2022-06-14 更新
Consistent Attack: Universal Adversarial Perturbation on Embodied Vision Navigation
Authors:You Qiaoben, Chengyang Ying, Xinning Zhou, Hang Su, Jun Zhu, Bo Zhang
Embodied agents in vision navigation coupled with deep neural networks have attracted increasing attention. However, deep neural networks are vulnerable to malicious adversarial noises, which may potentially cause catastrophic failures in Embodied Vision Navigation. Among these adversarial noises, universal adversarial perturbations (UAP), i.e., the image-agnostic perturbation applied on each frame received by the agent, are more critical for Embodied Vision Navigation since they are computation-efficient and application-practical during the attack. However, existing UAP methods do not consider the system dynamics of Embodied Vision Navigation. For extending UAP in the sequential decision setting, we formulate the disturbed environment under the universal noise $\delta$, as a $\delta$-disturbed Markov Decision Process ($\delta$-MDP). Based on the formulation, we analyze the properties of $\delta$-MDP and propose two novel Consistent Attack methods for attacking Embodied agents, which first consider the dynamic of the MDP by estimating the disturbed Q function and the disturbed distribution. In spite of victim models, our Consistent Attack can cause a significant drop in the performance for the Goalpoint task in habitat. Extensive experimental results indicate that there exist potential risks for applying Embodied Vision Navigation methods to the real world.
PDF
论文截图
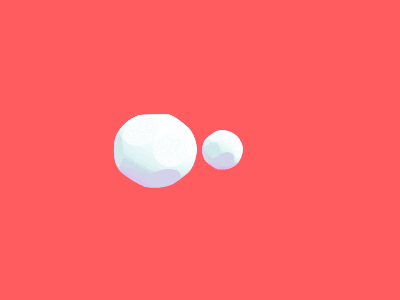
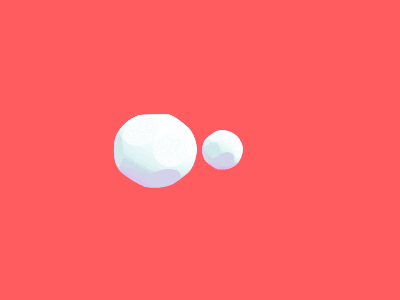
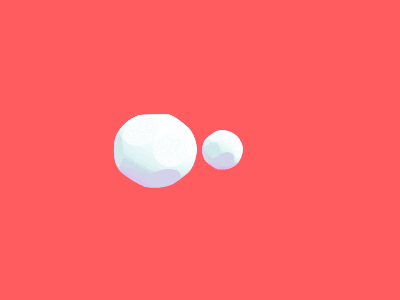
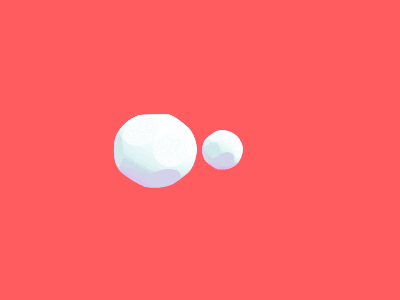