2022-04-29 更新
TransCenter: Transformers with Dense Representations for Multiple-Object Tracking
Authors:Yihong Xu, Yutong Ban, Guillaume Delorme, Chuang Gan, Daniela Rus, Xavier Alameda-Pineda
Transformers have proven superior performance for a wide variety of tasks since they were introduced, which has drawn in recent years the attention of the vision community where efforts were made such as image classification and object detection. Despite this wave, building an accurate and efficient multiple-object tracking (MOT) method with transformers is not a trivial task. We argue that the direct application of a transformer architecture with quadratic complexity and insufficient noise-initialized sparse queries — is not optimal for MOT. Inspired by recent research, we propose TransCenter, a transformer-based MOT architecture with dense representations for accurately tracking all the objects while keeping a reasonable runtime. Methodologically, we propose the use of dense image-related multi-scale detection queries produced by an efficient transformer architecture. The queries allow inferring targets’ locations globally and robustly from dense heatmap outputs. In parallel, a set of efficient sparse tracking queries interacting with image features in the TransCenter Decoder to associate object positions through time. TransCenter exhibits remarkable performance improvements and outperforms by a large margin the current state-of-the-art in two standard MOT benchmarks with two tracking (public/private) settings. The proposed efficient and accurate transformer architecture for MOT is proven with an extensive ablation study, demonstrating its advantage compared to more naive alternatives and concurrent works. The code will be made publicly available at https://github.com/yihongxu/transcenter.
PDF 16 pages, 10 figures, updated results
论文截图
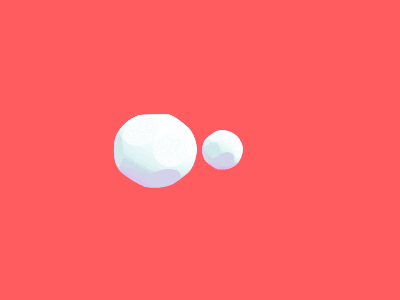
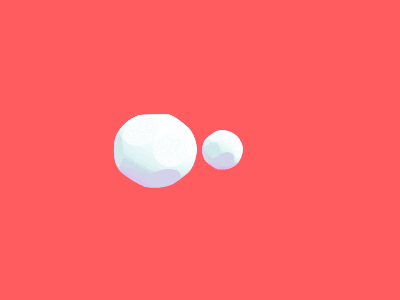
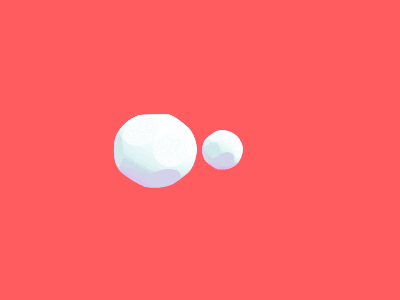
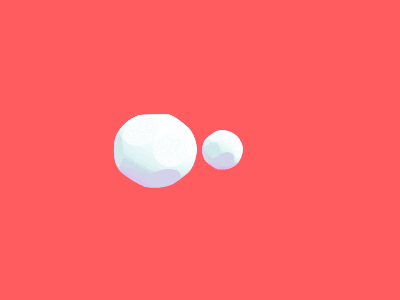
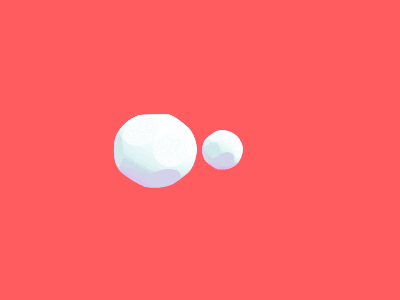
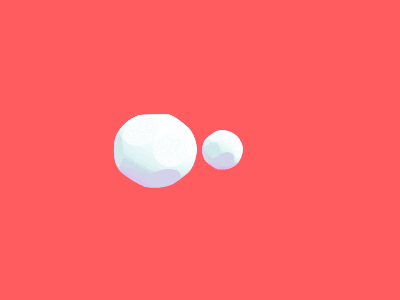
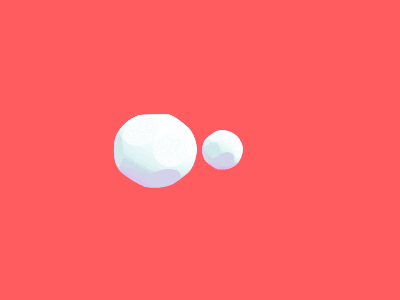
HRDA: Context-Aware High-Resolution Domain-Adaptive Semantic Segmentation
Authors:Lukas Hoyer, Dengxin Dai, Luc Van Gool
Unsupervised domain adaptation (UDA) aims to adapt a model trained on the source domain (e.g. synthetic data) to the target domain (e.g. real-world data) without requiring further annotations on the target domain. This work focuses on UDA for semantic segmentation as real-world pixel-wise annotations are particularly expensive to acquire. As UDA methods for semantic segmentation are usually GPU memory intensive, most previous methods operate only on downscaled images. We question this design as low-resolution predictions often fail to preserve fine details. The alternative of training with random crops of high-resolution images alleviates this problem but falls short in capturing long-range, domain-robust context information. Therefore, we propose HRDA, a multi-resolution training approach for UDA, that combines the strengths of small high-resolution crops to preserve fine segmentation details and large low-resolution crops to capture long-range context dependencies with a learned scale attention, while maintaining a manageable GPU memory footprint. HRDA enables adapting small objects and preserving fine segmentation details. It significantly improves the state-of-the-art performance by 5.5 mIoU for GTA-to-Cityscapes and 4.9 mIoU for Synthia-to-Cityscapes, resulting in unprecedented 73.8 and 65.8 mIoU, respectively. The implementation is available at https://github.com/lhoyer/HRDA.
PDF
论文截图
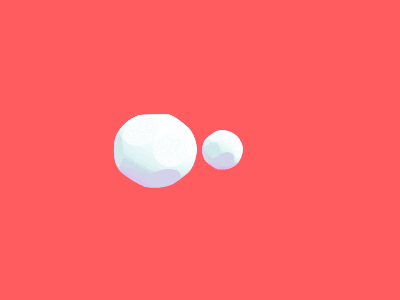
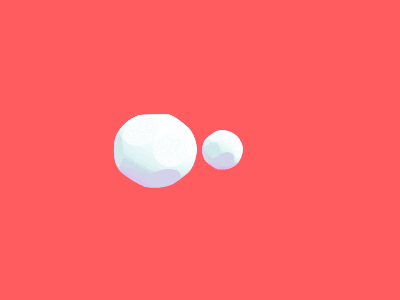
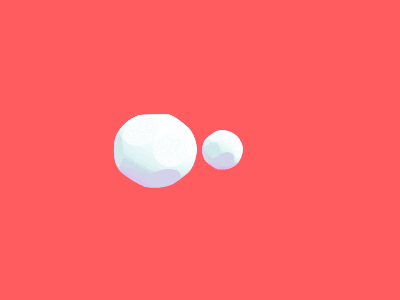
MDS-Net: A Multi-scale Depth Stratification Based Monocular 3D Object Detection Algorithm
Authors:Zhouzhen Xie, Yuying Song, Jingxuan Wu, Zecheng Li, Chunyi Song, Zhiwei Xu
Monocular 3D object detection is very challenging in autonomous driving due to the lack of depth information. This paper proposes a one-stage monocular 3D object detection algorithm based on multi-scale depth stratification, which uses the anchor-free method to detect 3D objects in a per-pixel prediction. In the proposed MDS-Net, a novel depth-based stratification structure is developed to improve the network’s ability of depth prediction by establishing mathematical models between depth and image size of objects. A new angle loss function is then developed to further improve the accuracy of the angle prediction and increase the convergence speed of training. An optimized soft-NMS is finally applied in the post-processing stage to adjust the confidence of candidate boxes. Experiments on the KITTI benchmark show that the MDS-Net outperforms the existing monocular 3D detection methods in 3D detection and BEV detection tasks while fulfilling real-time requirements.
PDF 9 pages
论文截图
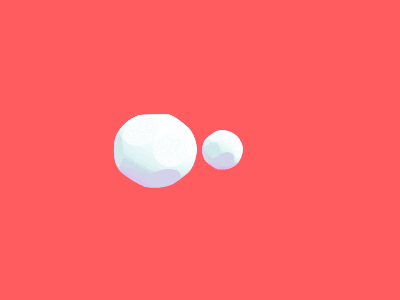
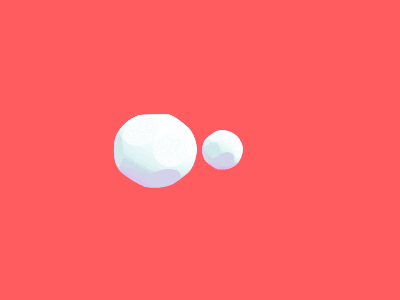
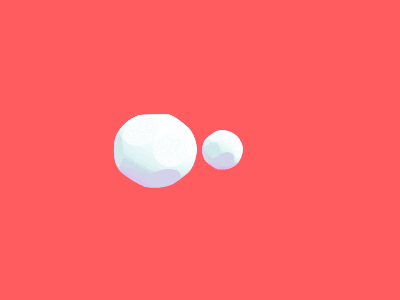
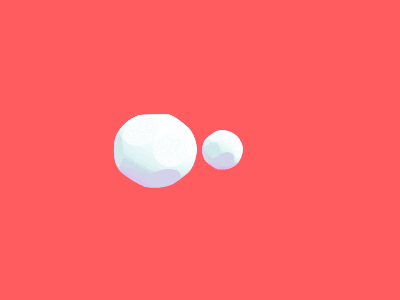
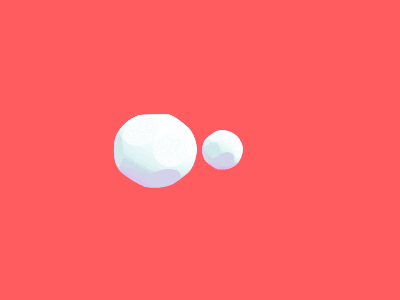
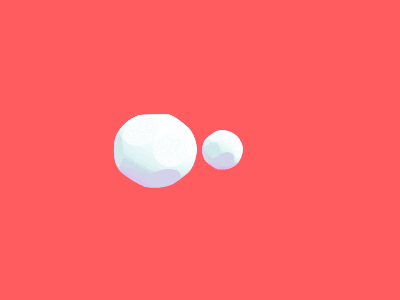
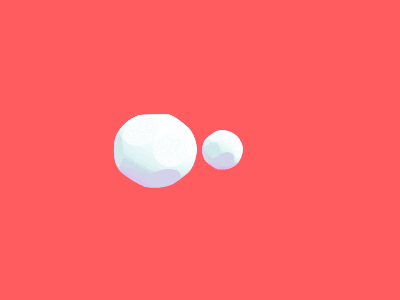
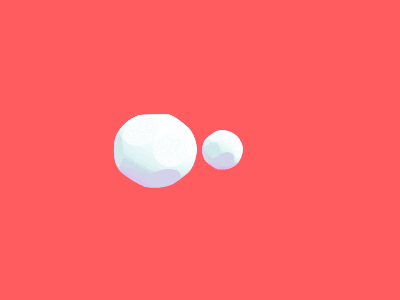