2022-04-15 更新
Distill and De-bias: Mitigating Bias in Face Recognition using Knowledge Distillation
Authors:Prithviraj Dhar, Joshua Gleason, Aniket Roy, Carlos D. Castillo, P. Jonathon Phillips, Rama Chellappa
Face recognition networks generally demonstrate bias with respect to sensitive attributes like gender, skintone etc. For gender and skintone, we observe that the regions of the face that a network attends to vary by the category of an attribute. This might contribute to bias. Building on this intuition, we propose a novel distillation-based approach called Distill and De-bias (D&D) to enforce a network to attend to similar face regions, irrespective of the attribute category. In D&D, we train a teacher network on images from one category of an attribute; e.g. light skintone. Then distilling information from the teacher, we train a student network on images of the remaining category; e.g., dark skintone. A feature-level distillation loss constrains the student network to generate teacher-like representations. This allows the student network to attend to similar face regions for all attribute categories and enables it to reduce bias. We also propose a second distillation step on top of D&D, called D&D++. For the D&D++ network, we distill the `un-biasedness’ of the D&D network into a new student network, the D&D++ network. We train the new network on all attribute categories; e.g., both light and dark skintones. This helps us train a network that is less biased for an attribute, while obtaining higher face verification performance than D&D. We show that D&D++ outperforms existing baselines in reducing gender and skintone bias on the IJB-C dataset, while obtaining higher face verification performance than existing adversarial de-biasing methods. We evaluate the effectiveness of our proposed methods on two state-of-the-art face recognition networks: Crystalface and ArcFace.
PDF
论文截图
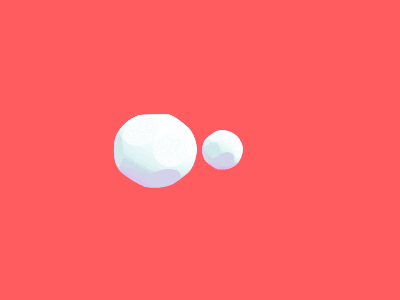
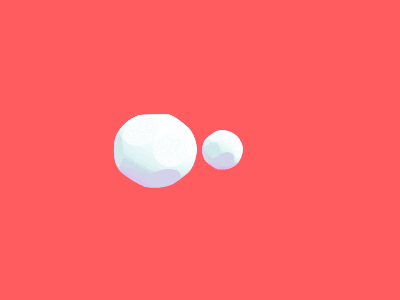
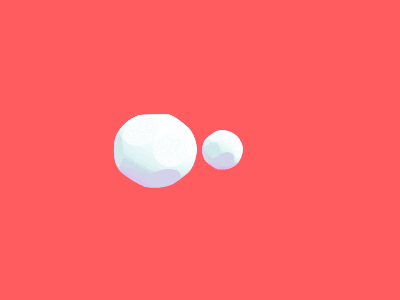
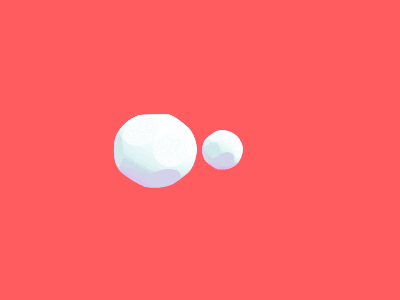
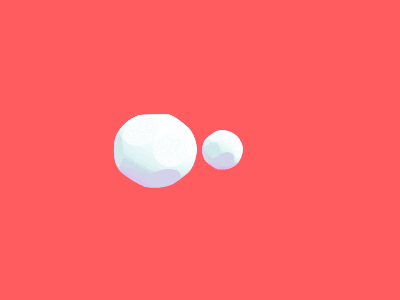
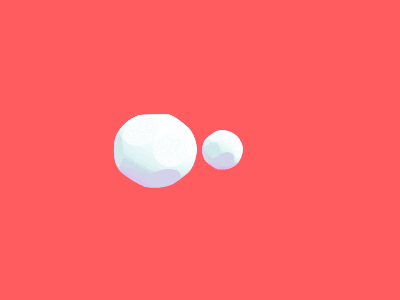
Federated Unsupervised Domain Adaptation for Face Recognition
Authors:Weiming Zhuang, Xin Gan, Yonggang Wen, Xuesen Zhang, Shuai Zhang, Shuai Yi
Given labeled data in a source domain, unsupervised domain adaptation has been widely adopted to generalize models for unlabeled data in a target domain, whose data distributions are different. However, existing works are inapplicable to face recognition under privacy constraints because they require sharing of sensitive face images between domains. To address this problem, we propose federated unsupervised domain adaptation for face recognition, FedFR. FedFR jointly optimizes clustering-based domain adaptation and federated learning to elevate performance on the target domain. Specifically, for unlabeled data in the target domain, we enhance a clustering algorithm with distance constrain to improve the quality of predicted pseudo labels. Besides, we propose a new domain constraint loss (DCL) to regularize source domain training in federated learning. Extensive experiments on a newly constructed benchmark demonstrate that FedFR outperforms the baseline and classic methods on the target domain by 3% to 14% on different evaluation metrics.
PDF ICME’22. arXiv admin note: substantial text overlap with arXiv:2105.07606
论文截图
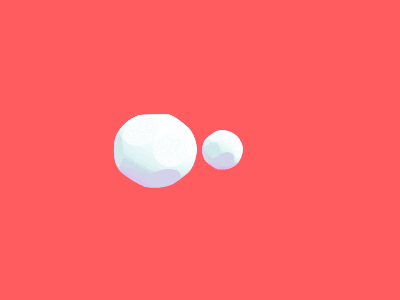
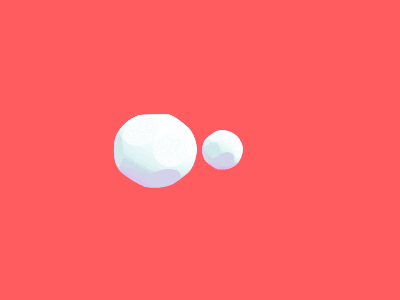
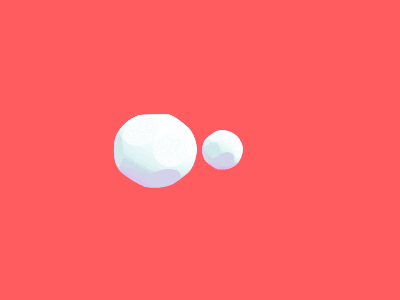
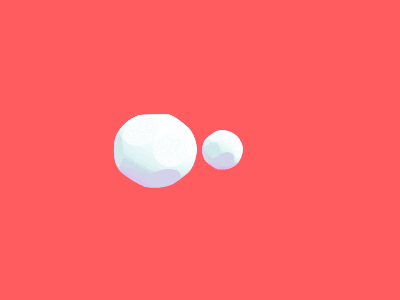
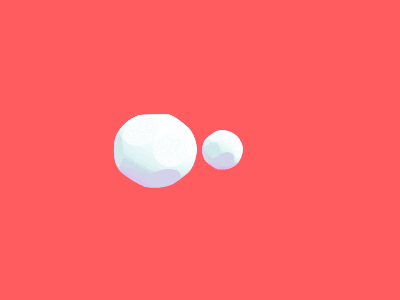
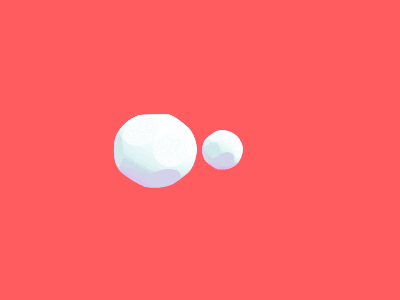