2022-05-16 更新
Contrastive Domain Disentanglement for Generalizable Medical Image Segmentation
Authors:Ran Gu, Jiangshan Lu, Jingyang Zhang, Wenhui Lei, Xiaofan Zhang, Guotai Wang, Shaoting Zhang
Efficiently utilizing discriminative features is crucial for convolutional neural networks to achieve remarkable performance in medical image segmentation and is also important for model generalization across multiple domains, where letting model recognize domain-specific and domain-invariant information among multi-site datasets is a reasonable strategy for domain generalization. Unfortunately, most of the recent disentangle networks are not directly adaptable to unseen-domain datasets because of the limitations of offered data distribution. To tackle this deficiency, we propose Contrastive Domain Disentangle (CDD) network for generalizable medical image segmentation. We first introduce a disentangle network to decompose medical images into an anatomical representation factor and a modality representation factor. Then, a style contrastive loss is proposed to encourage the modality representations from the same domain to distribute as close as possible while different domains are estranged from each other. Finally, we propose a domain augmentation strategy that can randomly generate new domains for model generalization training. Experimental results on multi-site fundus image datasets for optic cup and disc segmentation show that the CDD has good model generalization. Our proposed CDD outperforms several state-of-the-art methods in domain generalizable segmentation.
PDF 10 pages, 2 figures
论文截图
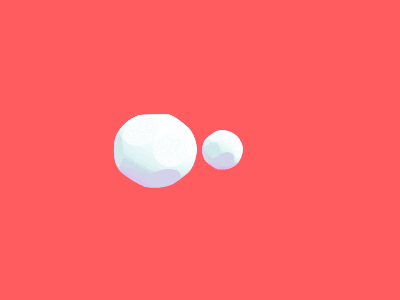
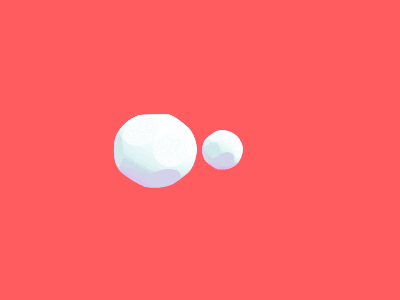
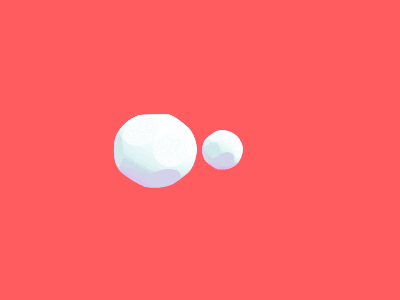
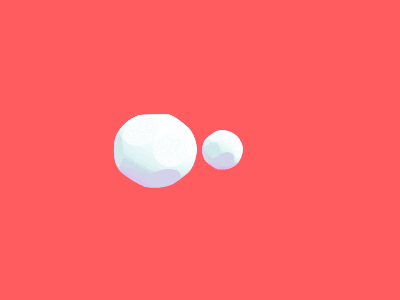
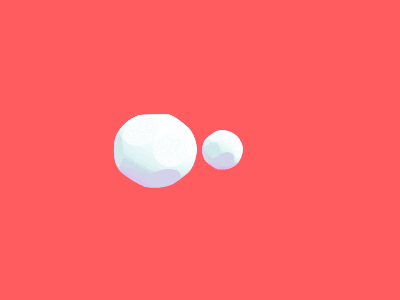