2022-05-31 更新
Point RCNN: An Angle-Free Framework for Rotated Object Detection
Authors:Qiang Zhou, Chaohui Yu, Zhibin Wang, Hao Li
Rotated object detection in aerial images is still challenging due to arbitrary orientations, large scale and aspect ratio variations, and extreme density of objects. Existing state-of-the-art rotated object detection methods mainly rely on angle-based detectors. However, angle regression can easily suffer from the long-standing boundary problem. To tackle this problem, we propose a purely angle-free framework for rotated object detection, called Point RCNN, which mainly consists of PointRPN and PointReg. In particular, PointRPN generates accurate rotated RoIs (RRoIs) by converting the learned representative points with a coarse-to-fine manner, which is motivated by RepPoints. Based on the learned RRoIs, PointReg performs corner points refinement for more accurate detection. In addition, aerial images are often severely unbalanced in categories, and existing methods almost ignore this issue. In this paper, we also experimentally verify that re-sampling the images of the rare categories will stabilize training and further improve the detection performance. Experiments demonstrate that our Point RCNN achieves the new state-of-the-art detection performance on commonly used aerial datasets, including DOTA-v1.0, DOTA-v1.5, and HRSC2016.
PDF
论文截图
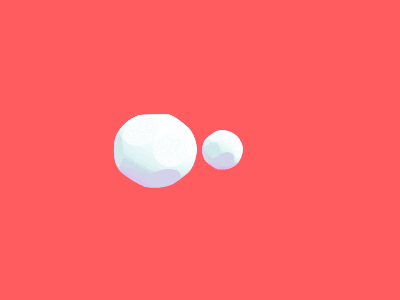
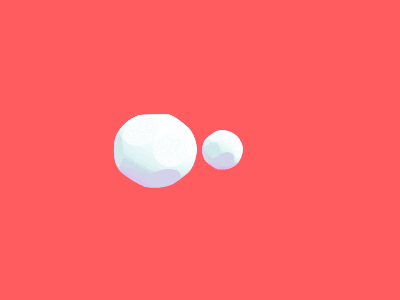
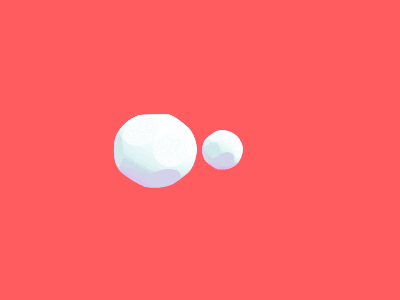
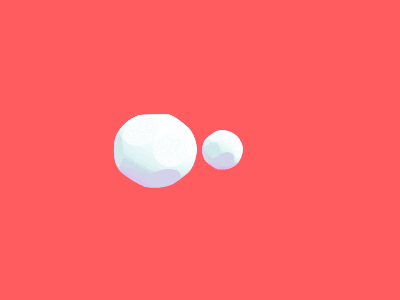
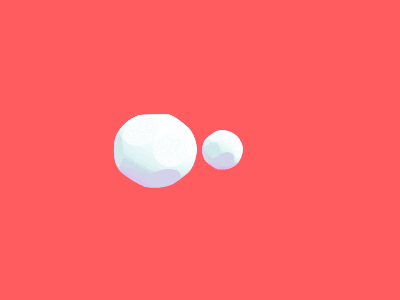
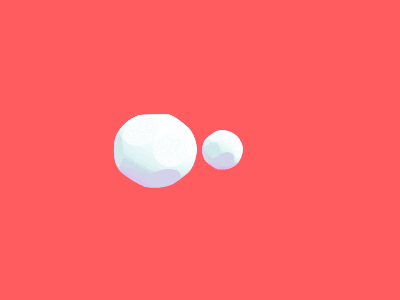
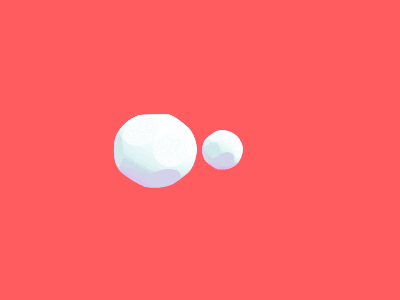
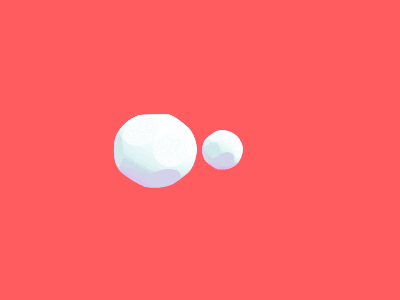
DAFNe: A One-Stage Anchor-Free Approach for Oriented Object Detection
Authors:Steven Lang, Fabrizio Ventola, Kristian Kersting
We present DAFNe, a Dense one-stage Anchor-Free deep Network for oriented object detection. As a one-stage model, it performs bounding box predictions on a dense grid over the input image, being architecturally simpler in design, as well as easier to optimize than its two-stage counterparts. Furthermore, as an anchor-free model, it reduces the prediction complexity by refraining from employing bounding box anchors. With DAFNe we introduce an orientation-aware generalization of the center-ness function for arbitrarily oriented bounding boxes to down-weight low-quality predictions and a center-to-corner bounding box prediction strategy that improves object localization performance. Our experiments show that DAFNe outperforms all previous one-stage anchor-free models on DOTA 1.0, DOTA 1.5, and UCAS-AOD and is on par with the best models on HRSC2016.
PDF Main paper: 8 pages, References: 2 pages, Appendix: 7 pages; Main paper: 6 figures, Appendix: 6 figures
论文截图
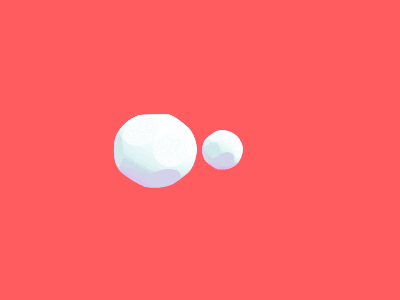
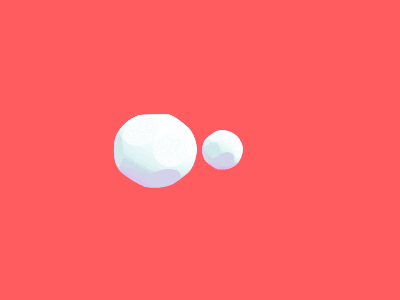
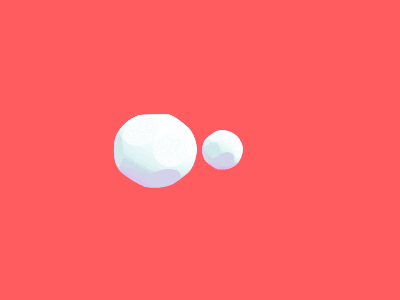
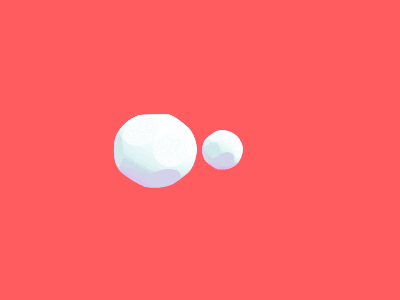
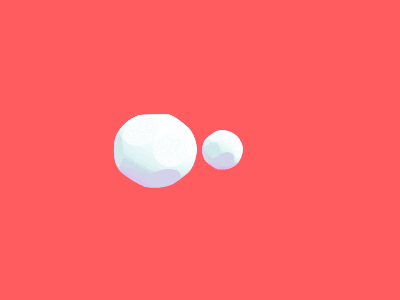
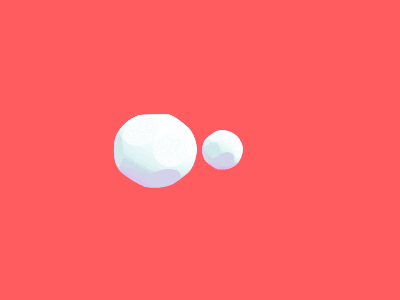
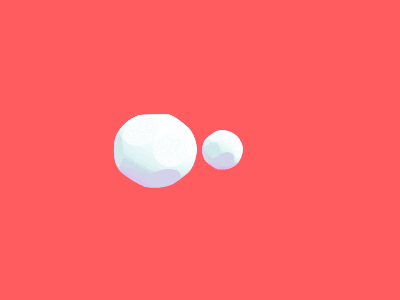
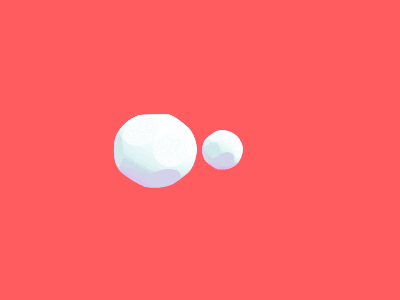
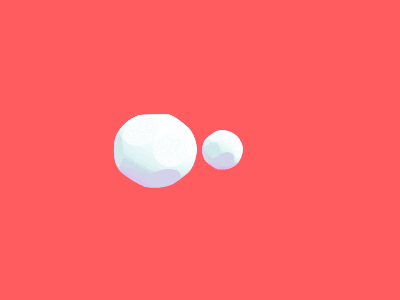
MonoDETR: Depth-guided Transformer for Monocular 3D Object Detection
Authors:Renrui Zhang, Han Qiu, Tai Wang, Ziyu Guo, Xuanzhuo Xu, Yu Qiao, Peng Gao, Hongsheng Li
Monocular 3D object detection has long been a challenging task in autonomous driving, which requires to decode 3D predictions solely from a single 2D image. Most existing methods follow conventional 2D object detectors to localize objects based on their centers, and predict 3D attributes by neighboring features around the centers. However, only using local features is insufficient to understand the scene-level 3D spatial structures and ignore the inter-object depth relations from contextual cues. In this paper, we introduce a novel framework for Monocular DEtection with a depth-guided TRansformer, named MonoDETR. The vanilla transformer is modified to be depth-aware and the whole detection process is then guided by depth. Specifically, we represent 3D object candidates as a set of queries and adopt an attention-based depth encoder to produce non-local depth embeddings of the input image. Then, we propose a depth-guided decoder with depth cross-attention modules to conduct both inter-query and query-scene depth feature interactions. In this way, each object query estimates its 3D attributes adaptively from the depth-guided regions from the image and is no longer constrained to use only neighboring visual features. MonoDETR is an end-to-end network without extra data or NMS post-processing and achieves state-of-the-art performance on KITTI benchmark with significant gains. Extensive ablation studies demonstrate the effectiveness of our approach and its potential to serve as a transformer baseline for future monocular 3D object detection research. Code is available at https://github.com/ZrrSkywalker/MonoDETR.
PDF
论文截图
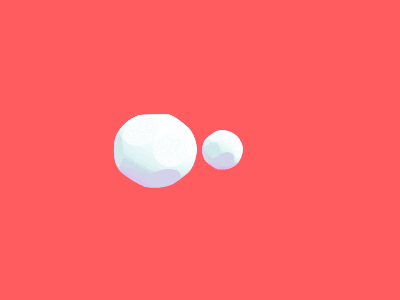
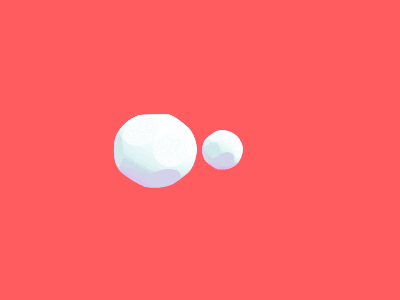
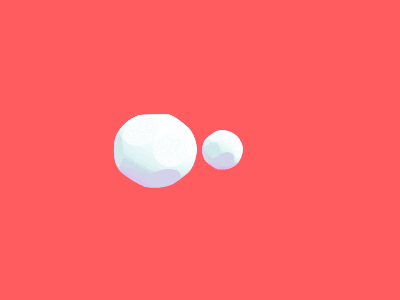
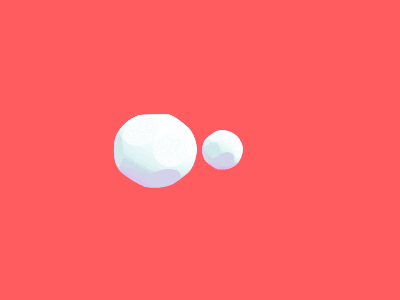
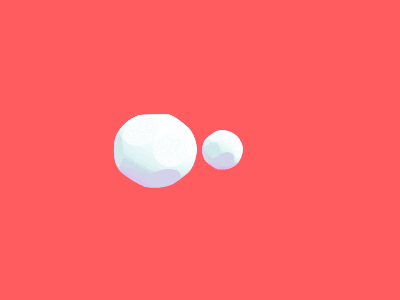
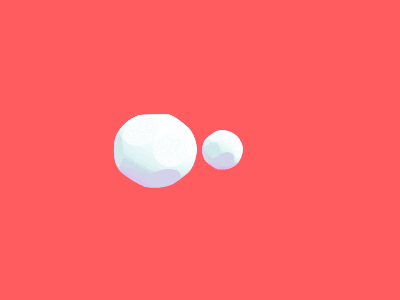
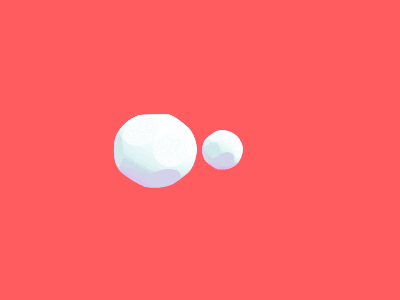
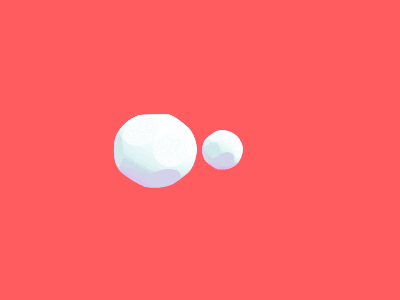
Towards Model Generalization for Monocular 3D Object Detection
Authors:Zhenyu Li, Zehui Chen, Ang Li, Liangji Fang, Qinhong Jiang, Xianming Liu, Junjun Jiang
Monocular 3D object detection (Mono3D) has achieved tremendous improvements with emerging large-scale autonomous driving datasets and the rapid development of deep learning techniques. However, caused by severe domain gaps (e.g., the field of view (FOV), pixel size, and object size among datasets), Mono3D detectors have difficulty in generalization, leading to drastic performance degradation on unseen domains. To solve these issues, we combine the position-invariant transform and multi-scale training with the pixel-size depth strategy to construct an effective unified camera-generalized paradigm (CGP). It fully considers discrepancies in the FOV and pixel size of images captured by different cameras. Moreover, we further investigate the obstacle in quantitative metrics when cross-dataset inference through an exhaustive systematic study. We discern that the size bias of prediction leads to a colossal failure. Hence, we propose the 2D-3D geometry-consistent object scaling strategy (GCOS) to bridge the gap via an instance-level augment. Our method called DGMono3D achieves remarkable performance on all evaluated datasets and surpasses the SoTA unsupervised domain adaptation scheme even without utilizing data on the target domain.
PDF Some mistakes are raised up and we need to re-write the paper and re-order the paper structure
论文截图
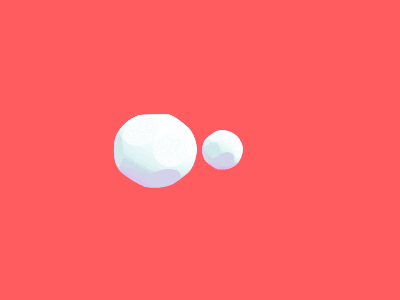
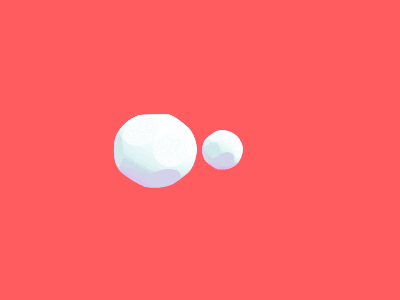
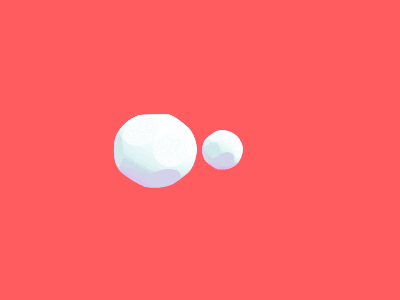
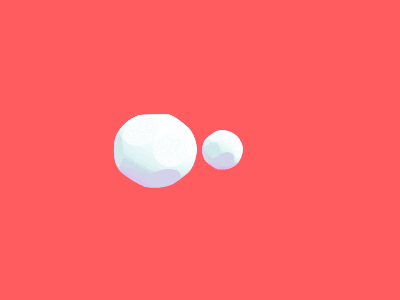
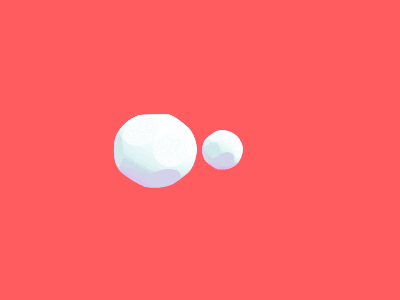
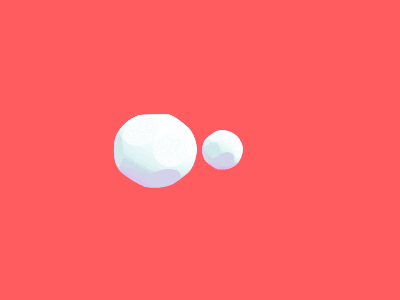
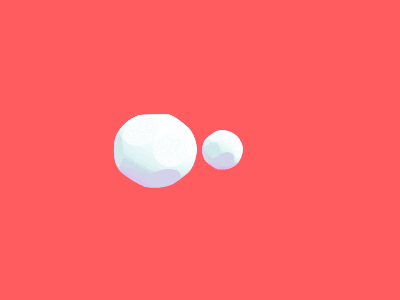
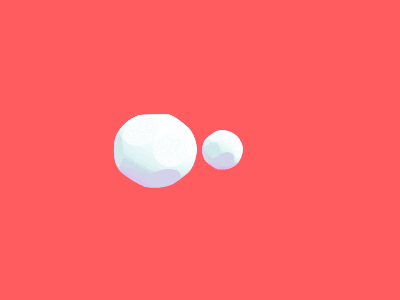
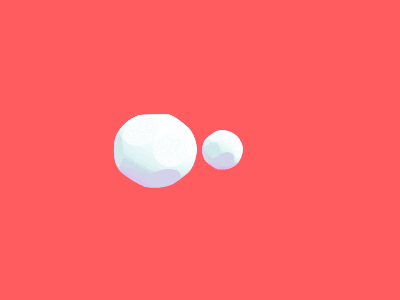