2022-06-13 更新
Polymorphic-GAN: Generating Aligned Samples across Multiple Domains with Learned Morph Maps
Authors:Seung Wook Kim, Karsten Kreis, Daiqing Li, Antonio Torralba, Sanja Fidler
Modern image generative models show remarkable sample quality when trained on a single domain or class of objects. In this work, we introduce a generative adversarial network that can simultaneously generate aligned image samples from multiple related domains. We leverage the fact that a variety of object classes share common attributes, with certain geometric differences. We propose Polymorphic-GAN which learns shared features across all domains and a per-domain morph layer to morph shared features according to each domain. In contrast to previous works, our framework allows simultaneous modelling of images with highly varying geometries, such as images of human faces, painted and artistic faces, as well as multiple different animal faces. We demonstrate that our model produces aligned samples for all domains and show how it can be used for applications such as segmentation transfer and cross-domain image editing, as well as training in low-data regimes. Additionally, we apply our Polymorphic-GAN on image-to-image translation tasks and show that we can greatly surpass previous approaches in cases where the geometric differences between domains are large.
PDF CVPR 2022 Oral
论文截图
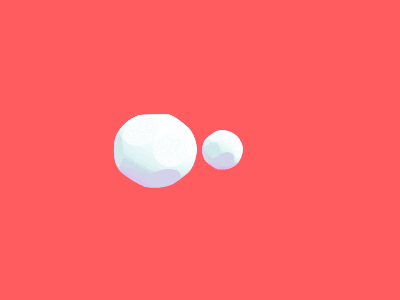
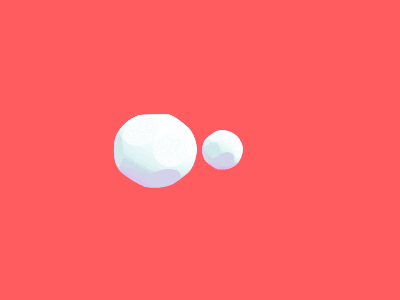
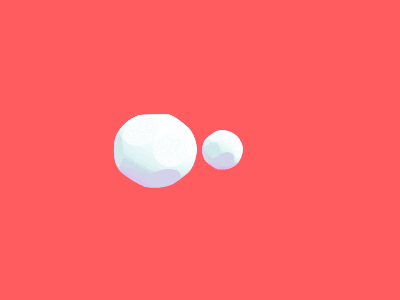
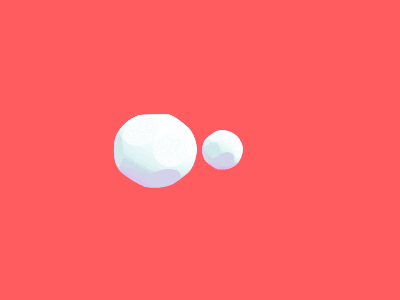
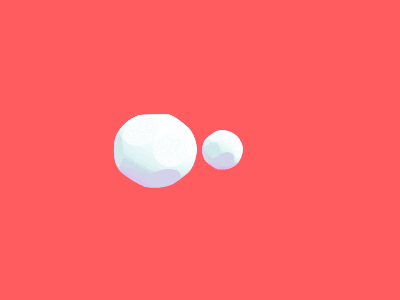
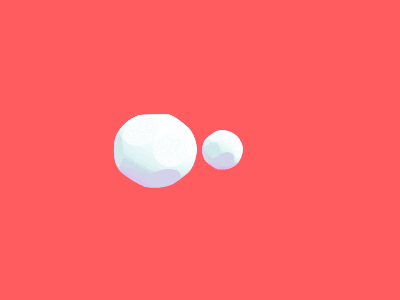
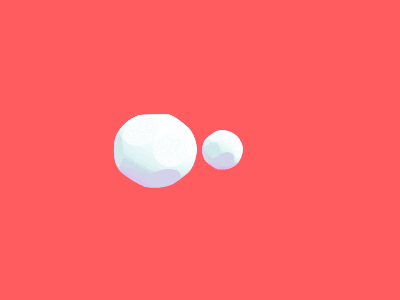
Reconstruct Face from Features Using GAN Generator as a Distribution Constraint
Authors:Xingbo Dong, Zhihui Miao, Lan Ma, Jiajun Shen, Zhe Jin, Zhenhua Guo, Andrew Beng Jin Teoh
Face recognition based on the deep convolutional neural networks (CNN) shows superior accuracy performance attributed to the high discriminative features extracted. Yet, the security and privacy of the extracted features from deep learning models (deep features) have been often overlooked. This paper proposes the reconstruction of face images from deep features without accessing the CNN network configurations as a constrained optimization problem. Such optimization minimizes the distance between the features extracted from the original face image and the reconstructed face image. Instead of directly solving the optimization problem in the image space, we innovatively reformulate the problem by looking for a latent vector of a GAN generator, then use it to generate the face image. The GAN generator serves as a dual role in this novel framework, i.e., face distribution constraint of the optimization goal and a face generator. On top of the novel optimization task, we also propose an attack pipeline to impersonate the target user based on the generated face image. Our results show that the generated face images can achieve a state-of-the-art successful attack rate of 98.0\% on LFW under type-I attack @ FAR of 0.1\%. Our work sheds light on the biometric deployment to meet the privacy-preserving and security policies.
PDF
论文截图
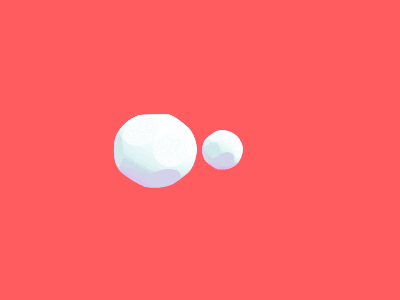
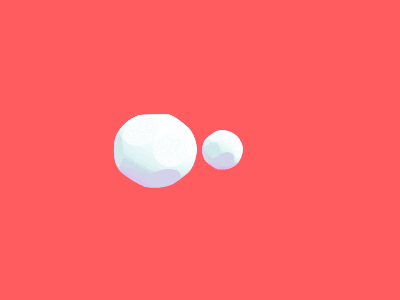
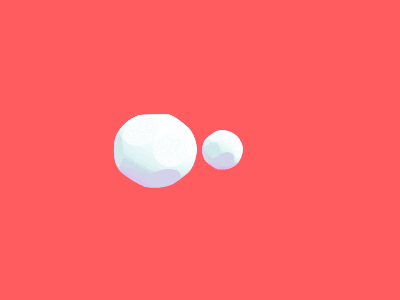
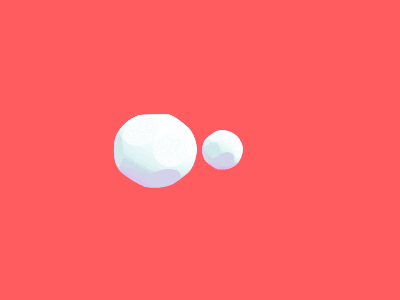
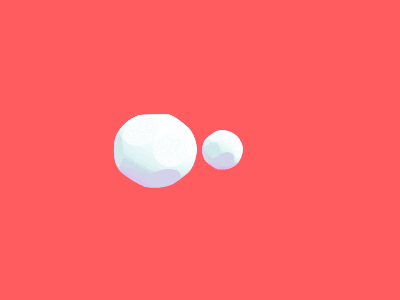
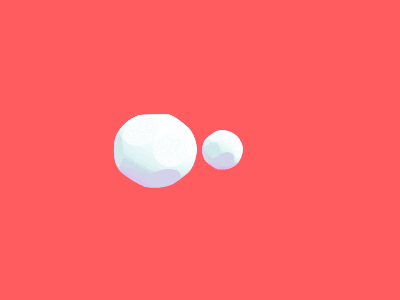
Domain Transformer: Predicting Samples of Unseen, Future Domains
Authors:Johannes Schneider
The data distribution commonly evolves over time leading to problems such as concept drift that often decrease classifier performance. Current techniques are not adequate for this problem because they either require detailed knowledge of the transformation or are not suited for anticipating unseen domains but can only adapt to domains, where data samples are available. We seek to predict unseen data (and their labels) allowing us to tackle challenges s a non-constant data distribution in a proactive manner rather than detecting and reacting to already existing changes that might already have led to errors. To this end, we learn a domain transformer in an unsupervised manner that allows generating data of unseen domains. Our approach first matches independently learned latent representations of two given domains obtained from an auto-encoder using a Cycle-GAN. In turn, a transformation of the original samples can be learned that can be applied iteratively to extrapolate to unseen domains. Our evaluation of CNNs on image data confirms the usefulness of the approach. It also achieves very good results on the well-known problem of unsupervised domain adaption, where only labels but no samples have to be predicted. Code is available at https://github.com/JohnTailor/DoTra.
PDF
论文截图
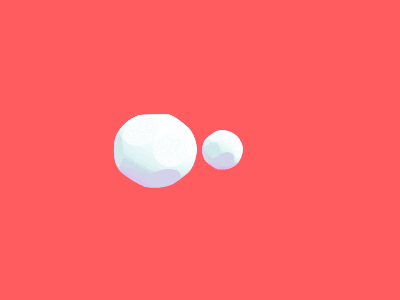
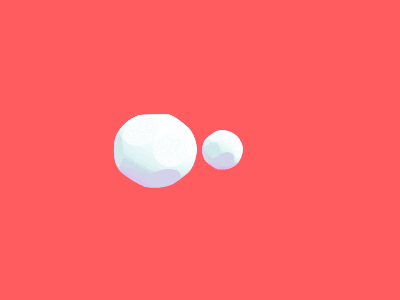
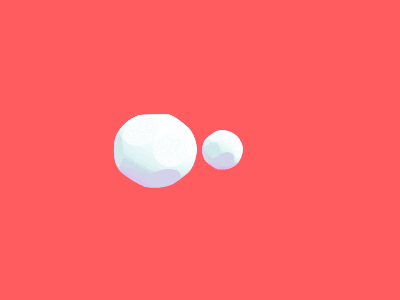
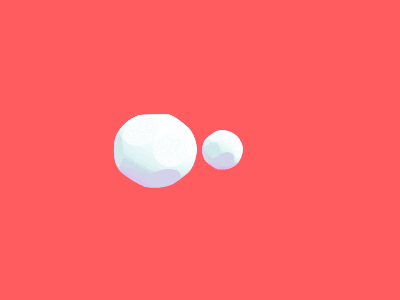
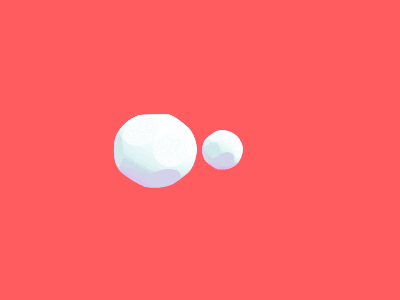
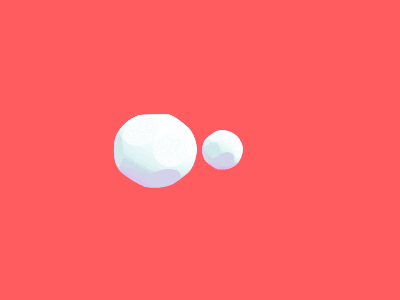
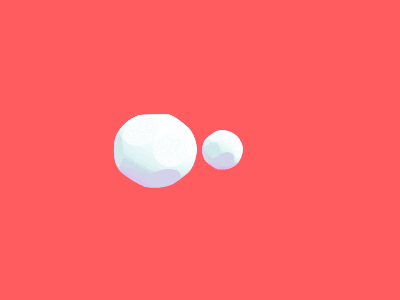
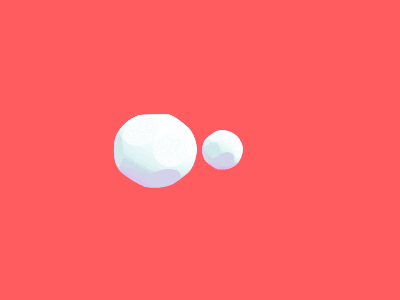