2022-07-08 更新
LaTeRF: Label and Text Driven Object Radiance Fields
Authors:Ashkan Mirzaei, Yash Kant, Jonathan Kelly, Igor Gilitschenski
Obtaining 3D object representations is important for creating photo-realistic simulators and collecting assets for AR/VR applications. Neural fields have shown their effectiveness in learning a continuous volumetric representation of a scene from 2D images, but acquiring object representations from these models with weak supervision remains an open challenge. In this paper we introduce LaTeRF, a method for extracting an object of interest from a scene given 2D images of the entire scene and known camera poses, a natural language description of the object, and a small number of point-labels of object and non-object points in the input images. To faithfully extract the object from the scene, LaTeRF extends the NeRF formulation with an additional `objectness’ probability at each 3D point. Additionally, we leverage the rich latent space of a pre-trained CLIP model combined with our differentiable object renderer, to inpaint the occluded parts of the object. We demonstrate high-fidelity object extraction on both synthetic and real datasets and justify our design choices through an extensive ablation study.
PDF
点此查看论文截图
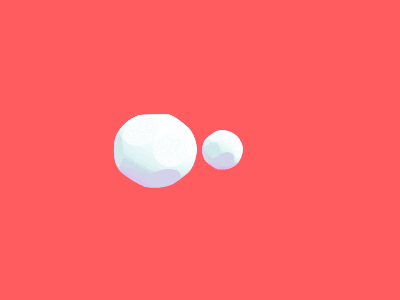
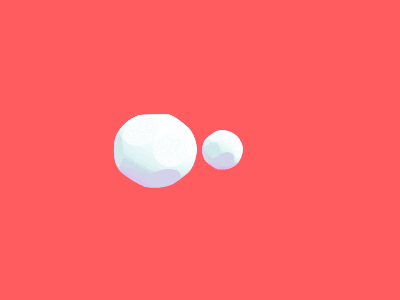