2022-05-24 更新
TRT-ViT: TensorRT-oriented Vision Transformer
Authors:Xin Xia, Jiashi Li, Jie Wu, Xing Wang, Xuefeng Xiao, Min Zheng, Rui Wang
We revisit the existing excellent Transformers from the perspective of practical application. Most of them are not even as efficient as the basic ResNets series and deviate from the realistic deployment scenario. It may be due to the current criterion to measure computation efficiency, such as FLOPs or parameters is one-sided, sub-optimal, and hardware-insensitive. Thus, this paper directly treats the TensorRT latency on the specific hardware as an efficiency metric, which provides more comprehensive feedback involving computational capacity, memory cost, and bandwidth. Based on a series of controlled experiments, this work derives four practical guidelines for TensorRT-oriented and deployment-friendly network design, e.g., early CNN and late Transformer at stage-level, early Transformer and late CNN at block-level. Accordingly, a family of TensortRT-oriented Transformers is presented, abbreviated as TRT-ViT. Extensive experiments demonstrate that TRT-ViT significantly outperforms existing ConvNets and vision Transformers with respect to the latency/accuracy trade-off across diverse visual tasks, e.g., image classification, object detection and semantic segmentation. For example, at 82.7% ImageNet-1k top-1 accuracy, TRT-ViT is 2.7$\times$ faster than CSWin and 2.0$\times$ faster than Twins. On the MS-COCO object detection task, TRT-ViT achieves comparable performance with Twins, while the inference speed is increased by 2.8$\times$.
PDF
论文截图
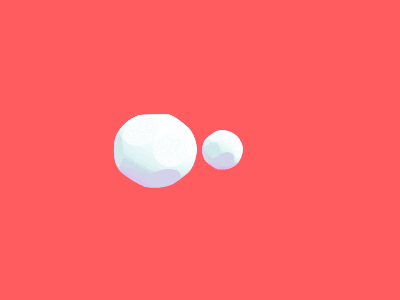
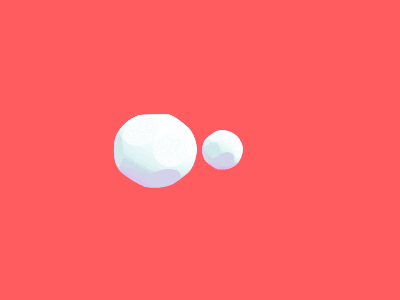
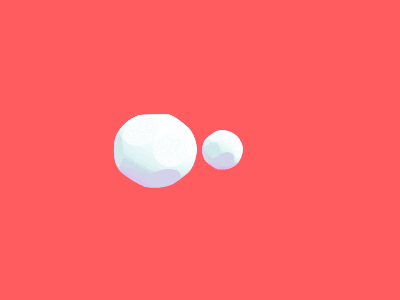
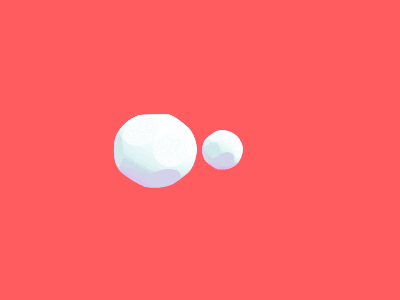
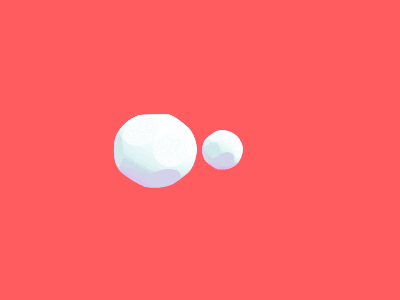
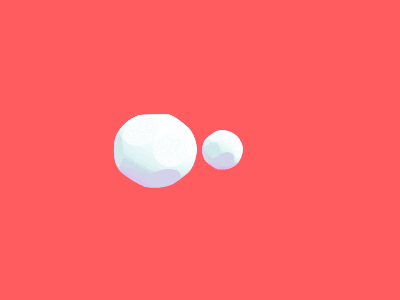
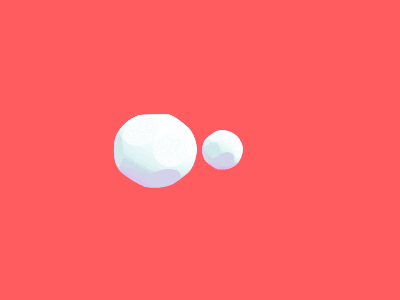
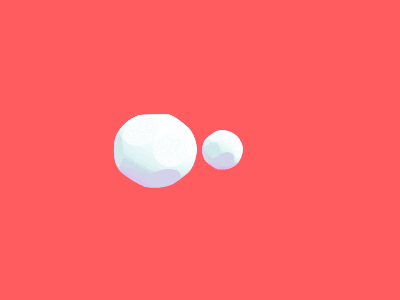
Supporting Vision-Language Model Inference with Causality-pruning Knowledge Prompt
Authors:Jiangmeng Li, Wenyi Mo, Wenwen Qiang, Bing Su, Changwen Zheng
Vision-language models are pre-trained by aligning image-text pairs in a common space so that the models can deal with open-set visual concepts by learning semantic information from textual labels. To boost the transferability of these models on downstream tasks in a zero-shot manner, recent works explore generating fixed or learnable prompts, i.e., classification weights are synthesized from natural language describing task-relevant categories, to reduce the gap between tasks in the training and test phases. However, how and what prompts can improve inference performance remains unclear. In this paper, we explicitly provide exploration and clarify the importance of including semantic information in prompts, while existing prompt methods generate prompts without exploring the semantic information of textual labels. A challenging issue is that manually constructing prompts, with rich semantic information, requires domain expertise and is extremely time-consuming. To this end, we propose Causality-pruning Knowledge Prompt (CapKP) for adapting pre-trained vision-language models to downstream image recognition. CapKP retrieves an ontological knowledge graph by treating the textual label as a query to explore task-relevant semantic information. To further refine the derived semantic information, CapKP introduces causality-pruning by following the first principle of Granger causality. Empirically, we conduct extensive evaluations to demonstrate the effectiveness of CapKP, e.g., with 8 shots, CapKP outperforms the manual-prompt method by 12.51% and the learnable-prompt method by 1.39% on average, respectively. Experimental analyses prove the superiority of CapKP in domain generalization compared to benchmark approaches.
PDF
论文截图
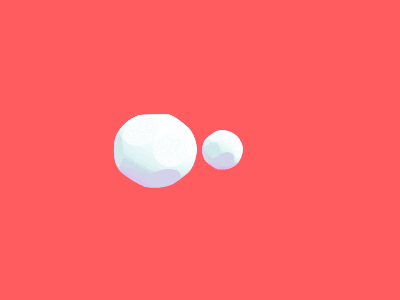
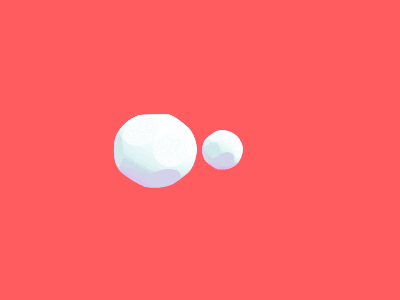
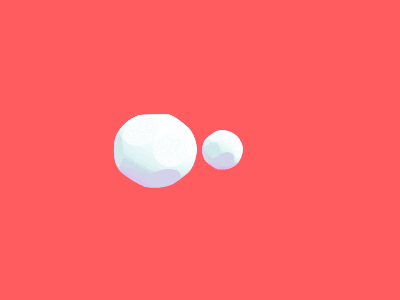
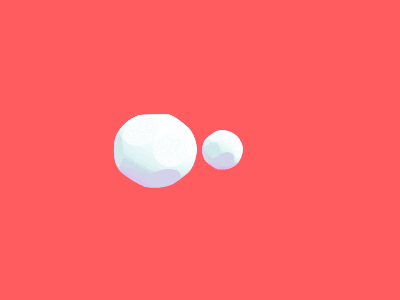
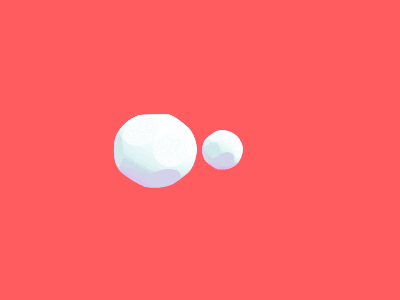
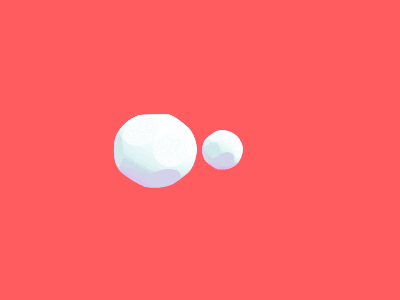
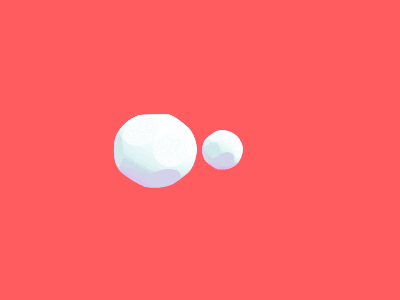
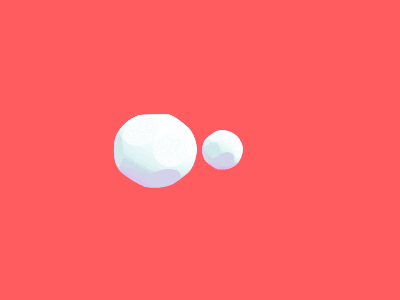
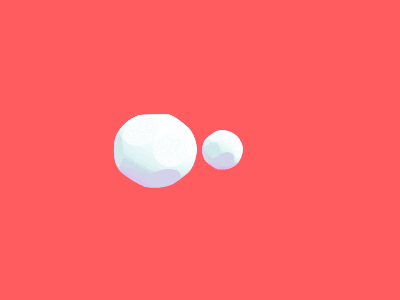
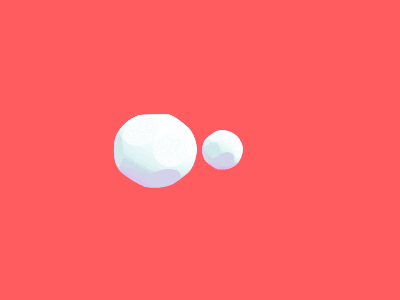
Vision Transformers in 2022: An Update on Tiny ImageNet
Authors:Ethan Huynh
The recent advances in image transformers have shown impressive results and have largely closed the gap between traditional CNN architectures. The standard procedure is to train on large datasets like ImageNet-21k and then finetune on ImageNet-1k. After finetuning, researches will often consider the transfer learning performance on smaller datasets such as CIFAR-10/100 but have left out Tiny ImageNet. This paper offers an update on vision transformers’ performance on Tiny ImageNet. I include Vision Transformer (ViT) , Data Efficient Image Transformer (DeiT), Class Attention in Image Transformer (CaiT), and Swin Transformers. In addition, Swin Transformers beats the current state-of-the-art result with a validation accuracy of 91.35%. Code is available here: https://github.com/ehuynh1106/TinyImageNet-Transformers
PDF
论文截图
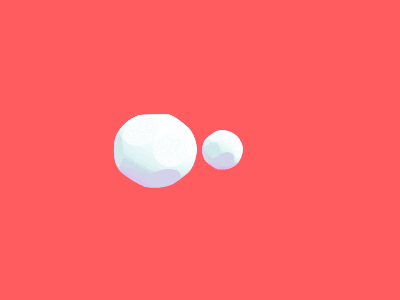
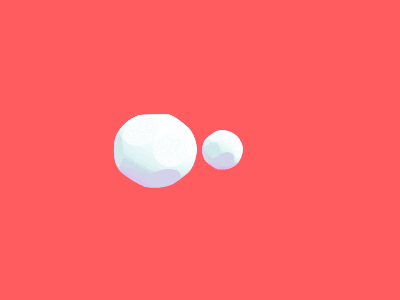
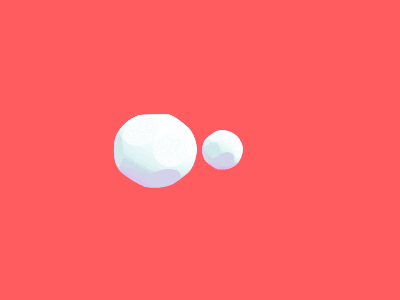
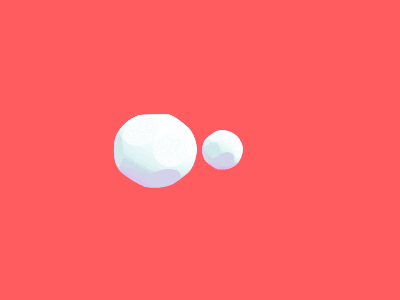
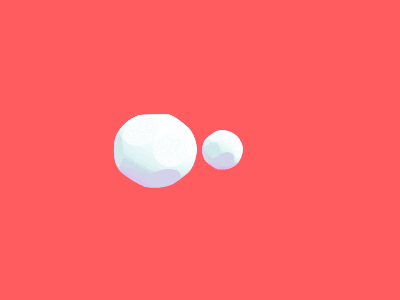
Super Vision Transformer
Authors:Mingbao Lin, Mengzhao Chen, Yuxin Zhang, Ke Li, Yunhang Shen, Chunhua Shen, Rongrong Ji
We attempt to reduce the computational costs in vision transformers (ViTs), which increase quadratically in the token number. We present a novel training paradigm that trains only one ViT model at a time, but is capable of providing improved image recognition performance with various computational costs. Here, the trained ViT model, termed super vision transformer (SuperViT), is empowered with the versatile ability to solve incoming patches of multiple sizes as well as preserve informative tokens with multiple keeping rates (the ratio of keeping tokens) to achieve good hardware efficiency for inference, given that the available hardware resources often change from time to time. Experimental results on ImageNet demonstrate that our SuperViT can considerably reduce the computational costs of ViT models with even performance increase. For example, we reduce 2x FLOPs of DeiT-S while increasing the Top-1 accuracy by 0.2% and 0.7% for 1.5x reduction. Also, our SuperViT significantly outperforms existing studies on efficient vision transformers. For example, when consuming the same amount of FLOPs, our SuperViT surpasses the recent state-of-the-art (SoTA) EViT by 1.1% when using DeiT-S as their backbones. The project of this work is made publicly available at https://github.com/lmbxmu/SuperViT.
PDF
论文截图
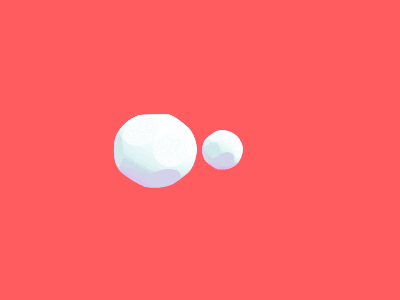
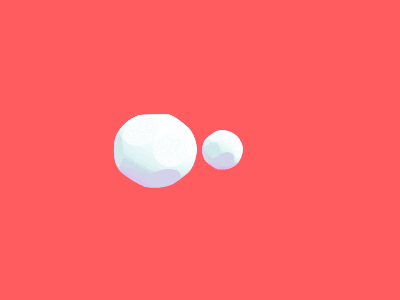
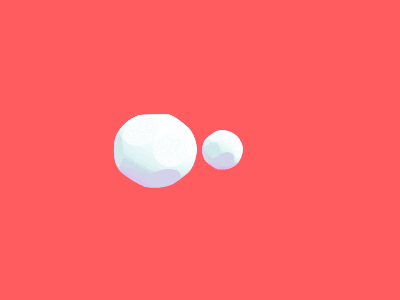
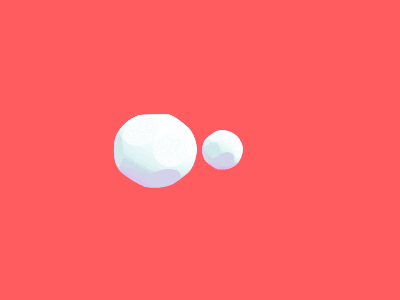
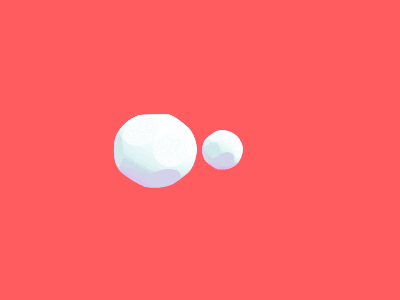
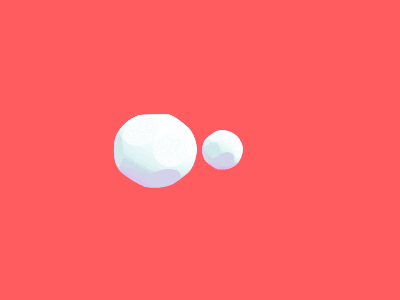
RestoreFormer: High-Quality Blind Face Restoration from Undegraded Key-Value Pairs
Authors:Zhouxia Wang, Jiawei Zhang, Runjian Chen, Wenping Wang, Ping Luo
Blind face restoration is to recover a high-quality face image from unknown degradations. As face image contains abundant contextual information, we propose a method, RestoreFormer, which explores fully-spatial attentions to model contextual information and surpasses existing works that use local operators. RestoreFormer has several benefits compared to prior arts. First, unlike the conventional multi-head self-attention in previous Vision Transformers (ViTs), RestoreFormer incorporates a multi-head cross-attention layer to learn fully-spatial interactions between corrupted queries and high-quality key-value pairs. Second, the key-value pairs in ResotreFormer are sampled from a reconstruction-oriented high-quality dictionary, whose elements are rich in high-quality facial features specifically aimed for face reconstruction, leading to superior restoration results. Third, RestoreFormer outperforms advanced state-of-the-art methods on one synthetic dataset and three real-world datasets, as well as produces images with better visual quality.
PDF Accepted by CVPR 2022
论文截图
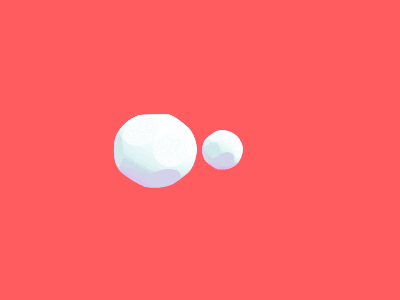
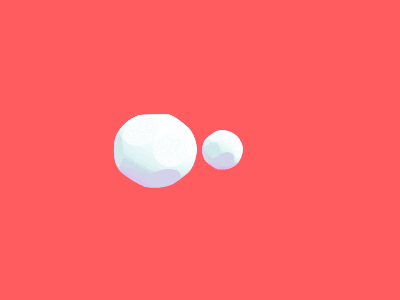
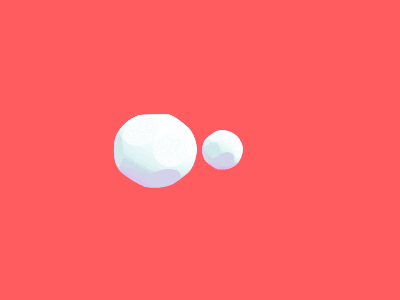
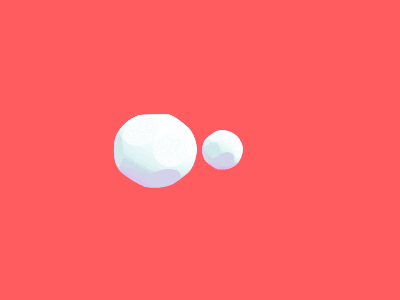
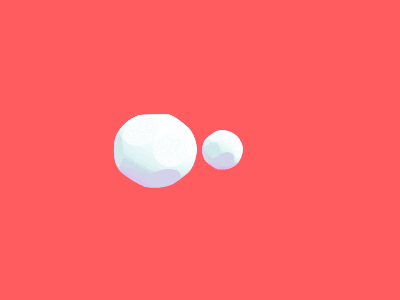
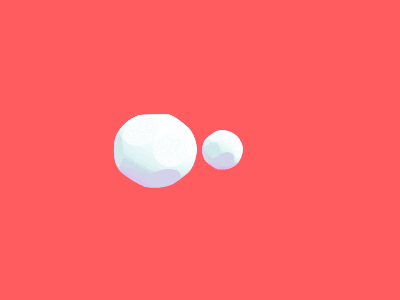