2022-05-09 更新
Data Determines Distributional Robustness in Contrastive Language Image Pre-training (CLIP)
Authors:Alex Fang, Gabriel Ilharco, Mitchell Wortsman, Yuhao Wan, Vaishaal Shankar, Achal Dave, Ludwig Schmidt
Contrastively trained image-text models such as CLIP, ALIGN, and BASIC have demonstrated unprecedented robustness to multiple challenging natural distribution shifts. Since these image-text models differ from previous training approaches in several ways, an important question is what causes the large robustness gains. We answer this question via a systematic experimental investigation. Concretely, we study five different possible causes for the robustness gains: (i) the training set size, (ii) the training distribution, (iii) language supervision at training time, (iv) language supervision at test time, and (v) the contrastive loss function. Our experiments show that the more diverse training distribution is the main cause for the robustness gains, with the other factors contributing little to no robustness. Beyond our experimental results, we also introduce ImageNet-Captions, a version of ImageNet with original text annotations from Flickr, to enable further controlled experiments of language-image training.
PDF
论文截图
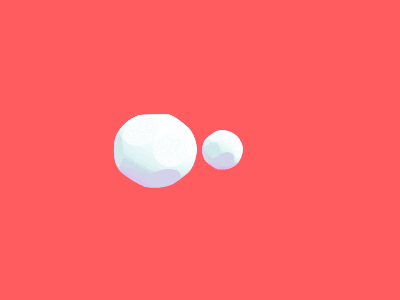
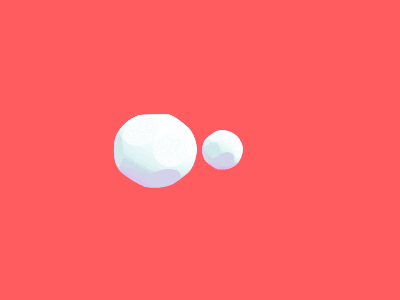
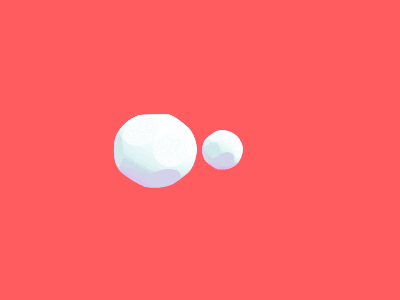
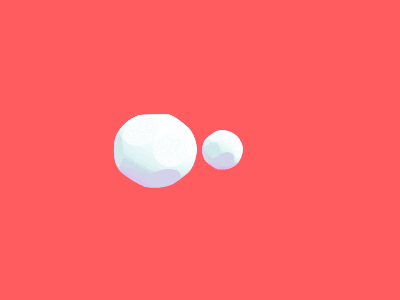
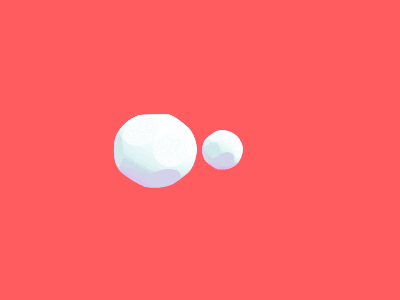
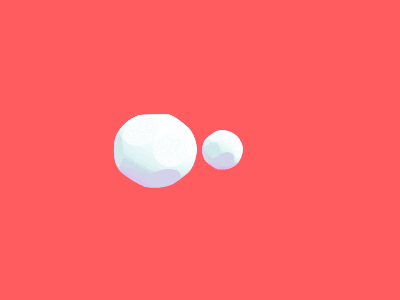
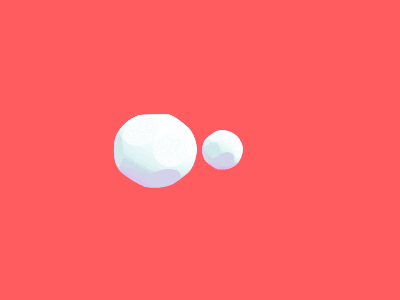
Zero-Episode Few-Shot Contrastive Predictive Coding: Solving intelligence tests without prior training
Authors:T. Barak, Y. Loewenstein
Video prediction models often combine three components: an encoder from pixel space to a small latent space, a latent space prediction model, and a generative model back to pixel space. However, the large and unpredictable pixel space makes training such models difficult, requiring many training examples. We argue that finding a predictive latent variable and using it to evaluate the consistency of a future image enables data-efficient predictions because it precludes the necessity of a generative model training. To demonstrate it, we created sequence completion intelligence tests in which the task is to identify a predictably changing feature in a sequence of images and use this prediction to select the subsequent image. We show that a one-dimensional Markov Contrastive Predictive Coding (M-CPC_1D) model solves these tests efficiently, with only five examples. Finally, we demonstrate the usefulness of M-CPC_1D in solving two tasks without prior training: anomaly detection and stochastic movement video prediction.
PDF
论文截图
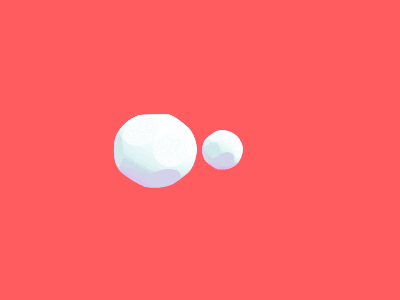
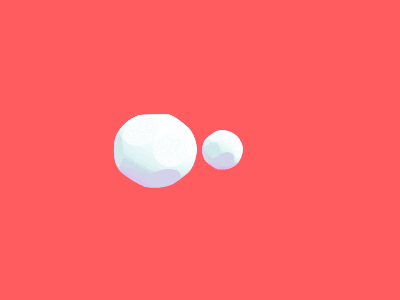
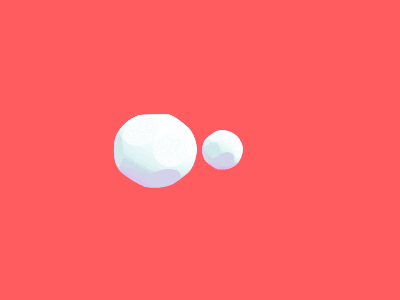
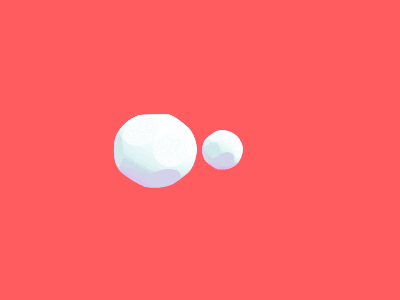
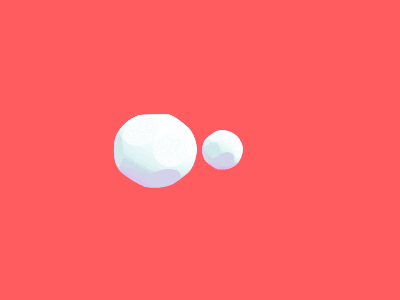
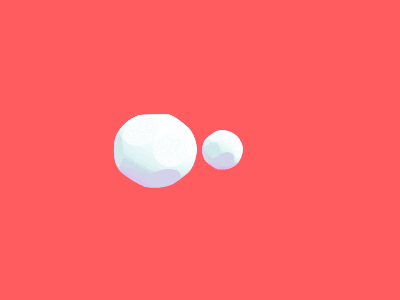
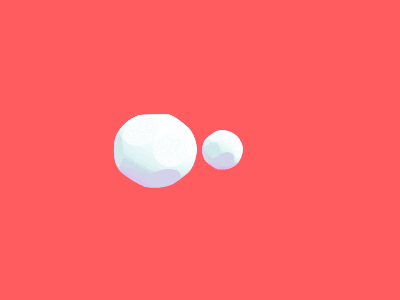
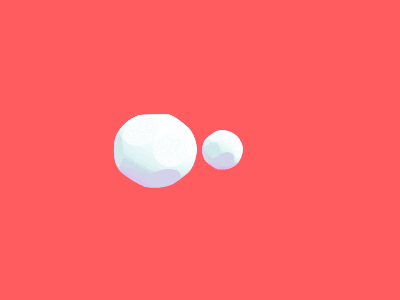
Residual Contrastive Learning for Image Reconstruction: Learning Transferable Representations from Noisy Images
Authors:Nanqing Dong, Matteo Maggioni, Yongxin Yang, Eduardo Pérez-Pellitero, Ales Leonardis, Steven McDonagh
This paper is concerned with contrastive learning (CL) for low-level image restoration and enhancement tasks. We propose a new label-efficient learning paradigm based on residuals, residual contrastive learning (RCL), and derive an unsupervised visual representation learning framework, suitable for low-level vision tasks with noisy inputs. While supervised image reconstruction aims to minimize residual terms directly, RCL alternatively builds a connection between residuals and CL by defining a novel instance discrimination pretext task, using residuals as the discriminative feature. Our formulation mitigates the severe task misalignment between instance discrimination pretext tasks and downstream image reconstruction tasks, present in existing CL frameworks. Experimentally, we find that RCL can learn robust and transferable representations that improve the performance of various downstream tasks, such as denoising and super resolution, in comparison with recent self-supervised methods designed specifically for noisy inputs. Additionally, our unsupervised pre-training can significantly reduce annotation costs whilst maintaining performance competitive with fully-supervised image reconstruction.
PDF Accepted by IJCAI 2022
论文截图
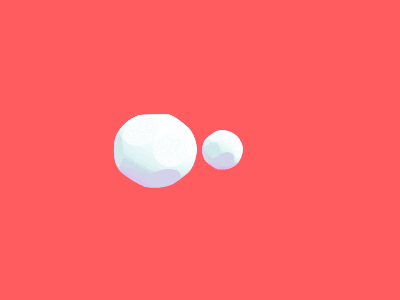
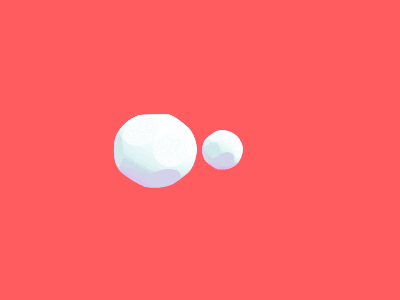
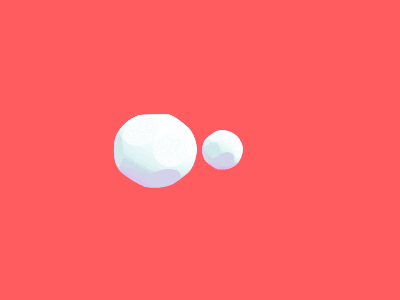
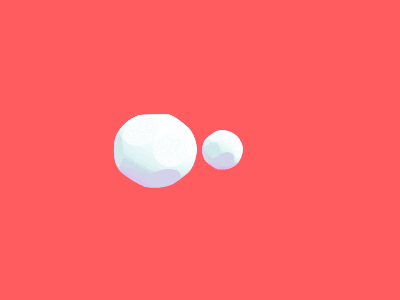
Keep the Caption Information: Preventing Shortcut Learning in Contrastive Image-Caption Retrieval
Authors:Maurits Bleeker, Andrew Yates, Maarten de Rijke
To train image-caption retrieval (ICR) methods, contrastive loss functions are a common choice for optimization functions. Unfortunately, contrastive ICR methods are vulnerable to learning shortcuts: decision rules that perform well on the training data but fail to transfer to other testing conditions. We introduce an approach to reduce shortcut feature representations for the ICR task: latent target decoding (LTD). We add an additional decoder to the learning framework to reconstruct the input caption, which prevents the image and caption encoder from learning shortcut features. Instead of reconstructing input captions in the input space, we decode the semantics of the caption in a latent space. We implement the LTD objective as an optimization constraint, to ensure that the reconstruction loss is below a threshold value while primarily optimizing for the contrastive loss. Importantly, LTD does not depend on additional training data or expensive (hard) negative mining strategies. Our experiments show that, unlike reconstructing the input caption, LTD reduces shortcut learning and improves generalizability by obtaining higher recall@k and r-precision scores. Additionally, we show that the evaluation scores benefit from implementing LTD as an optimization constraint instead of a dual loss.
PDF Preprint
论文截图
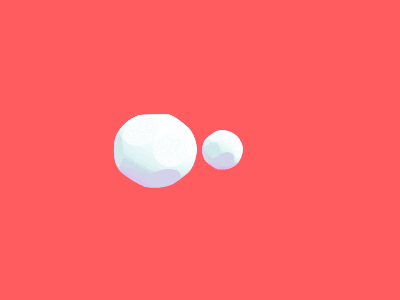
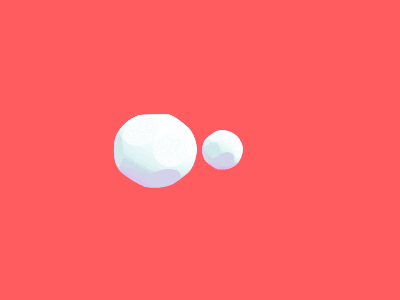
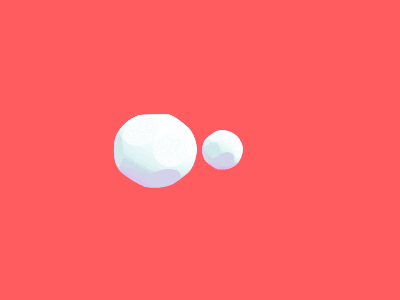
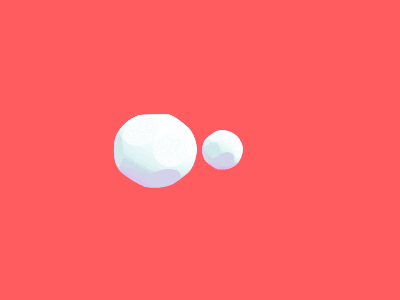
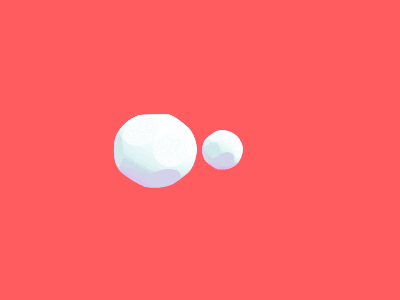