2022-03-24 更新
FIBER: Fill-in-the-Blanks as a Challenging Video Understanding Evaluation Framework
Authors:Santiago Castro, Ruoyao Wang, Pingxuan Huang, Ian Stewart, Oana Ignat, Nan Liu, Jonathan C. Stroud, Rada Mihalcea
We propose fill-in-the-blanks as a video understanding evaluation framework and introduce FIBER — a novel dataset consisting of 28,000 videos and descriptions in support of this evaluation framework. The fill-in-the-blanks setting tests a model’s understanding of a video by requiring it to predict a masked noun phrase in the caption of the video, given the video and the surrounding text. The FIBER benchmark does not share the weaknesses of the current state-of-the-art language-informed video understanding tasks, namely: (1) video question answering using multiple-choice questions, where models perform relatively well because they exploit linguistic biases in the task formulation, thus making our framework challenging for the current state-of-the-art systems to solve; and (2) video captioning, which relies on an open-ended evaluation framework that is often inaccurate because system answers may be perceived as incorrect if they differ in form from the ground truth. The FIBER dataset and our code are available at https://lit.eecs.umich.edu/fiber/.
PDF Accepted at ACL 2022 Main conference. Camera-ready version
论文截图
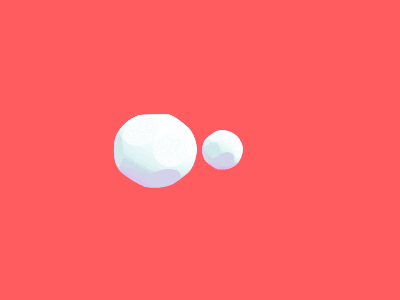
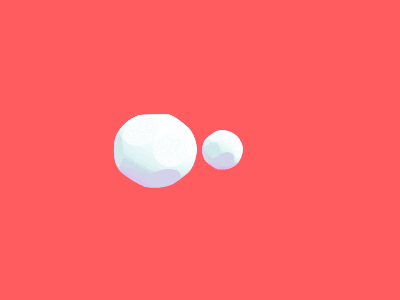
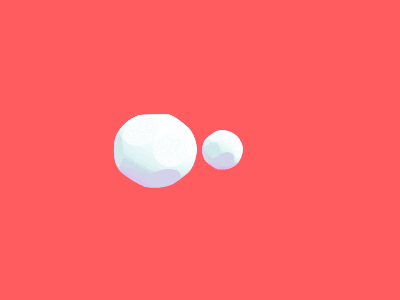
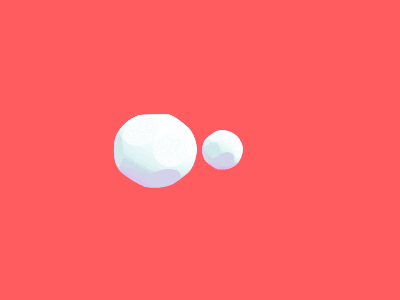
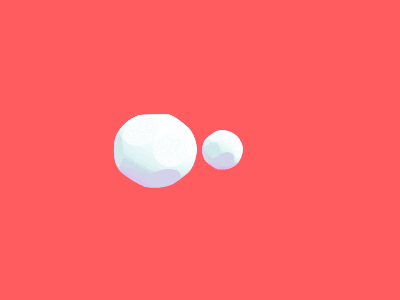
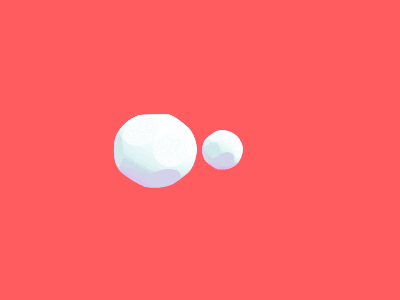
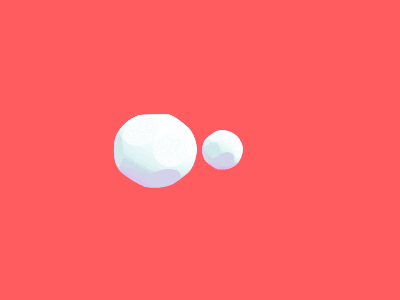
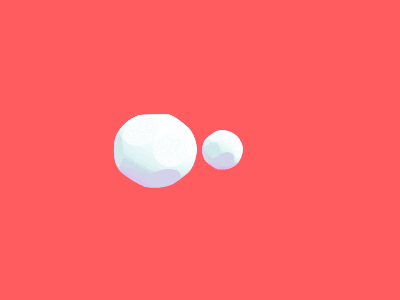