2022-05-23 更新
Uncertainty-aware Contrastive Distillation for Incremental Semantic Segmentation
Authors:Guanglei Yang, Enrico Fini, Dan Xu, Paolo Rota, Mingli Ding, Moin Nabi, Xavier Alameda-Pineda, Elisa Ricci
A fundamental and challenging problem in deep learning is catastrophic forgetting, i.e. the tendency of neural networks to fail to preserve the knowledge acquired from old tasks when learning new tasks. This problem has been widely investigated in the research community and several Incremental Learning (IL) approaches have been proposed in the past years. While earlier works in computer vision have mostly focused on image classification and object detection, more recently some IL approaches for semantic segmentation have been introduced. These previous works showed that, despite its simplicity, knowledge distillation can be effectively employed to alleviate catastrophic forgetting. In this paper, we follow this research direction and, inspired by recent literature on contrastive learning, we propose a novel distillation framework, Uncertainty-aware Contrastive Distillation (\method). In a nutshell, \method~is operated by introducing a novel distillation loss that takes into account all the images in a mini-batch, enforcing similarity between features associated to all the pixels from the same classes, and pulling apart those corresponding to pixels from different classes. In order to mitigate catastrophic forgetting, we contrast features of the new model with features extracted by a frozen model learned at the previous incremental step. Our experimental results demonstrate the advantage of the proposed distillation technique, which can be used in synergy with previous IL approaches, and leads to state-of-art performance on three commonly adopted benchmarks for incremental semantic segmentation. The code is available at \url{https://github.com/ygjwd12345/UCD}.
PDF TPAMI
论文截图
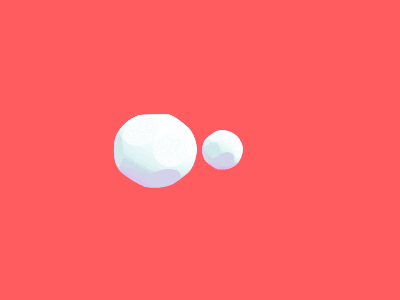
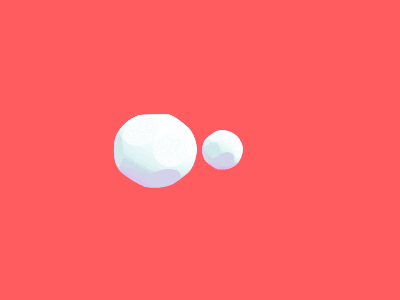
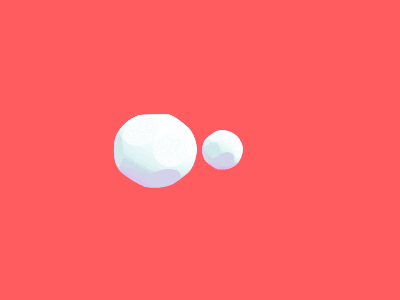
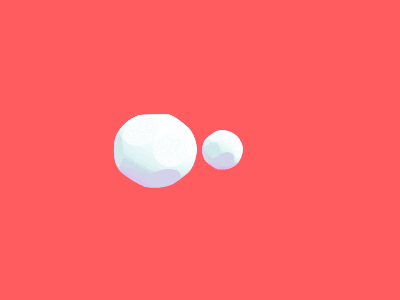
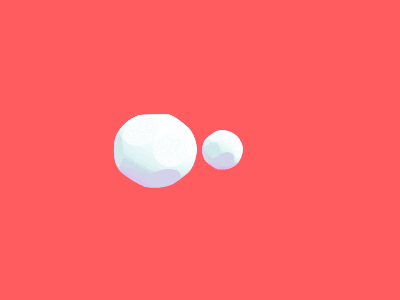
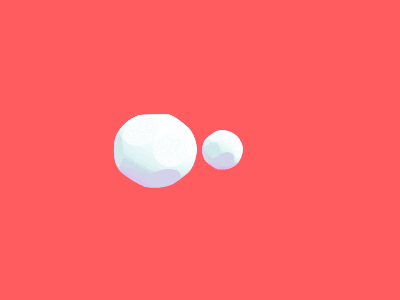
Synthesis in Style: Semantic Segmentation of Historical Documents using Synthetic Data
Authors:Christian Bartz, Hendrik Raetz, Jona Otholt, Christoph Meinel, Haojin Yang
One of the most pressing problems in the automated analysis of historical documents is the availability of annotated training data. The problem is that labeling samples is a time-consuming task because it requires human expertise and thus, cannot be automated well. In this work, we propose a novel method to construct synthetic labeled datasets for historical documents where no annotations are available. We train a StyleGAN model to synthesize document images that capture the core features of the original documents. While originally, the StyleGAN architecture was not intended to produce labels, it indirectly learns the underlying semantics to generate realistic images. Using our approach, we can extract the semantic information from the intermediate feature maps and use it to generate ground truth labels. To investigate if our synthetic dataset can be used to segment the text in historical documents, we use it to train multiple supervised segmentation models and evaluate their performance. We also train these models on another dataset created by a state-of-the-art synthesis approach to show that the models trained on our dataset achieve better results while requiring even less human annotation effort.
PDF Code available at: https://github.com/hendraet/synthesis-in-style
论文截图
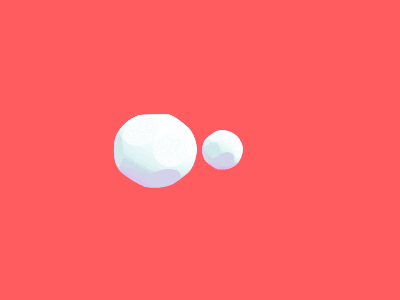
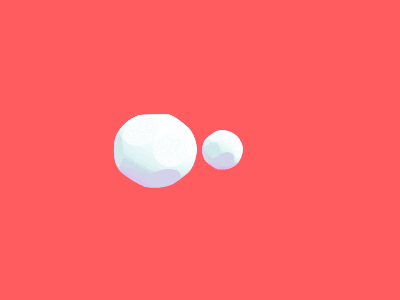
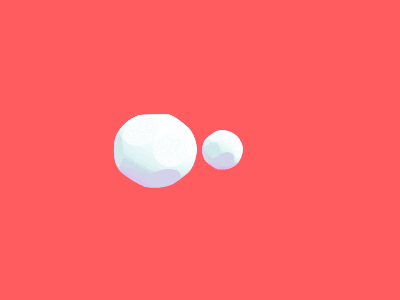
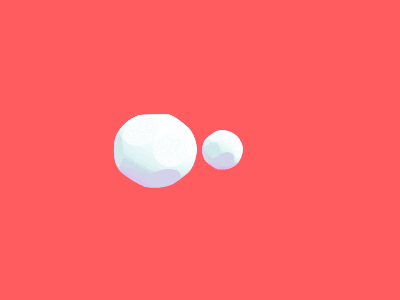
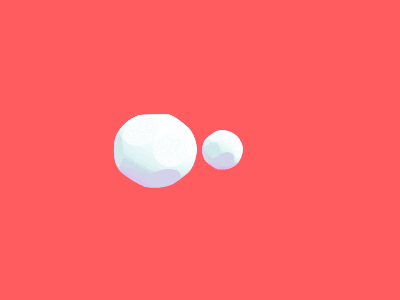
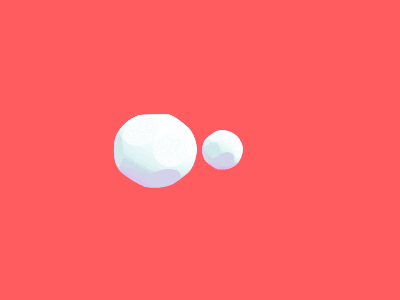
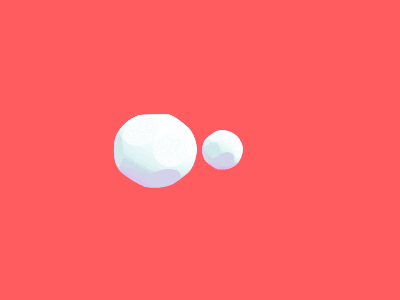
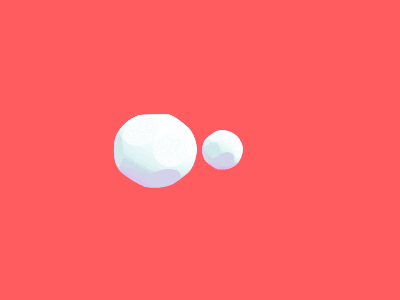
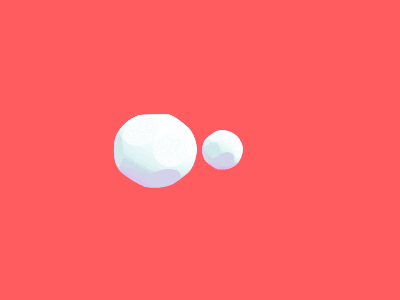