2022-04-08 更新
L2G: A Simple Local-to-Global Knowledge Transfer Framework for Weakly Supervised Semantic Segmentation
Authors:Peng-Tao Jiang, Yuqi Yang, Qibin Hou, Yunchao Wei
Mining precise class-aware attention maps, a.k.a, class activation maps, is essential for weakly supervised semantic segmentation. In this paper, we present L2G, a simple online local-to-global knowledge transfer framework for high-quality object attention mining. We observe that classification models can discover object regions with more details when replacing the input image with its local patches. Taking this into account, we first leverage a local classification network to extract attentions from multiple local patches randomly cropped from the input image. Then, we utilize a global network to learn complementary attention knowledge across multiple local attention maps online. Our framework conducts the global network to learn the captured rich object detail knowledge from a global view and thereby produces high-quality attention maps that can be directly used as pseudo annotations for semantic segmentation networks. Experiments show that our method attains 72.1% and 44.2% mIoU scores on the validation set of PASCAL VOC 2012 and MS COCO 2014, respectively, setting new state-of-the-art records. Code is available at https://github.com/PengtaoJiang/L2G.
PDF Accepted at CVPR 2022
论文截图
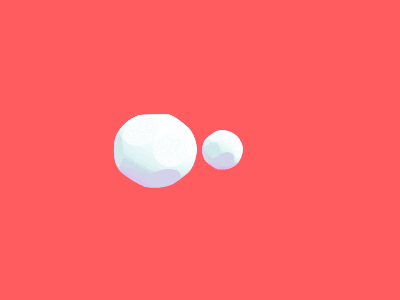
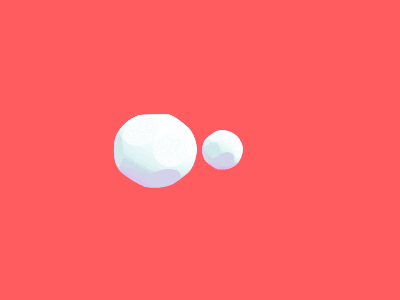
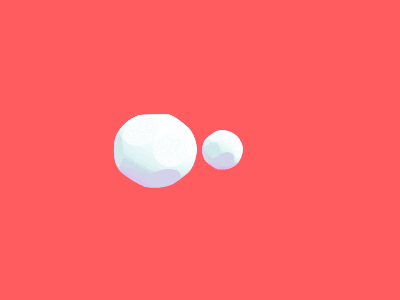
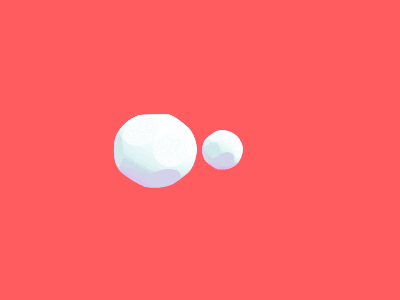
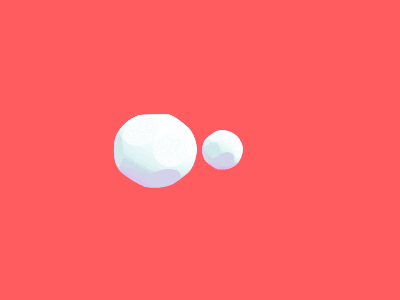
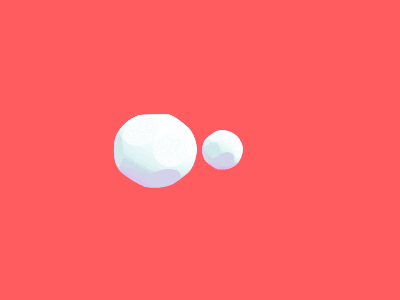
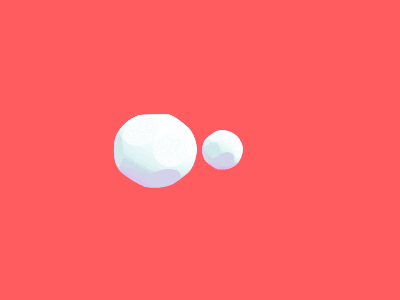
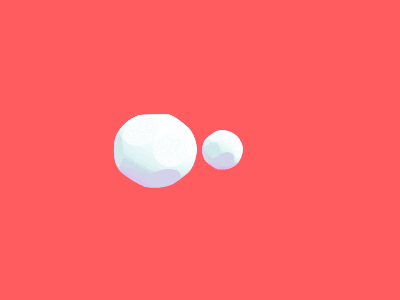
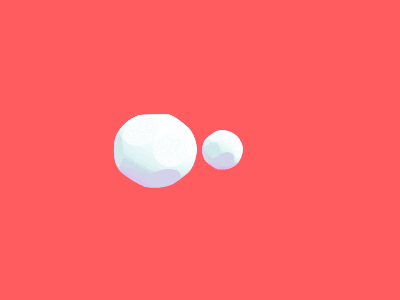
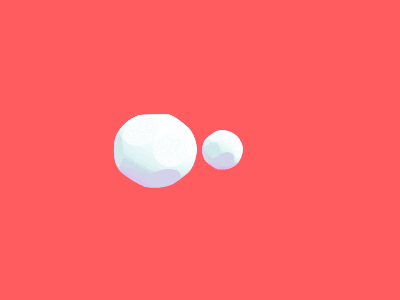
Instance Segmentation of Unlabeled Modalities via Cyclic Segmentation GAN
Authors:Leander Lauenburg, Zudi Lin, Ruihan Zhang, Márcia dos Santos, Siyu Huang, Ignacio Arganda-Carreras, Edward S. Boyden, Hanspeter Pfister, Donglai Wei
Instance segmentation for unlabeled imaging modalities is a challenging but essential task as collecting expert annotation can be expensive and time-consuming. Existing works segment a new modality by either deploying a pre-trained model optimized on diverse training data or conducting domain translation and image segmentation as two independent steps. In this work, we propose a novel Cyclic Segmentation Generative Adversarial Network (CySGAN) that conducts image translation and instance segmentation jointly using a unified framework. Besides the CycleGAN losses for image translation and supervised losses for the annotated source domain, we introduce additional self-supervised and segmentation-based adversarial objectives to improve the model performance by leveraging unlabeled target domain images. We benchmark our approach on the task of 3D neuronal nuclei segmentation with annotated electron microscopy (EM) images and unlabeled expansion microscopy (ExM) data. Our CySGAN outperforms both pretrained generalist models and the baselines that sequentially conduct image translation and segmentation. Our implementation and the newly collected, densely annotated ExM nuclei dataset, named NucExM, are available at https://connectomics-bazaar.github.io/proj/CySGAN/index.html.
PDF 13 pages with appendix
论文截图
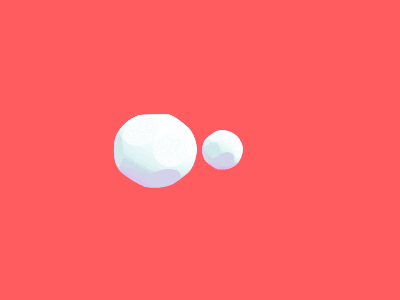
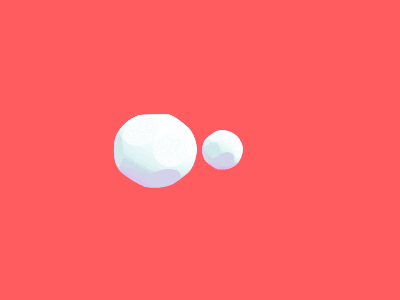
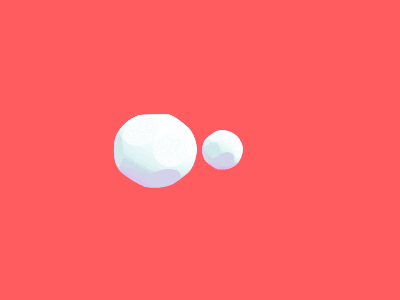
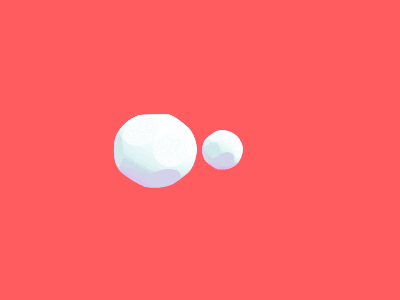
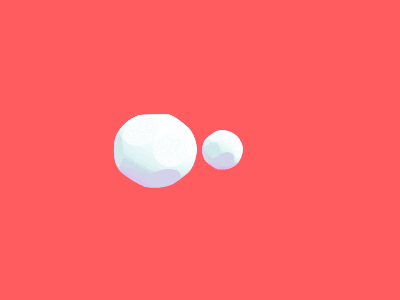
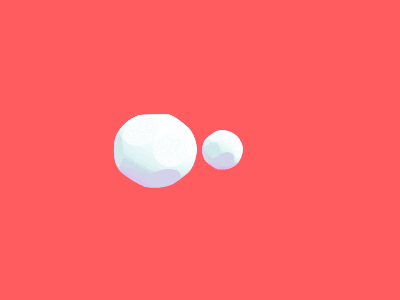
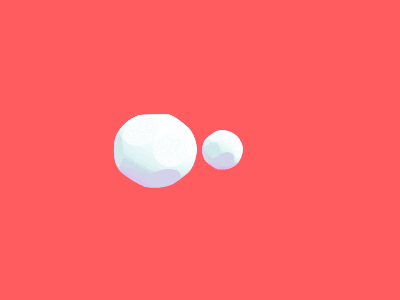
Slicing Aided Hyper Inference and Fine-tuning for Small Object Detection
Authors:Fatih Cagatay Akyon, Sinan Onur Altinuc, Alptekin Temizel
Detection of small objects and objects far away in the scene is a major challenge in surveillance applications. Such objects are represented by small number of pixels in the image and lack sufficient details, making them difficult to detect using conventional detectors. In this work, an open-source framework called Slicing Aided Hyper Inference (SAHI) is proposed that provides a generic slicing aided inference and fine-tuning pipeline for small object detection. The proposed technique is generic in the sense that it can be applied on top of any available object detector without any fine-tuning. Experimental evaluations, using object detection baselines on the Visdrone and xView aerial object detection datasets show that the proposed inference method can increase object detection AP by 6.8%, 5.1% and 5.3% for FCOS, VFNet and TOOD detectors, respectively. Moreover, the detection accuracy can be further increased with a slicing aided fine-tuning, resulting in a cumulative increase of 12.7%, 13.4% and 14.5% AP in the same order. Proposed technique has been integrated with Detectron2, MMDetection and YOLOv5 models and it is publicly available at https://github.com/obss/sahi.git .
PDF Submitted to ICIP 2022, 5 pages, 4 figures, 2 tables
论文截图
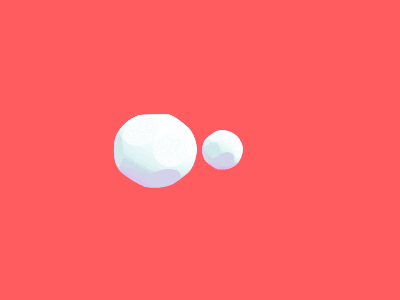
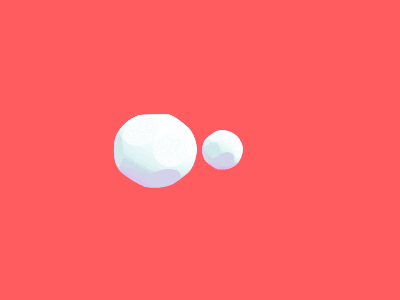
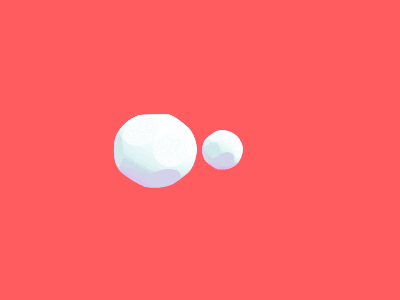
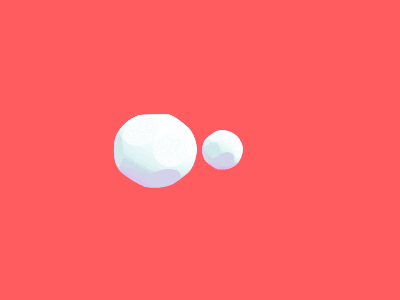
Coarse-to-Fine Feature Mining for Video Semantic Segmentation
Authors:Guolei Sun, Yun Liu, Henghui Ding, Thomas Probst, Luc Van Gool
The contextual information plays a core role in semantic segmentation. As for video semantic segmentation, the contexts include static contexts and motional contexts, corresponding to static content and moving content in a video clip, respectively. The static contexts are well exploited in image semantic segmentation by learning multi-scale and global/long-range features. The motional contexts are studied in previous video semantic segmentation. However, there is no research about how to simultaneously learn static and motional contexts which are highly correlated and complementary to each other. To address this problem, we propose a Coarse-to-Fine Feature Mining (CFFM) technique to learn a unified presentation of static contexts and motional contexts. This technique consists of two parts: coarse-to-fine feature assembling and cross-frame feature mining. The former operation prepares data for further processing, enabling the subsequent joint learning of static and motional contexts. The latter operation mines useful information/contexts from the sequential frames to enhance the video contexts of the features of the target frame. The enhanced features can be directly applied for the final prediction. Experimental results on popular benchmarks demonstrate that the proposed CFFM performs favorably against state-of-the-art methods for video semantic segmentation. Our implementation is available at https://github.com/GuoleiSun/VSS-CFFM
PDF Accepted to CVPR2022
论文截图
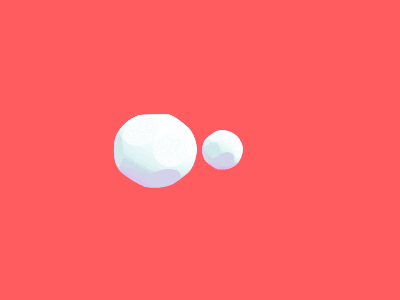
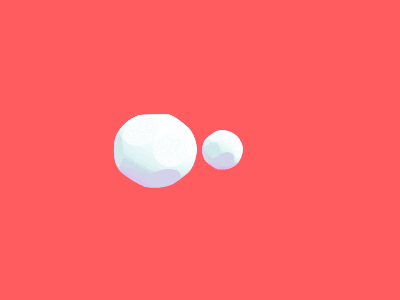
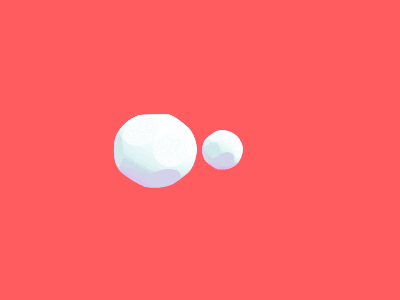
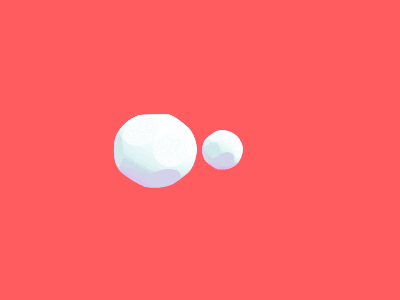
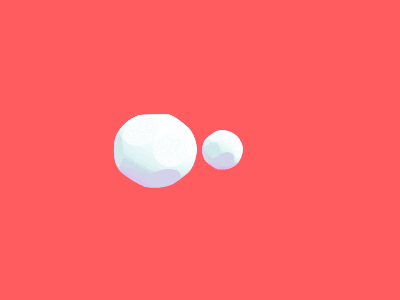