2022-07-08 更新
Selectively increasing the diversity of GAN-generated samples
Authors:Jan Dubiński, Kamil Deja, Sandro Wenzel, Przemysław Rokita, Tomasz Trzciński
Generative Adversarial Networks (GANs) are powerful models able to synthesize data samples closely resembling the distribution of real data, yet the diversity of those generated samples is limited due to the so-called mode collapse phenomenon observed in GANs. Especially prone to mode collapse are conditional GANs, which tend to ignore the input noise vector and focus on the conditional information. Recent methods proposed to mitigate this limitation increase the diversity of generated samples, yet they reduce the performance of the models when similarity of samples is required. To address this shortcoming, we propose a novel method to selectively increase the diversity of GAN-generated samples. By adding a simple, yet effective regularization to the training loss function we encourage the generator to discover new data modes for inputs related to diverse outputs while generating consistent samples for the remaining ones. More precisely, we maximise the ratio of distances between generated images and input latent vectors scaling the effect according to the diversity of samples for a given conditional input. We show the superiority of our method in a synthetic benchmark as well as a real-life scenario of simulating data from the Zero Degree Calorimeter of ALICE experiment in LHC, CERN.
PDF
点此查看论文截图
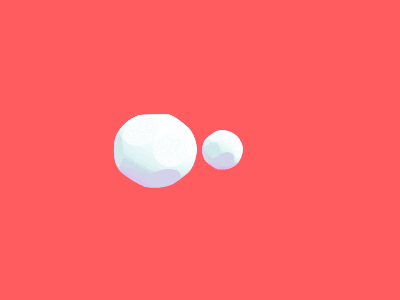
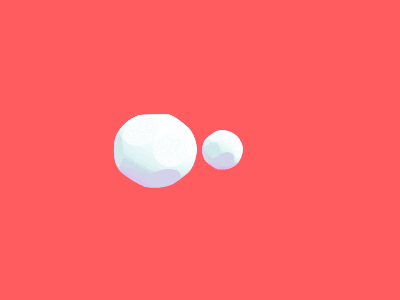
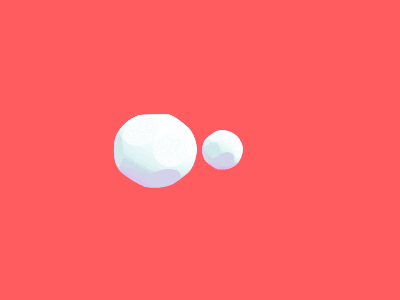
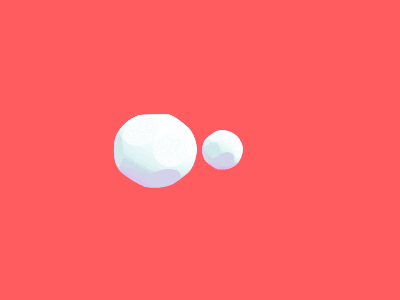
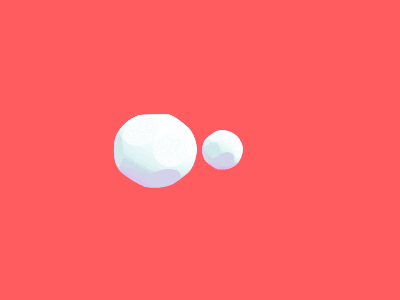
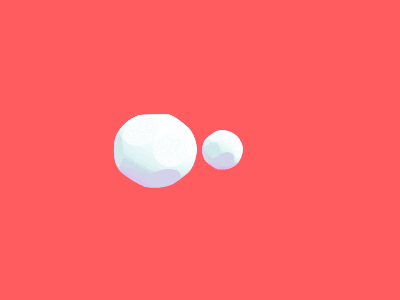
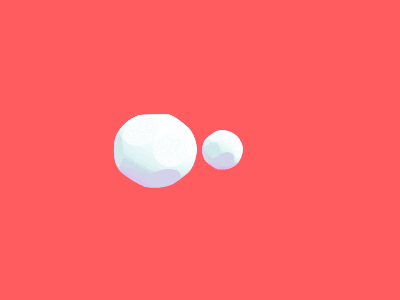