, and aggregating features between multiple views. Current methods require mesh reconstruction or specialized sensors to recover occlusions, and use heuristics to select and aggregate available images. In contrast, we propose an end-to-end trainable multi-view aggregation model leveraging the viewing conditions of 3D points to merge features from images taken at arbitrary positions. Our method can combine standard 2D and 3D networks and outperforms both 3D models operating on colorized point clouds and hybrid 2D/3D networks without requiring colorization, meshing, or true depth maps. We set a new state-of-the-art for large-scale indoor/outdoor semantic segmentation on S3DIS (74.7 mIoU 6-Fold) and on KITTI-360 (58.3 mIoU). Our full pipeline is accessible at https://github.com/drprojects/DeepViewAgg, and only requires raw 3D scans and a set of images and poses.
PDF Accepted to CVPR 2022 with an Oral presentation and Best Paper candidate; camera ready version. 17 pages, 11 figures. Code and data available at https://github.com/drprojects/DeepViewAgg
点此查看论文截图
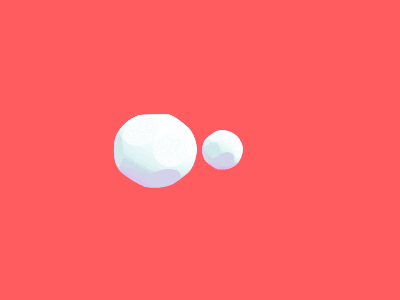
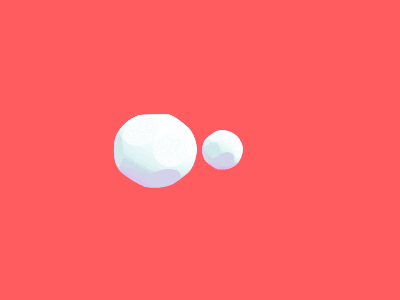
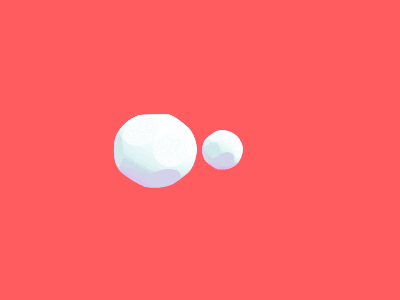
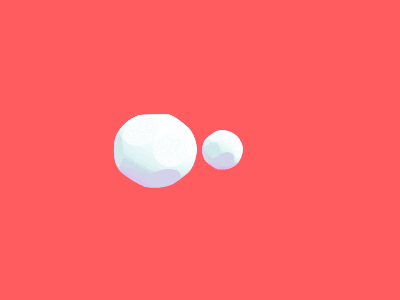
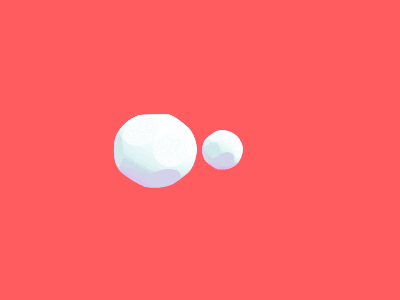
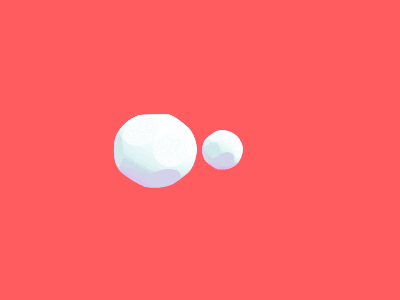
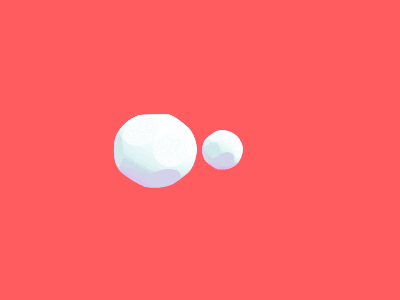
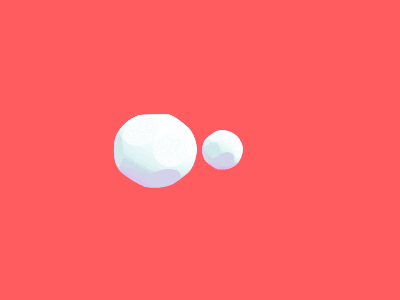
Bridging the Gap between Object and Image-level Representations for Open-Vocabulary Detection
Authors:Hanoona Rasheed, Muhammad Maaz, Muhammad Uzair Khattak, Salman Khan, Fahad Shahbaz Khan
Existing open-vocabulary object detectors typically enlarge their vocabulary sizes by leveraging different forms of weak supervision. This helps generalize to novel objects at inference. Two popular forms of weak-supervision used in open-vocabulary detection (OVD) include pretrained CLIP model and image-level supervision. We note that both these modes of supervision are not optimally aligned for the detection task: CLIP is trained with image-text pairs and lacks precise localization of objects while the image-level supervision has been used with heuristics that do not accurately specify local object regions. In this work, we propose to address this problem by performing object-centric alignment of the language embeddings from the CLIP model. Furthermore, we visually ground the objects with only image-level supervision using a pseudo-labeling process that provides high-quality object proposals and helps expand the vocabulary during training. We establish a bridge between the above two object-alignment strategies via a novel weight transfer function that aggregates their complimentary strengths. In essence, the proposed model seeks to minimize the gap between object and image-centric representations in the OVD setting. On the COCO benchmark, our proposed approach achieves 40.3 AP50 on novel classes, an absolute 11.9 gain over the previous best performance.For LVIS, we surpass the state-of-the-art ViLD model by 5.0 mask AP for rare categories and 3.4 overall. Code: https://bit.ly/3byZoQp.
PDF
点此查看论文截图
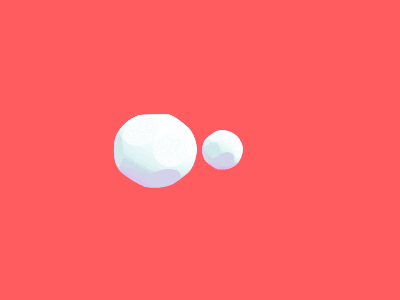
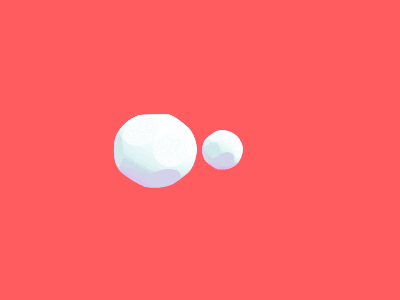
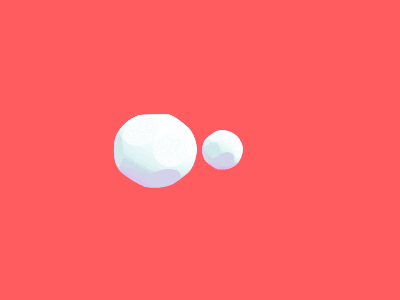
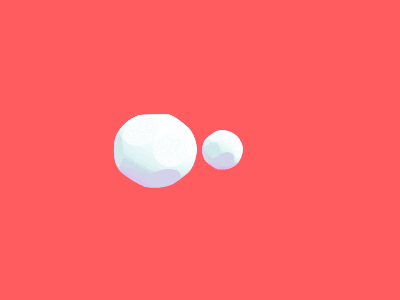
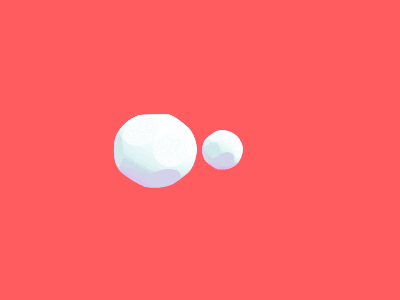
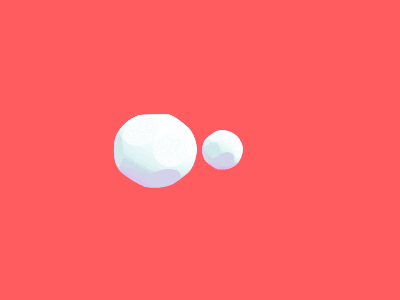
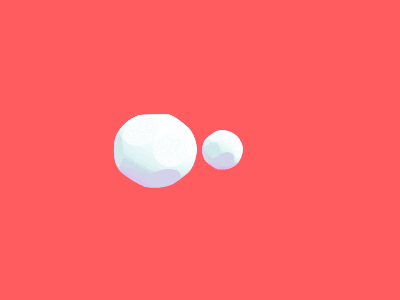
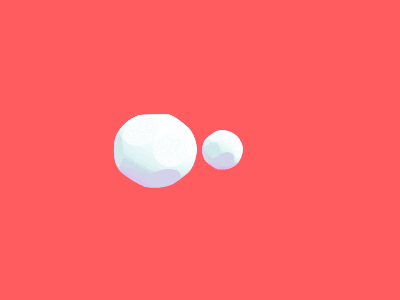
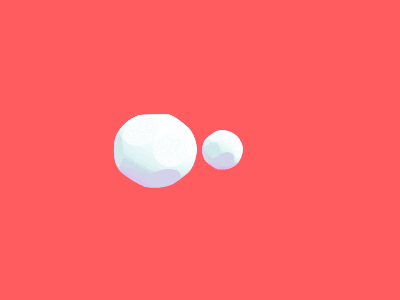
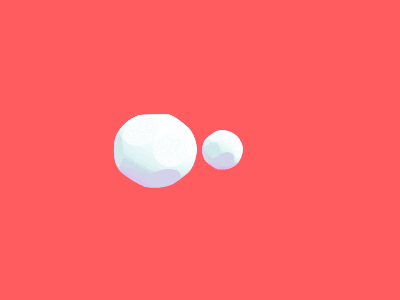
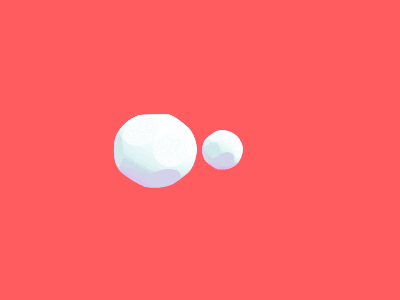