2022-05-28 更新
Improving Human Image Synthesis with Residual Fast Fourier Transformation and Wasserstein Distance
Authors:Jianhan Wu, Shijing Si, Jianzong Wang, Jing Xiao
With the rapid development of the Metaverse, virtual humans have emerged, and human image synthesis and editing techniques, such as pose transfer, have recently become popular. Most of the existing techniques rely on GANs, which can generate good human images even with large variants and occlusions. But from our best knowledge, the existing state-of-the-art method still has the following problems: the first is that the rendering effect of the synthetic image is not realistic, such as poor rendering of some regions. And the second is that the training of GAN is unstable and slow to converge, such as model collapse. Based on the above two problems, we propose several methods to solve them. To improve the rendering effect, we use the Residual Fast Fourier Transform Block to replace the traditional Residual Block. Then, spectral normalization and Wasserstein distance are used to improve the speed and stability of GAN training. Experiments demonstrate that the methods we offer are effective at solving the problems listed above, and we get state-of-the-art scores in LPIPS and PSNR.
PDF This paper is accepted by IJCNN2022
论文截图
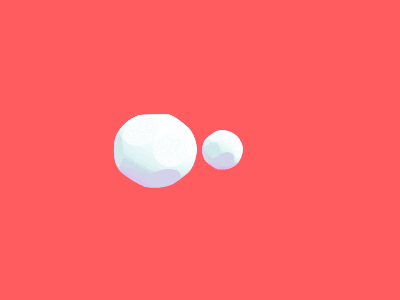
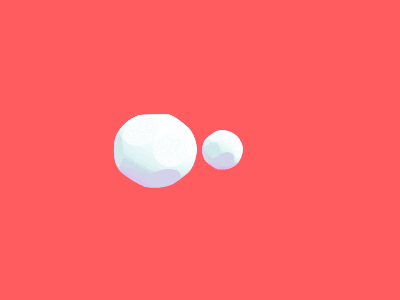
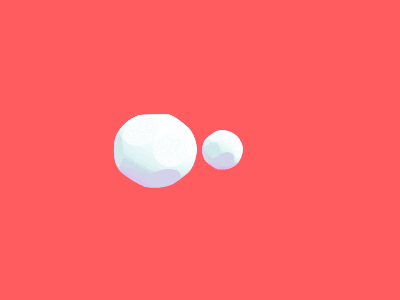
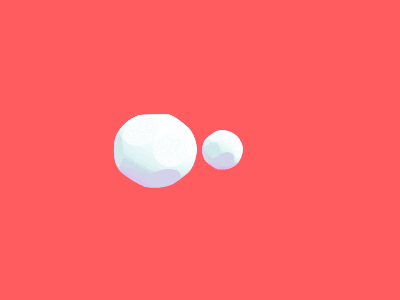
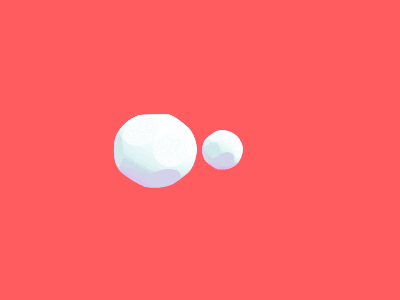
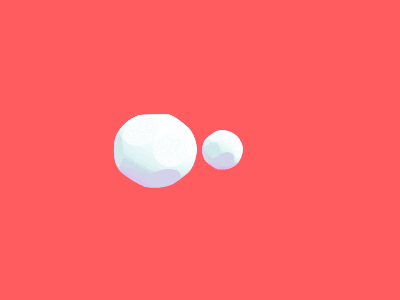
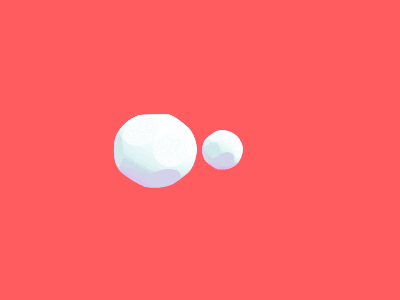
Region-aware Knowledge Distillation for Efficient Image-to-Image Translation
Authors:Linfeng Zhang, Xin Chen, Runpei Dong, Kaisheng Ma
Recent progress in image-to-image translation has witnessed the success of generative adversarial networks (GANs). However, GANs usually contain a huge number of parameters, which lead to intolerant memory and computation consumption and limit their deployment on edge devices. To address this issue, knowledge distillation is proposed to transfer the knowledge from a cumbersome teacher model to an efficient student model. However, most previous knowledge distillation methods are designed for image classification and lead to limited performance in image-to-image translation. In this paper, we propose Region-aware Knowledge Distillation ReKo to compress image-to-image translation models. Firstly, ReKo adaptively finds the crucial regions in the images with an attention module. Then, patch-wise contrastive learning is adopted to maximize the mutual information between students and teachers in these crucial regions. Experiments with eight comparison methods on nine datasets demonstrate the substantial effectiveness of ReKo on both paired and unpaired image-to-image translation. For instance, our 7.08X compressed and 6.80X accelerated CycleGAN student outperforms its teacher by 1.33 and 1.04 FID scores on Horse to Zebra and Zebra to Horse, respectively. Codes will be released on GitHub.
PDF
论文截图
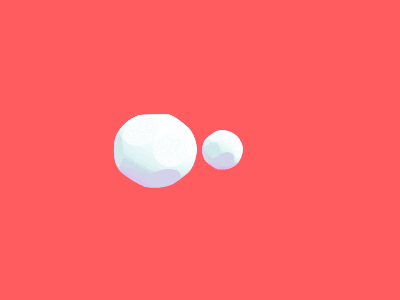
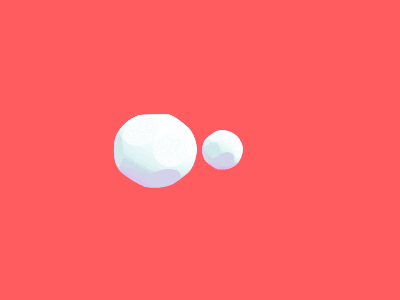
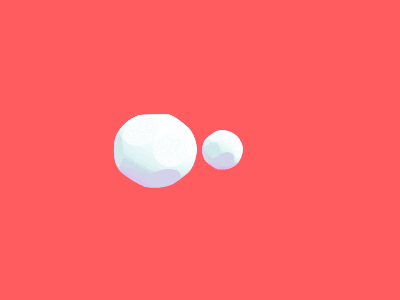
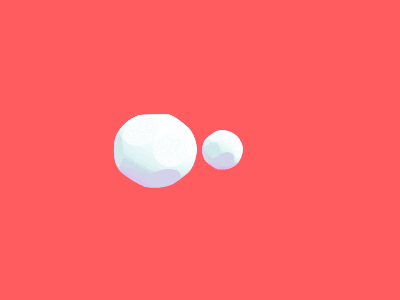
One-Shot Face Reenactment on Megapixels
Authors:Wonjun Kang, Geonsu Lee, Hyung Il Koo, Nam Ik Cho
The goal of face reenactment is to transfer a target expression and head pose to a source face while preserving the source identity. With the popularity of face-related applications, there has been much research on this topic. However, the results of existing methods are still limited to low-resolution and lack photorealism. In this work, we present a one-shot and high-resolution face reenactment method called MegaFR. To be precise, we leverage StyleGAN by using 3DMM-based rendering images and overcome the lack of high-quality video datasets by designing a loss function that works without high-quality videos. Also, we apply iterative refinement to deal with extreme poses and/or expressions. Since the proposed method controls source images through 3DMM parameters, we can explicitly manipulate source images. We apply MegaFR to various applications such as face frontalization, eye in-painting, and talking head generation. Experimental results show that our method successfully disentangles identity from expression and head pose, and outperforms conventional methods.
PDF 29 pages, 19 figures
论文截图
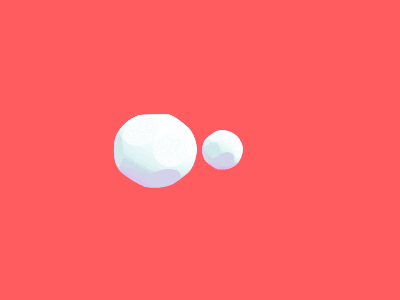
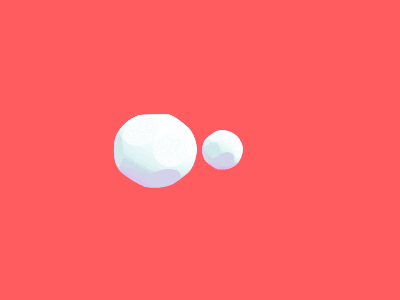
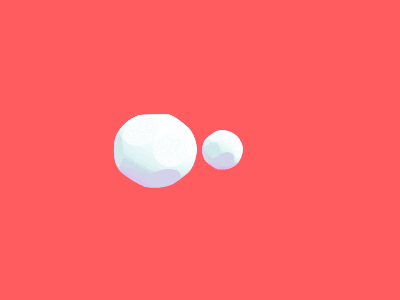
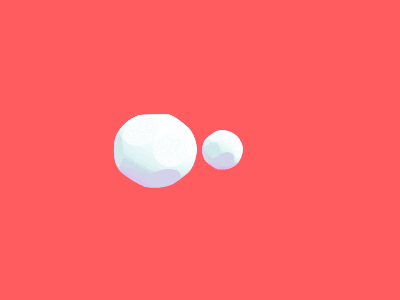
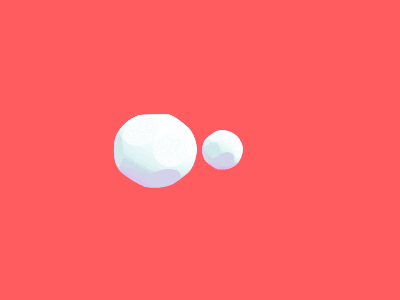
Analytical Interpretation of Latent Codes in InfoGAN with SAR Images
Authors:Zhenpeng Feng, Milos Dakovic, Hongbing Ji, Mingzhe Zhu, Ljubisa Stankovic
Generative Adversarial Networks (GANs) can synthesize abundant photo-realistic synthetic aperture radar (SAR) images. Some recent GANs (e.g., InfoGAN), are even able to edit specific properties of the synthesized images by introducing latent codes. It is crucial for SAR image synthesis since the targets in real SAR images are with different properties due to the imaging mechanism. Despite the success of InfoGAN in manipulating properties, there still lacks a clear explanation of how these latent codes affect synthesized properties, thus editing specific properties usually relies on empirical trials, unreliable and time-consuming. In this paper, we show that latent codes are disentangled to affect the properties of SAR images in a non-linear manner. By introducing some property estimators for latent codes, we are able to provide a completely analytical nonlinear model to decompose the entangled causality between latent codes and different properties. The qualitative and quantitative experimental results further reveal that the properties can be calculated by latent codes, inversely, the satisfying latent codes can be estimated given desired properties. In this case, properties can be manipulated by latent codes as we expect.
PDF 13 pages, 14 figures
论文截图
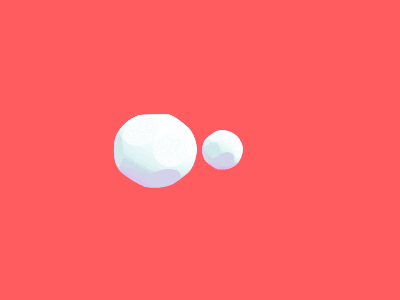
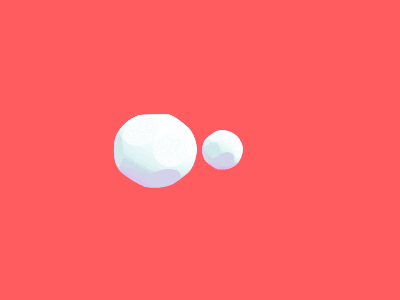
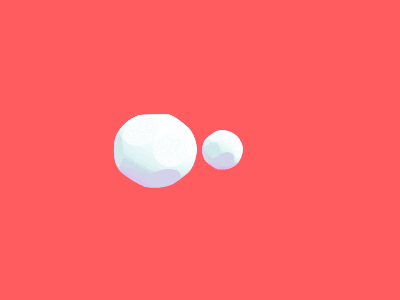
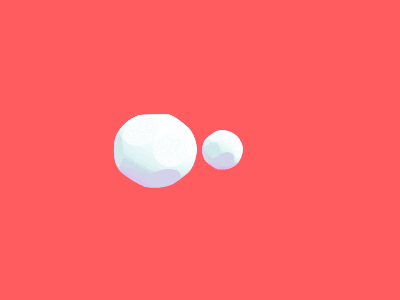
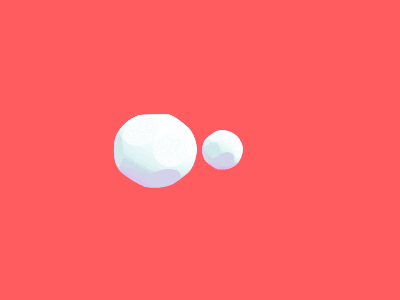
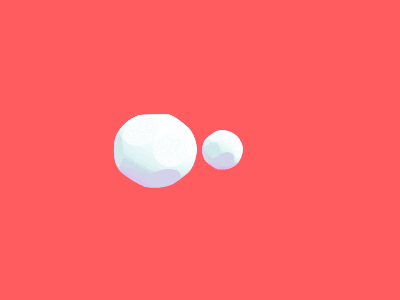
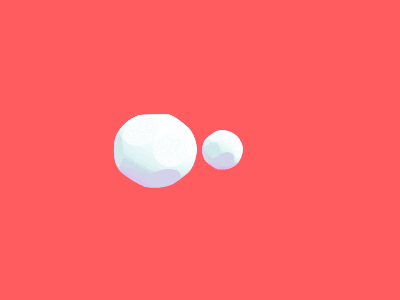
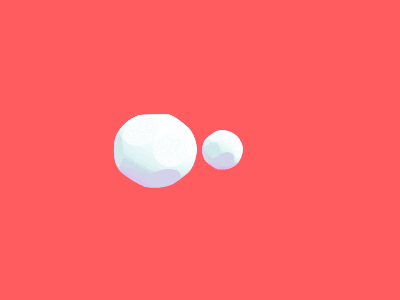
Text-to-Face Generation with StyleGAN2
Authors:D. M. A. Ayanthi, Sarasi Munasinghe
Synthesizing images from text descriptions has become an active research area with the advent of Generative Adversarial Networks. The main goal here is to generate photo-realistic images that are aligned with the input descriptions. Text-to-Face generation (T2F) is a sub-domain of Text-to-Image generation (T2I) that is more challenging due to the complexity and variation of facial attributes. It has a number of applications mainly in the domain of public safety. Even though several models are available for T2F, there is still the need to improve the image quality and the semantic alignment. In this research, we propose a novel framework, to generate facial images that are well-aligned with the input descriptions. Our framework utilizes the high-resolution face generator, StyleGAN2, and explores the possibility of using it in T2F. Here, we embed text in the input latent space of StyleGAN2 using BERT embeddings and oversee the generation of facial images using text descriptions. We trained our framework on attribute-based descriptions to generate images of 1024x1024 in resolution. The images generated exhibit a 57% similarity to the ground truth images, with a face semantic distance of 0.92, outperforming state-of-the-artwork. The generated images have a FID score of 118.097 and the experimental results show that our model generates promising images.
PDF 16 pages, 5 figures, for conference, https://aircconline.com/csit/papers/vol12/csit120805.pdf
论文截图
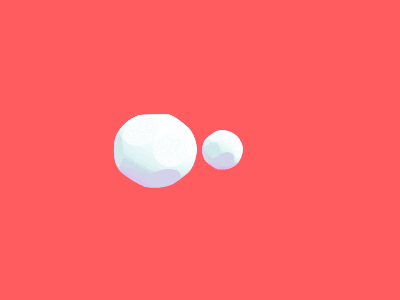
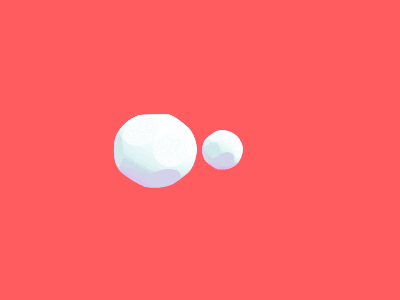
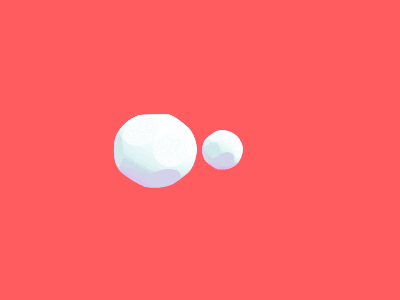
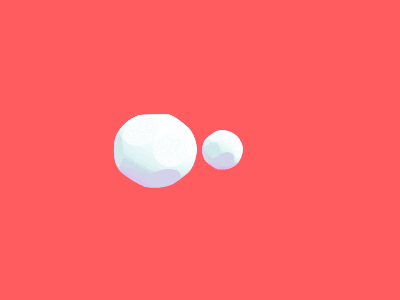
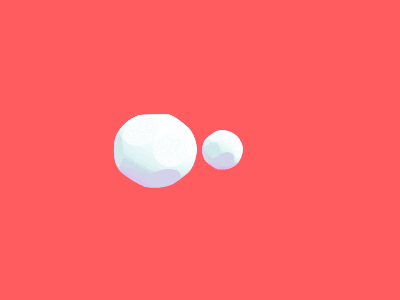
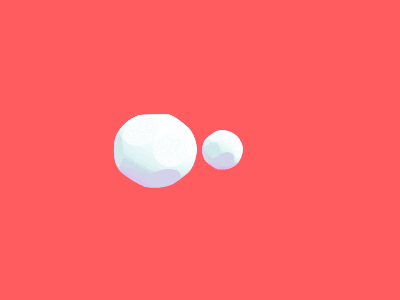
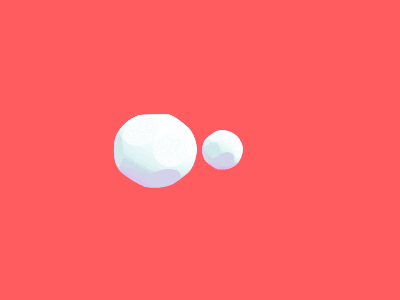