2022-03-30 更新
UnShadowNet: Illumination Critic Guided Contrastive Learning For Shadow Removal
Authors:Subhrajyoti Dasgupta, Arindam Das, Sudip Das, Andrei Bursuc, Ujjwal Bhattacharya, Senthil Yogamani
Shadows are frequently encountered natural phenomena that significantly hinder the performance of computer vision perception systems in practical settings, e.g., autonomous driving. A solution to this would be to eliminate shadow regions from the images before the processing of the perception system. Yet, training such a solution requires pairs of aligned shadowed and non-shadowed images which are difficult to obtain. We introduce a novel weakly supervised shadow removal framework UnShadowNet trained using contrastive learning. It comprises of a DeShadower network responsible for removal of the extracted shadow under the guidance of an Illumination network which is trained adversarially by the illumination critic and a Refinement network to further remove artifacts. We show that UnShadowNet can also be easily extended to a fully-supervised setup to exploit the ground-truth when available. UnShadowNet outperforms existing state-of-the-art approaches on three publicly available shadow datasets (ISTD, adjusted ISTD, SRD) in both the weakly and fully supervised setups.
PDF
论文截图
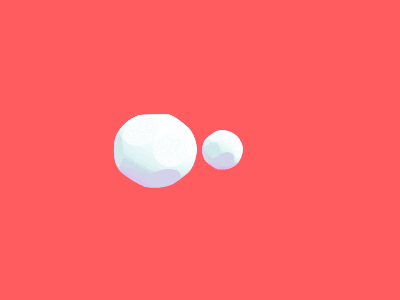
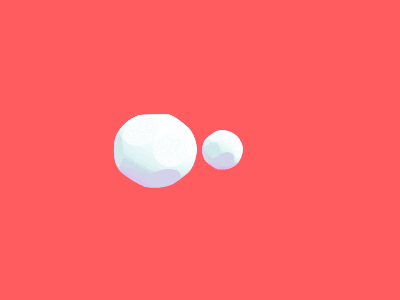
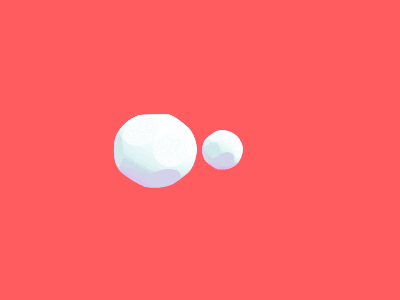
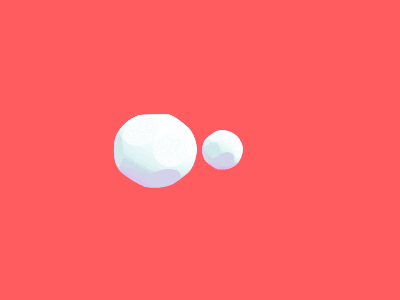
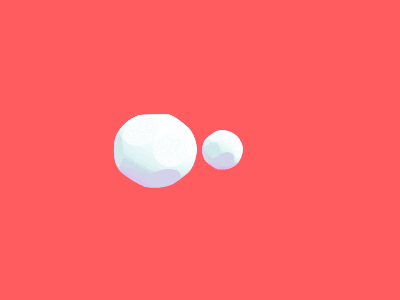
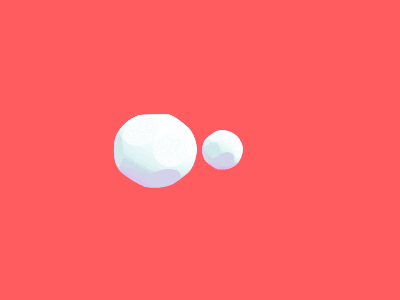
Robust Single Image Dehazing Based on Consistent and Contrast-Assisted Reconstruction
Authors:De Cheng, Yan Li, Dingwen Zhang, Nannan Wang, Xinbo Gao, Jiande Sun
Single image dehazing as a fundamental low-level vision task, is essential for the development of robust intelligent surveillance system. In this paper, we make an early effort to consider dehazing robustness under variational haze density, which is a realistic while under-studied problem in the research filed of singe image dehazing. To properly address this problem, we propose a novel density-variational learning framework to improve the robustness of the image dehzing model assisted by a variety of negative hazy images, to better deal with various complex hazy scenarios. Specifically, the dehazing network is optimized under the consistency-regularized framework with the proposed Contrast-Assisted Reconstruction Loss (CARL). The CARL can fully exploit the negative information to facilitate the traditional positive-orient dehazing objective function, by squeezing the dehazed image to its clean target from different directions. Meanwhile, the consistency regularization keeps consistent outputs given multi-level hazy images, thus improving the model robustness. Extensive experimental results on two synthetic and three real-world datasets demonstrate that our method significantly surpasses the state-of-the-art approaches.
PDF
论文截图
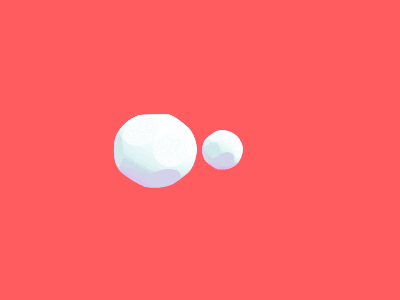
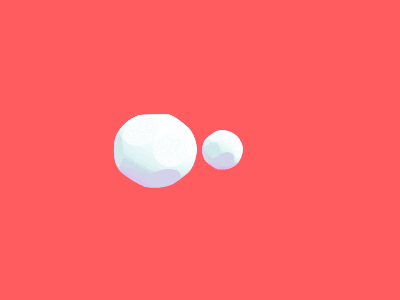
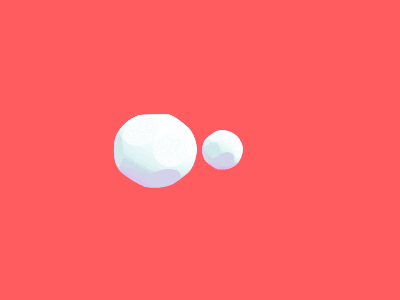
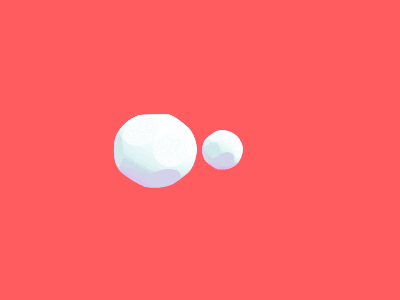
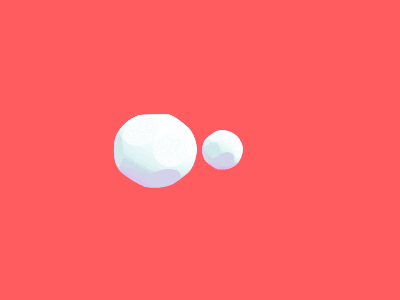
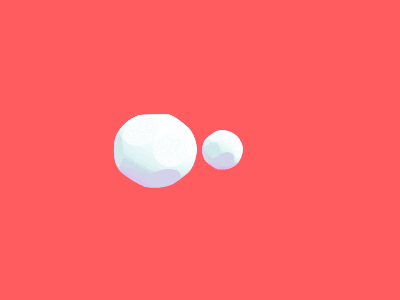
Min-Max Similarity: A Contrastive Learning Based Semi-Supervised Learning Network for Surgical Tools Segmentation
Authors:Ange Lou, Xing Yao, Ziteng Liu, Jack Noble
Segmentation of images is a popular topic in medical AI. This is mainly due to the difficulty to obtain a significant number of pixel-level annotated data to train a neural network. To address this issue, we proposed a semi-supervised segmentation network based on contrastive learning. In contrast to the previous state-of-the-art, we introduce a contrastive learning form of dual-view training by employing classifiers and projectors to build all-negative, and positive and negative feature pairs respectively to formulate the learning problem as solving min-max similarity problem. The all-negative pairs are used to supervise the networks learning from different views and make sure to capture general features, and the consistency of unlabeled predictions is measured by pixel-wise contrastive loss between positive and negative pairs. To quantitative and qualitative evaluate our proposed method, we test it on two public endoscopy surgical tool segmentation datasets and one cochlear implant surgery dataset which we manually annotate the cochlear implant in surgical videos. The segmentation performance (dice coefficients) indicates that our proposed method outperforms state-of-the-art semi-supervised and fully supervised segmentation algorithms consistently. The code is publicly available at: https://github.com/AngeLouCN/Min_Max_Similarity
PDF
论文截图
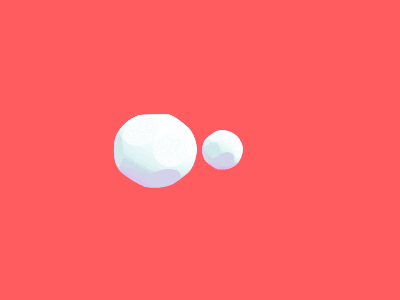
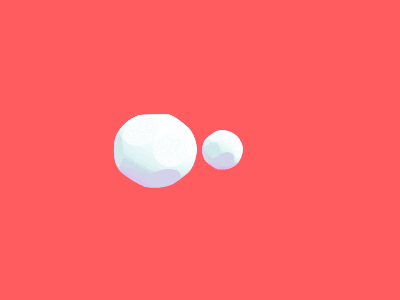
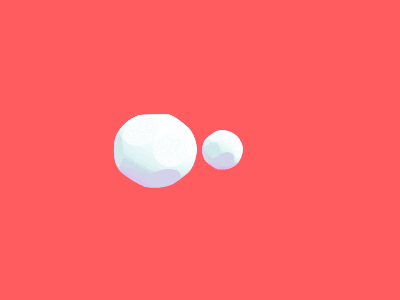
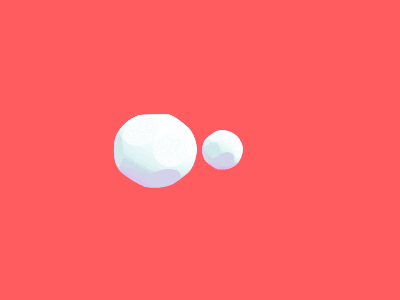
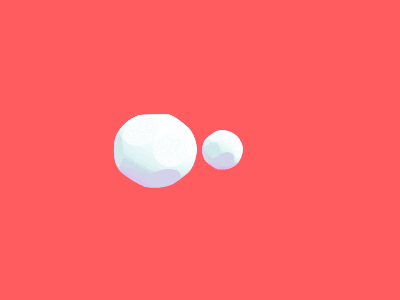
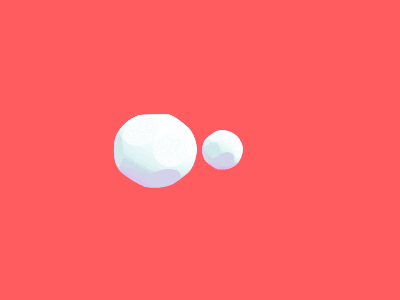