2022-07-06 更新
AGIC: Approximate Gradient Inversion Attack on Federated Learning
Authors:Jin Xu, Chi Hong, Jiyue Huang, Lydia Y. Chen, Jérémie Decouchant
Federated learning is a private-by-design distributed learning paradigm where clients train local models on their own data before a central server aggregates their local updates to compute a global model. Depending on the aggregation method used, the local updates are either the gradients or the weights of local learning models. Recent reconstruction attacks apply a gradient inversion optimization on the gradient update of a single minibatch to reconstruct the private data used by clients during training. As the state-of-the-art reconstruction attacks solely focus on single update, realistic adversarial scenarios are overlooked, such as observation across multiple updates and updates trained from multiple mini-batches. A few studies consider a more challenging adversarial scenario where only model updates based on multiple mini-batches are observable, and resort to computationally expensive simulation to untangle the underlying samples for each local step. In this paper, we propose AGIC, a novel Approximate Gradient Inversion Attack that efficiently and effectively reconstructs images from both model or gradient updates, and across multiple epochs. In a nutshell, AGIC (i) approximates gradient updates of used training samples from model updates to avoid costly simulation procedures, (ii) leverages gradient/model updates collected from multiple epochs, and (iii) assigns increasing weights to layers with respect to the neural network structure for reconstruction quality. We extensively evaluate AGIC on three datasets, CIFAR-10, CIFAR-100 and ImageNet. Our results show that AGIC increases the peak signal-to-noise ratio (PSNR) by up to 50% compared to two representative state-of-the-art gradient inversion attacks. Furthermore, AGIC is faster than the state-of-the-art simulation based attack, e.g., it is 5x faster when attacking FedAvg with 8 local steps in between model updates.
PDF This paper is accepted at the 41st International Symposium on Reliable Distributed Systems (SRDS 2022)
点此查看论文截图
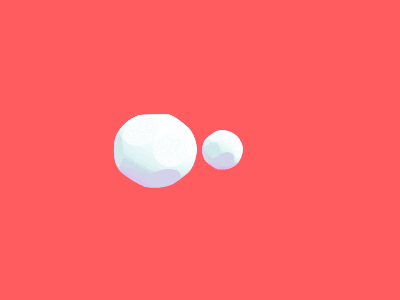
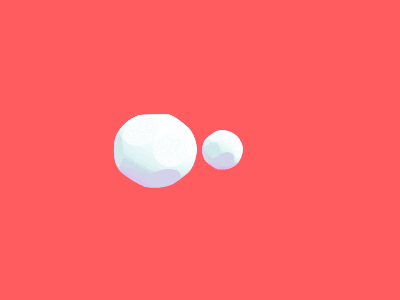
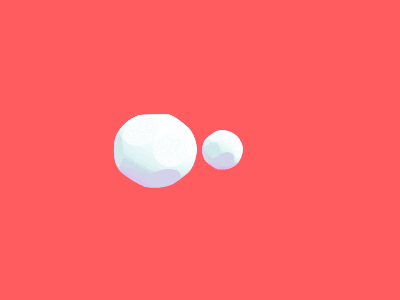
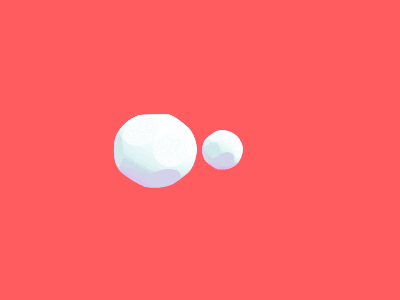
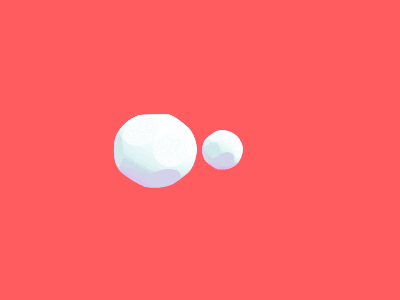
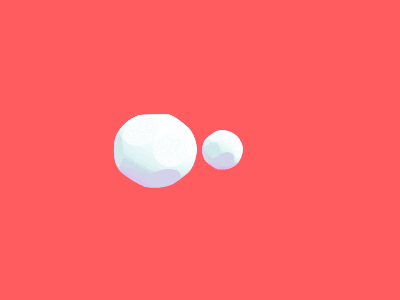
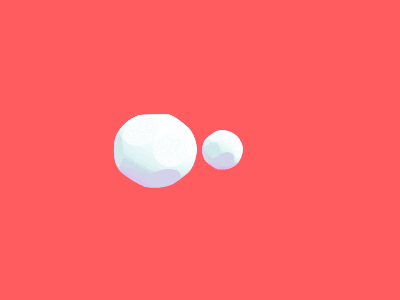
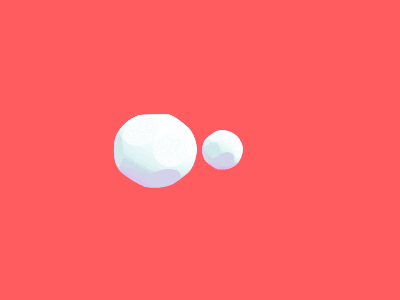
Task-agnostic Defense against Adversarial Patch Attacks
Authors:Ke Xu, Yao Xiao, Zhaoheng Zheng, Kaijie Cai, Ram Nevatia
Adversarial patch attacks mislead neural networks by injecting adversarial pixels within a designated local region. Patch attacks can be highly effective in a variety of tasks and physically realizable via attachment (e.g. a sticker) to the real-world objects. Despite the diversity in attack patterns, adversarial patches tend to be highly textured and different in appearance from natural images. We exploit this property and present PatchZero, a task-agnostic defense against white-box adversarial patches. Specifically, our defense detects the adversarial pixels and “zeros out” the patch region by repainting with mean pixel values. We formulate the patch detection problem as a semantic segmentation task such that our model can generalize to patches of any size and shape. We further design a two-stage adversarial training scheme to defend against the stronger adaptive attacks. We thoroughly evaluate PatchZero on the image classification (ImageNet, RESISC45), object detection (PASCAL VOC), and video classification (UCF101) datasets. Our method achieves SOTA robust accuracy without any degradation in the benign performance.
PDF
点此查看论文截图
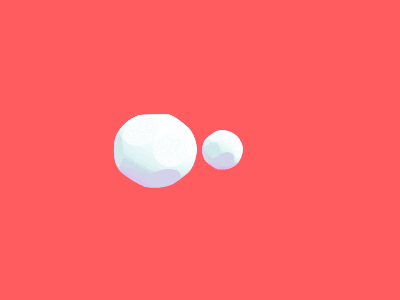
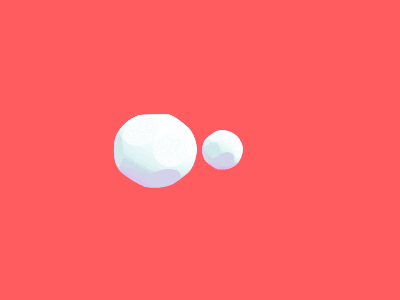
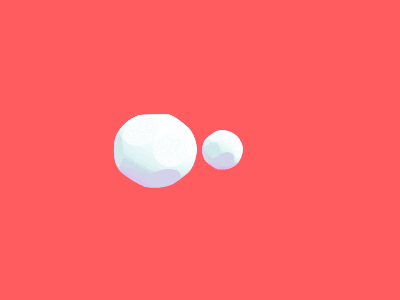
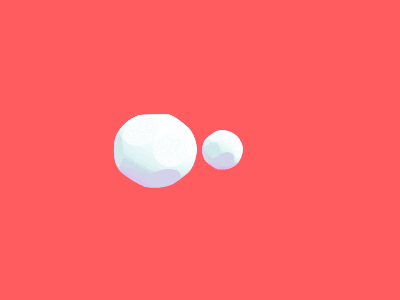