2022-04-13 更新
Self-supervised Vision Transformers for Joint SAR-optical Representation Learning
Authors:Yi Wang, Conrad M Albrecht, Xiao Xiang Zhu
Self-supervised learning (SSL) has attracted much interest in remote sensing and earth observation due to its ability to learn task-agnostic representations without human annotation. While most of the existing SSL works in remote sensing utilize ConvNet backbones and focus on a single modality, we explore the potential of vision transformers (ViTs) for joint SAR-optical representation learning. Based on DINO, a state-of-the-art SSL algorithm that distills knowledge from two augmented views of an input image, we combine SAR and optical imagery by concatenating all channels to a unified input. Subsequently, we randomly mask out channels of one modality as a data augmentation strategy. While training, the model gets fed optical-only, SAR-only, and SAR-optical image pairs learning both inner- and intra-modality representations. Experimental results employing the BigEarthNet-MM dataset demonstrate the benefits of both, the ViT backbones and the proposed multimodal SSL algorithm DINO-MM.
PDF 4 pages, 1 figure; IGARSS 2022
论文截图
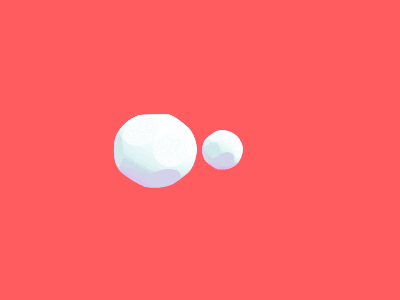
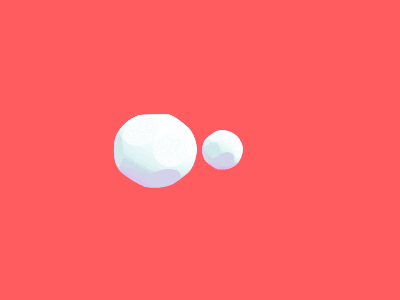
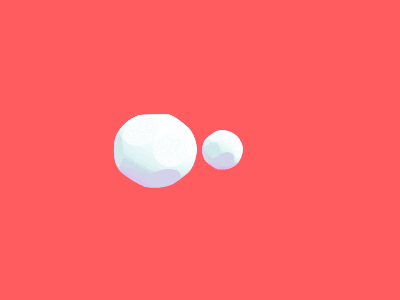
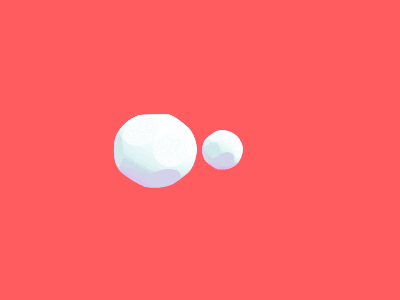
DiT: Self-supervised Pre-training for Document Image Transformer
Authors:Junlong Li, Yiheng Xu, Tengchao Lv, Lei Cui, Cha Zhang, Furu Wei
Image Transformer has recently achieved significant progress for natural image understanding, either using supervised (ViT, DeiT, etc.) or self-supervised (BEiT, MAE, etc.) pre-training techniques. In this paper, we propose DiT, a self-supervised pre-trained Document Image Transformer model using large-scale unlabeled text images for Document AI tasks, which is essential since no supervised counterparts ever exist due to the lack of human labeled document images. We leverage DiT as the backbone network in a variety of vision-based Document AI tasks, including document image classification, document layout analysis, table detection as well as text detection for OCR. Experiment results have illustrated that the self-supervised pre-trained DiT model achieves new state-of-the-art results on these downstream tasks, e.g. document image classification (91.11 $\rightarrow$ 92.69), document layout analysis (91.0 $\rightarrow$ 94.9), table detection (94.23 $\rightarrow$ 96.55) and text detection for OCR (93.07 $\rightarrow$ 94.29). The code and pre-trained models are publicly available at \url{https://aka.ms/msdit}.
PDF Work in Progress
论文截图
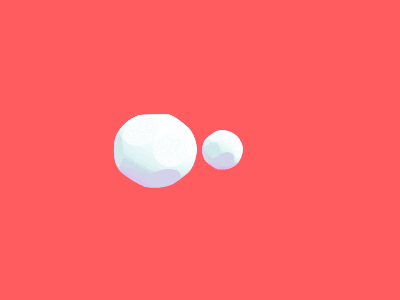
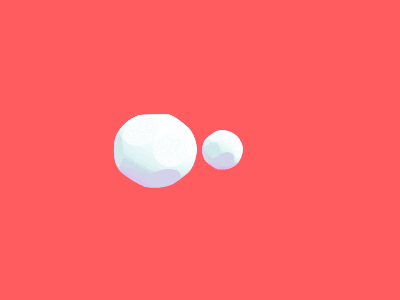
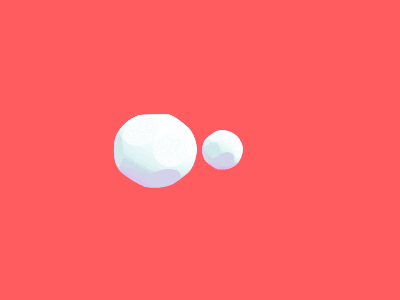
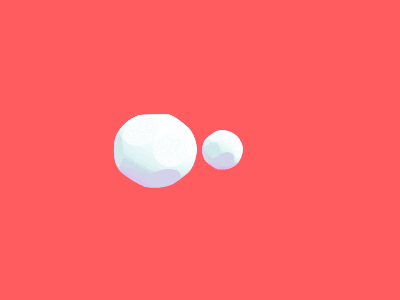
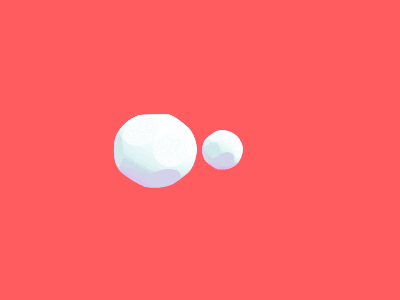
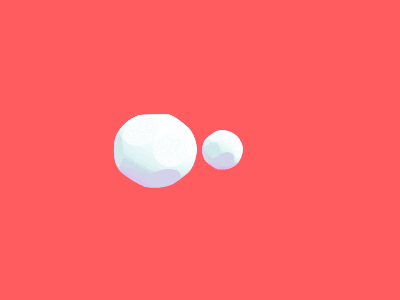
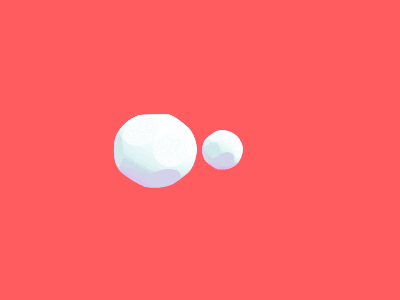