2022-05-28 更新
Active Domain Adaptation with Multi-level Contrastive Units for Semantic Segmentation
Authors:Hao Zhang, Ruimao Zhang, Zhanglin Peng, Junle Wang, Yanqing Jing
To further reduce the cost of semi-supervised domain adaptation (SSDA) labeling, a more effective way is to use active learning (AL) to annotate a selected subset with specific properties. However, domain adaptation tasks are always addressed in two interactive aspects: domain transfer and the enhancement of discrimination, which requires the selected data to be both uncertain under the model and diverse in feature space. Contrary to active learning in classification tasks, it is usually challenging to select pixels that contain both the above properties in segmentation tasks, leading to the complex design of pixel selection strategy. To address such an issue, we propose a novel Active Domain Adaptation scheme with Multi-level Contrastive Units (ADA-MCU) for semantic image segmentation. A simple pixel selection strategy followed with the construction of multi-level contrastive units is introduced to optimize the model for both domain adaptation and active supervised learning. In practice, MCUs are constructed from intra-image, cross-image, and cross-domain levels by using both labeled and unlabeled pixels. At each level, we define contrastive losses from center-to-center and pixel-to-pixel manners, with the aim of jointly aligning the category centers and reducing outliers near the decision boundaries. In addition, we also introduce a categories correlation matrix to implicitly describe the relationship between categories, which are used to adjust the weights of the losses for MCUs. Extensive experimental results on standard benchmarks show that the proposed method achieves competitive performance against state-of-the-art SSDA methods with 50% fewer labeled pixels and significantly outperforms state-of-the-art with a large margin by using the same level of annotation cost.
PDF
论文截图
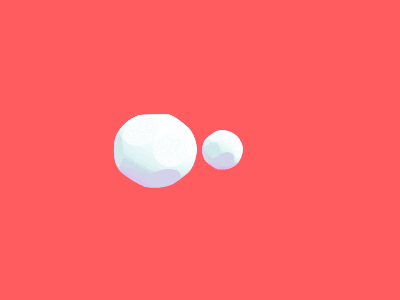
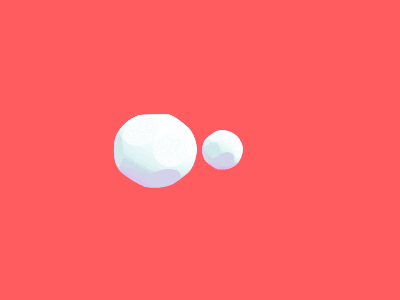
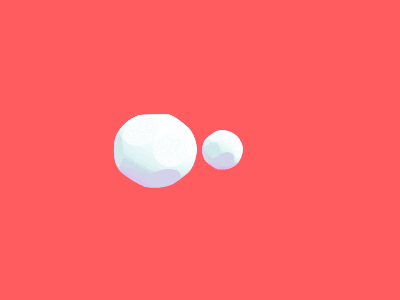
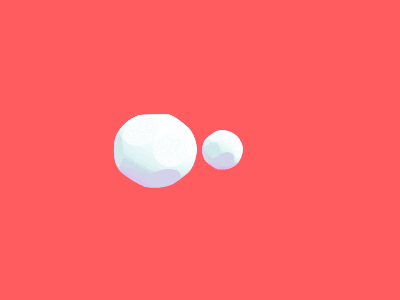
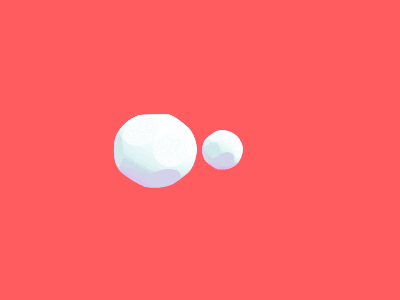
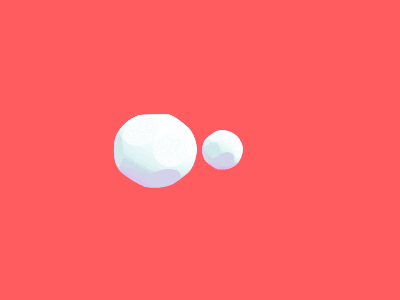
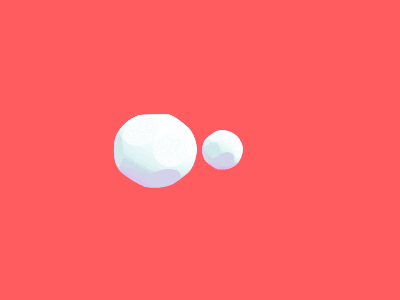
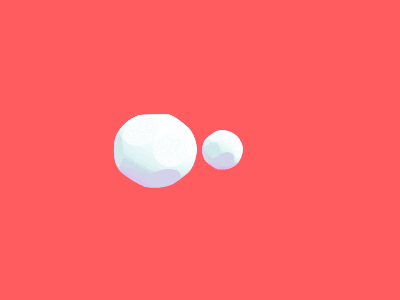
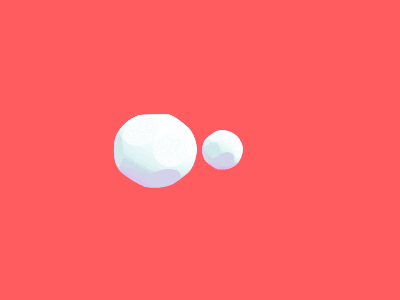
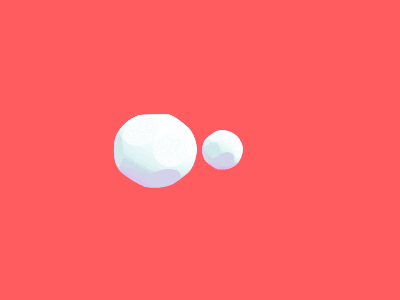
3D Object Detection for Autonomous Driving: A Survey
Authors:Rui Qian, Xin Lai, Xirong Li
Autonomous driving is regarded as one of the most promising remedies to shield human beings from severe crashes. To this end, 3D object detection serves as the core basis of perception stack especially for the sake of path planning, motion prediction, and collision avoidance etc. Taking a quick glance at the progress we have made, we attribute challenges to visual appearance recovery in the absence of depth information from images, representation learning from partially occluded unstructured point clouds, and semantic alignments over heterogeneous features from cross modalities. Despite existing efforts, 3D object detection for autonomous driving is still in its infancy. Recently, a large body of literature have been investigated to address this 3D vision task. Nevertheless, few investigations have looked into collecting and structuring this growing knowledge. We therefore aim to fill this gap in a comprehensive survey, encompassing all the main concerns including sensors, datasets, performance metrics and the recent state-of-the-art detection methods, together with their pros and cons. Furthermore, we provide quantitative comparisons with the state of the art. A case study on fifteen selected representative methods is presented, involved with runtime analysis, error analysis, and robustness analysis. Finally, we provide concluding remarks after an in-depth analysis of the surveyed works and identify promising directions for future work.
PDF The manuscript is accepted by Pattern Recognition on 14 May 2022
论文截图
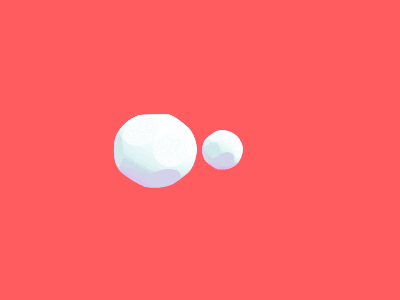
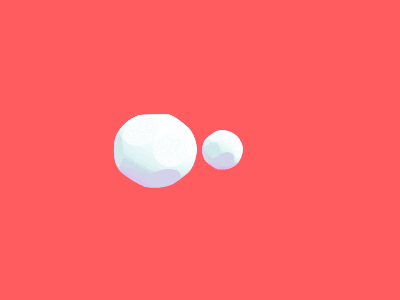
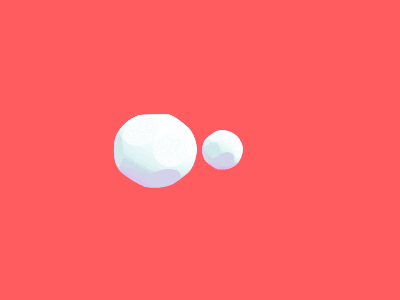
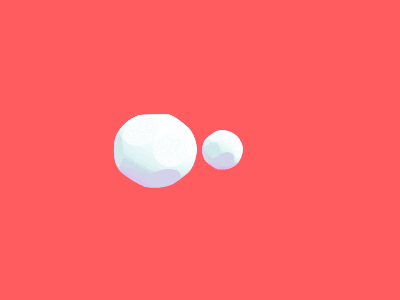
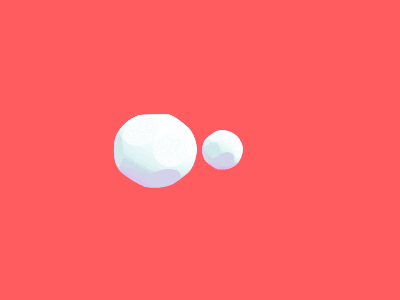
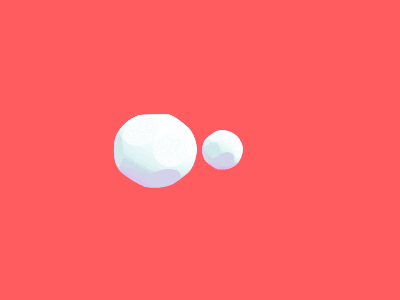
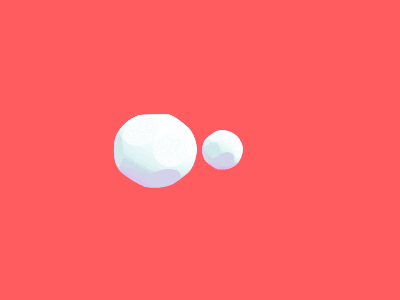
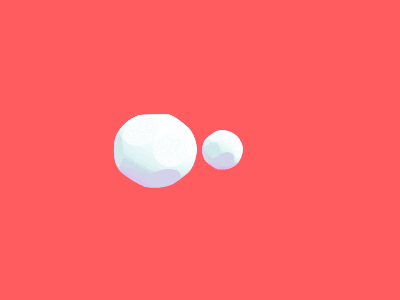
SemAffiNet: Semantic-Affine Transformation for Point Cloud Segmentation
Authors:Ziyi Wang, Yongming Rao, Xumin Yu, Jie Zhou, Jiwen Lu
Conventional point cloud semantic segmentation methods usually employ an encoder-decoder architecture, where mid-level features are locally aggregated to extract geometric information. However, the over-reliance on these class-agnostic local geometric representations may raise confusion between local parts from different categories that are similar in appearance or spatially adjacent. To address this issue, we argue that mid-level features can be further enhanced with semantic information, and propose semantic-affine transformation that transforms features of mid-level points belonging to different categories with class-specific affine parameters. Based on this technique, we propose SemAffiNet for point cloud semantic segmentation, which utilizes the attention mechanism in the Transformer module to implicitly and explicitly capture global structural knowledge within local parts for overall comprehension of each category. We conduct extensive experiments on the ScanNetV2 and NYUv2 datasets, and evaluate semantic-affine transformation on various 3D point cloud and 2D image segmentation baselines, where both qualitative and quantitative results demonstrate the superiority and generalization ability of our proposed approach. Code is available at https://github.com/wangzy22/SemAffiNet.
PDF Accepted to CVPR 2022
论文截图
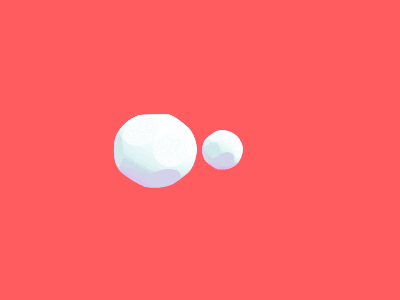
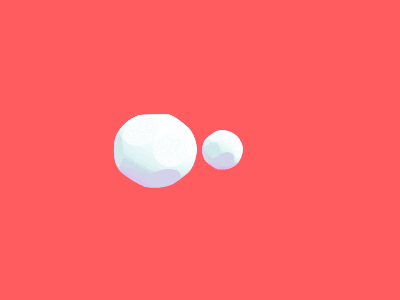
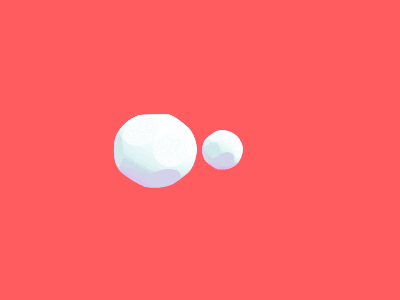
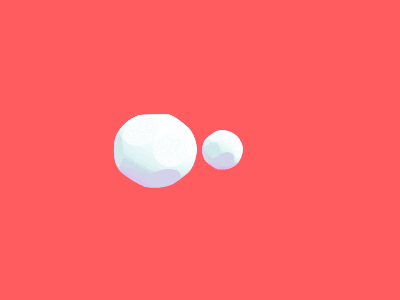
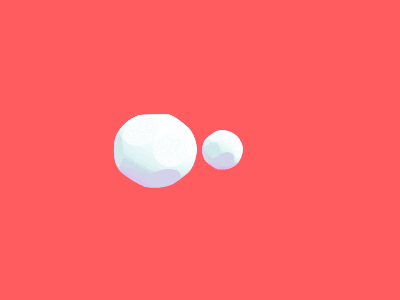
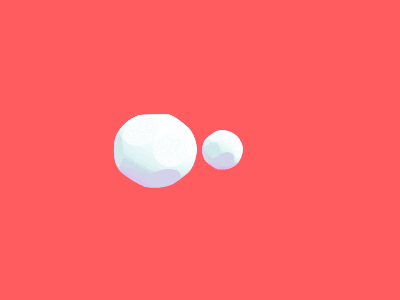
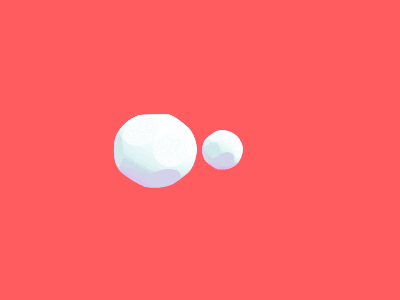
Towards Model Generalization for Monocular 3D Object Detection
Authors:Zhenyu Li, Zehui Chen, Ang Li, Liangji Fang, Qinhong Jiang, Xianming Liu, Junjun Jiang
Monocular 3D object detection (Mono3D) has achieved tremendous improvements with emerging large-scale autonomous driving datasets and the rapid development of deep learning techniques. However, caused by severe domain gaps (e.g., the field of view (FOV), pixel size, and object size among datasets), Mono3D detectors have difficulty in generalization, leading to drastic performance degradation on unseen domains. To solve these issues, we combine the position-invariant transform and multi-scale training with the pixel-size depth strategy to construct an effective unified camera-generalized paradigm (CGP). It fully considers discrepancies in the FOV and pixel size of images captured by different cameras. Moreover, we further investigate the obstacle in quantitative metrics when cross-dataset inference through an exhaustive systematic study. We discern that the size bias of prediction leads to a colossal failure. Hence, we propose the 2D-3D geometry-consistent object scaling strategy (GCOS) to bridge the gap via an instance-level augment. Our method called DGMono3D achieves remarkable performance on all evaluated datasets and surpasses the SoTA unsupervised domain adaptation scheme even without utilizing data on the target domain.
PDF
论文截图
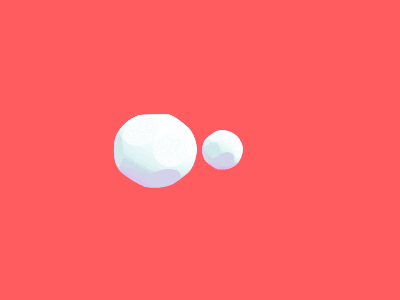
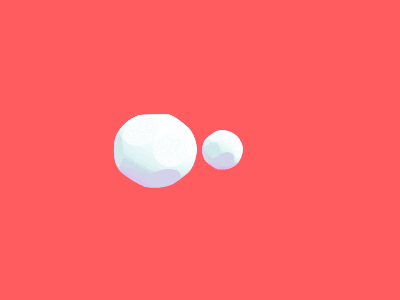
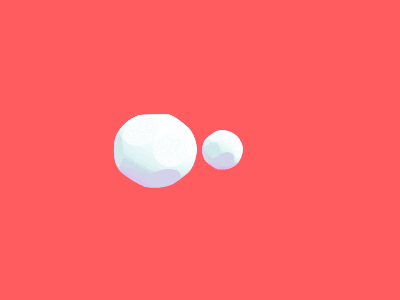
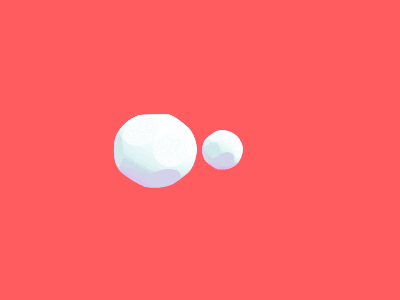
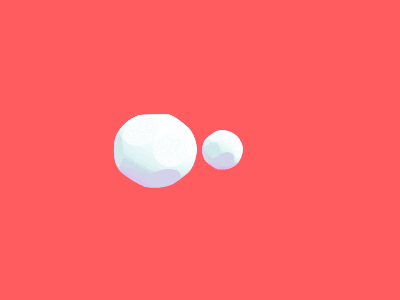
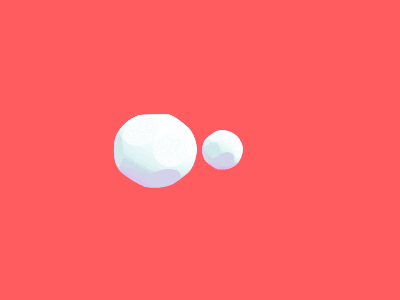
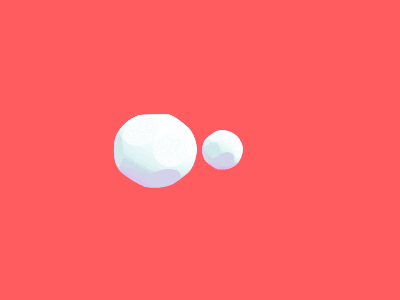
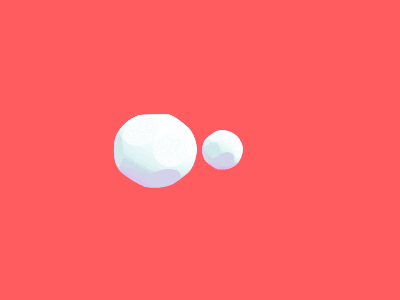
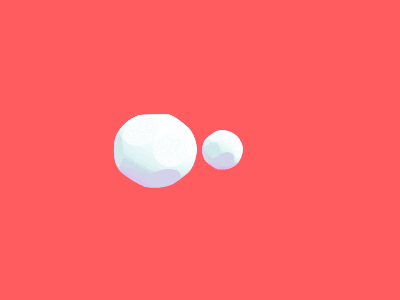
What to look at and where: Semantic and Spatial Refined Transformer for detecting human-object interactions
Authors:A S M Iftekhar, Hao Chen, Kaustav Kundu, Xinyu Li, Joseph Tighe, Davide Modolo
We propose a novel one-stage Transformer-based semantic and spatial refined transformer (SSRT) to solve the Human-Object Interaction detection task, which requires to localize humans and objects, and predicts their interactions. Differently from previous Transformer-based HOI approaches, which mostly focus at improving the design of the decoder outputs for the final detection, SSRT introduces two new modules to help select the most relevant object-action pairs within an image and refine the queries’ representation using rich semantic and spatial features. These enhancements lead to state-of-the-art results on the two most popular HOI benchmarks: V-COCO and HICO-DET.
PDF CVPR 2022 Oral
论文截图
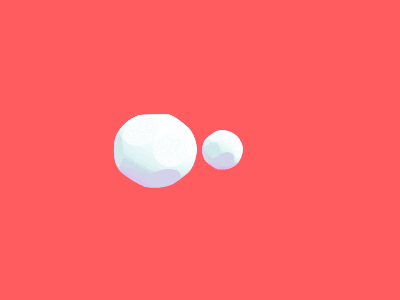
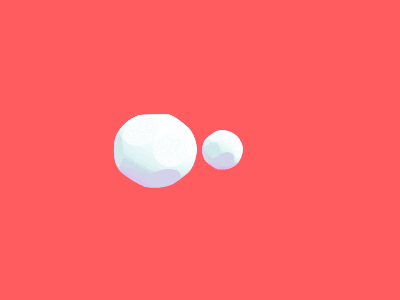
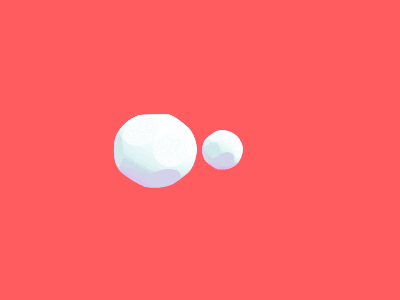
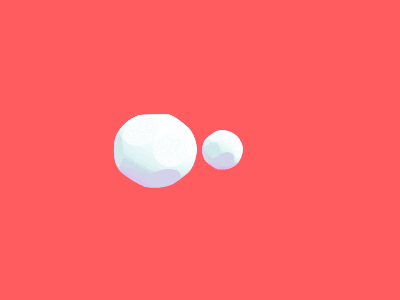
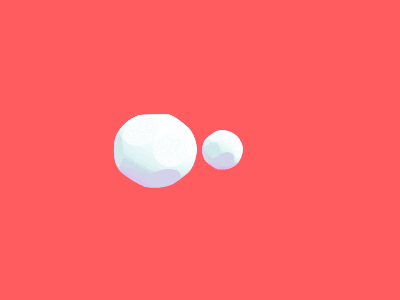
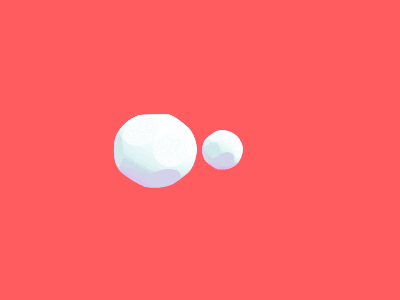