2022-03-24 更新
Regional Semantic Contrast and Aggregation for Weakly Supervised Semantic Segmentation
Authors:Tianfei Zhou, Meijie Zhang, Fang Zhao, Jianwu Li
Learning semantic segmentation from weakly-labeled (e.g., image tags only) data is challenging since it is hard to infer dense object regions from sparse semantic tags. Despite being broadly studied, most current efforts directly learn from limited semantic annotations carried by individual image or image pairs, and struggle to obtain integral localization maps. Our work alleviates this from a novel perspective, by exploring rich semantic contexts synergistically among abundant weakly-labeled training data for network learning and inference. In particular, we propose regional semantic contrast and aggregation (RCA) . RCA is equipped with a regional memory bank to store massive, diverse object patterns appearing in training data, which acts as strong support for exploration of dataset-level semantic structure. Particularly, we propose i) semantic contrast to drive network learning by contrasting massive categorical object regions, leading to a more holistic object pattern understanding, and ii) semantic aggregation to gather diverse relational contexts in the memory to enrich semantic representations. In this manner, RCA earns a strong capability of fine-grained semantic understanding, and eventually establishes new state-of-the-art results on two popular benchmarks, i.e., PASCAL VOC 2012 and COCO 2014.
PDF Accepted to CVPR 2022. Code: https://github.com/maeve07/RCA.git
论文截图
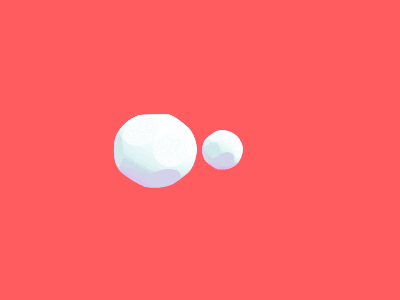
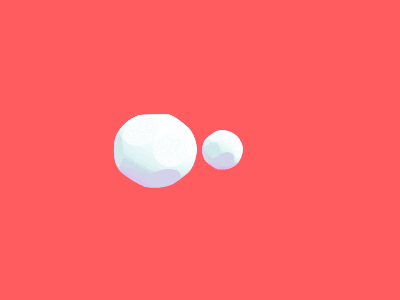
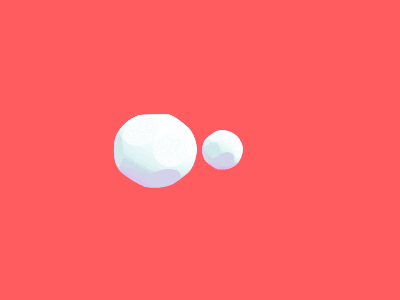
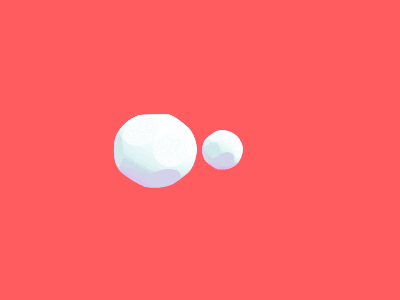
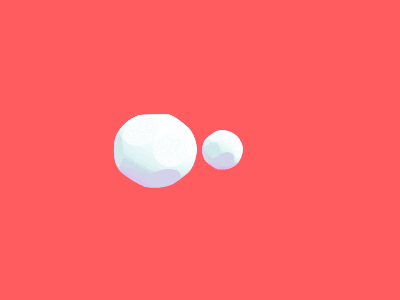
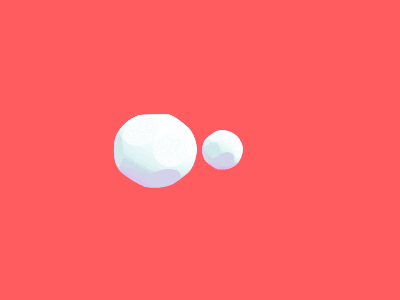
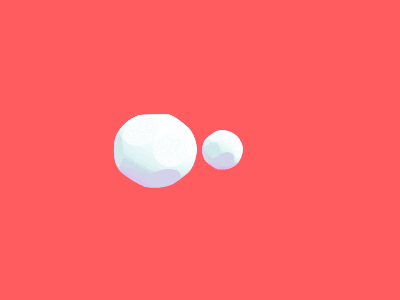
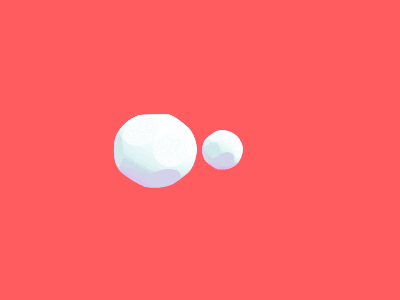
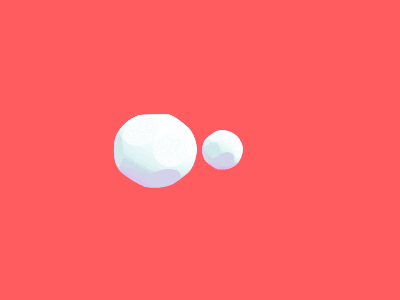
On the Arbitrary-Oriented Object Detection: Classification based Approaches Revisited
Authors:Xue Yang, Junchi Yan
Arbitrary-oriented object detection has been a building block for rotation sensitive tasks. We first show that the boundary problem suffered in existing dominant regression-based rotation detectors, is caused by angular periodicity or corner ordering, according to the parameterization protocol. We also show that the root cause is that the ideal predictions can be out of the defined range. Accordingly, we transform the angular prediction task from a regression problem to a classification one. For the resulting circularly distributed angle classification problem, we first devise a Circular Smooth Label technique to handle the periodicity of angle and increase the error tolerance to adjacent angles. To reduce the excessive model parameters by Circular Smooth Label, we further design a Densely Coded Labels, which greatly reduces the length of the encoding. Finally, we further develop an object heading detection module, which can be useful when the exact heading orientation information is needed e.g. for ship and plane heading detection. We release our OHD-SJTU dataset and OHDet detector for heading detection. Extensive experimental results on three large-scale public datasets for aerial images i.e. DOTA, HRSC2016, OHD-SJTU, and face dataset FDDB, as well as scene text dataset ICDAR2015 and MLT, show the effectiveness of our approach.
PDF 19 pages, 16 figures, 18 tables, journal version of CSL (ECCV2020) and DCL (CVPR2021), accepted by IJCV2022
论文截图
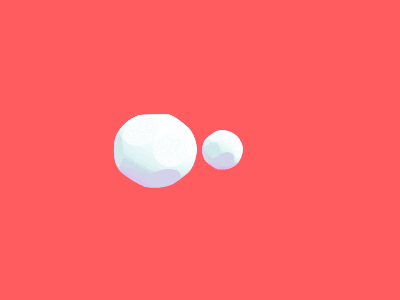
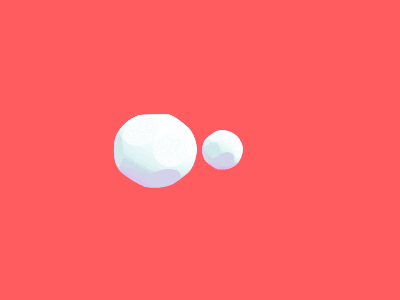
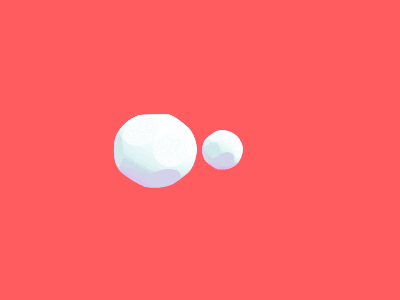
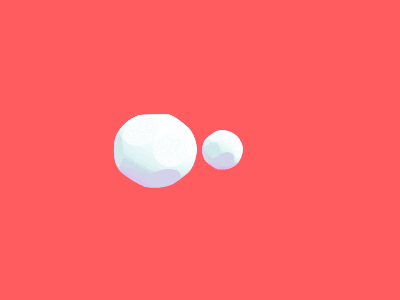
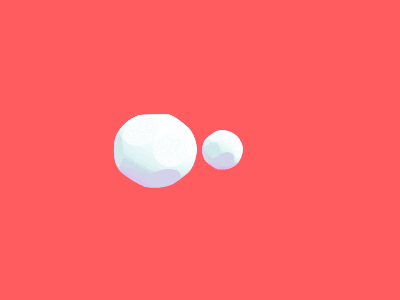
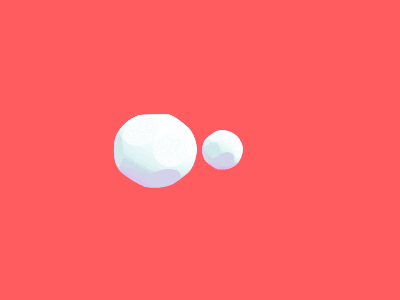
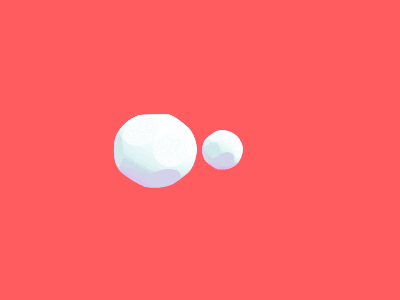
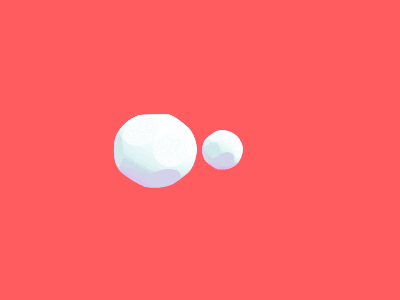
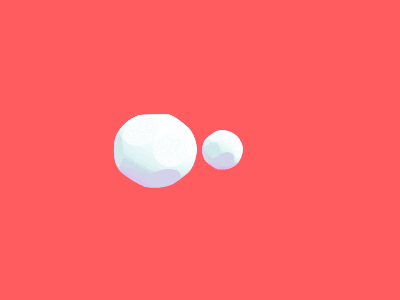
Unsupervised Salient Object Detection with Spectral Cluster Voting
Authors:Gyungin Shin, Samuel Albanie, Weidi Xie
In this paper, we tackle the challenging task of unsupervised salient object detection (SOD) by leveraging spectral clustering on self-supervised features. We make the following contributions: (i) We revisit spectral clustering and demonstrate its potential to group the pixels of salient objects; (ii) Given mask proposals from multiple applications of spectral clustering on image features computed from various self-supervised models, e.g., MoCov2, SwAV, DINO, we propose a simple but effective winner-takes-all voting mechanism for selecting the salient masks, leveraging object priors based on framing and distinctiveness; (iii) Using the selected object segmentation as pseudo groundtruth masks, we train a salient object detector, dubbed SelfMask, which outperforms prior approaches on three unsupervised SOD benchmarks. Code is publicly available at https://github.com/NoelShin/selfmask.
PDF 14 pages, 5 figures
论文截图
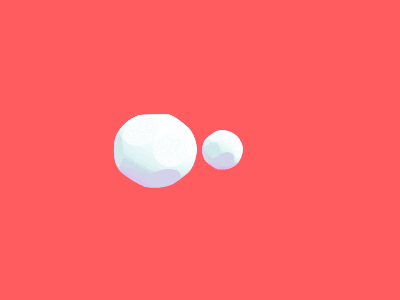
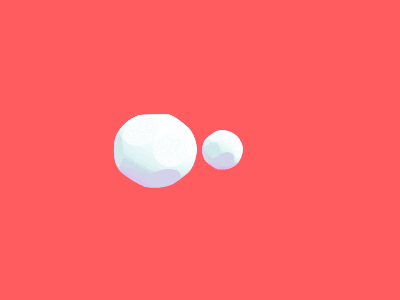
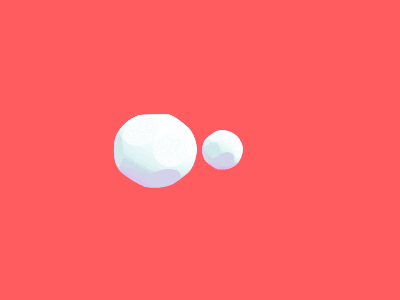
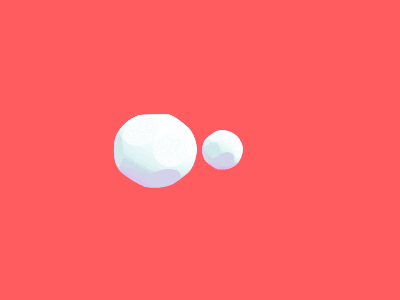
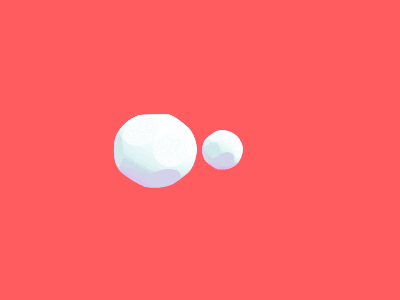
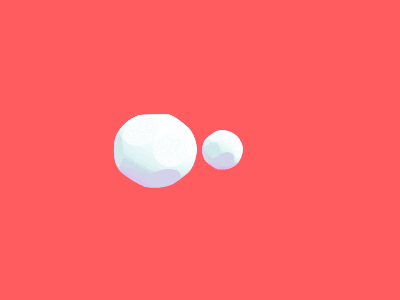
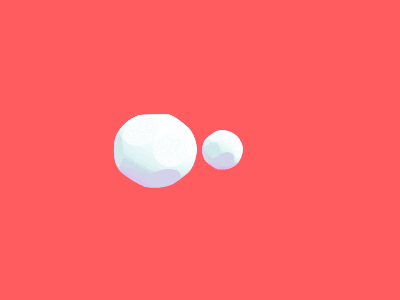
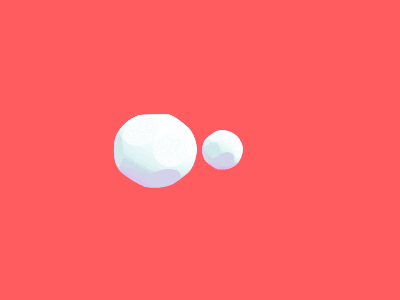
StructToken : Rethinking Semantic Segmentation with Structural Prior
Authors:Fangjian Lin, Zhanhao Liang, Junjun He, Miao Zheng, Shengwei Tian, Kai Chen
In this paper, we present structure token (StructToken), a new paradigm for semantic segmentation. From a perspective on semantic segmentation as per-pixel classification, the previous deep learning-based methods learn the per-pixel representation first through an encoder and a decoder head and then classify each pixel representation to a specific category to obtain the semantic masks. Differently, we propose a structure-aware algorithm that takes structural information as prior to predict semantic masks directly without per-pixel classification. Specifically, given an input image, the learnable structure token interacts with the image representations to reason the final semantic masks. Three interaction approaches are explored and the results not only outperform the state-of-the-art methods but also contain more structural information. Experiments are conducted on three widely used datasets including ADE20k, Cityscapes, and COCO-Stuff 10K. We hope that structure token could serve as an alternative for semantic segmentation and inspire future research.
PDF 22 pages, 10 figures
论文截图
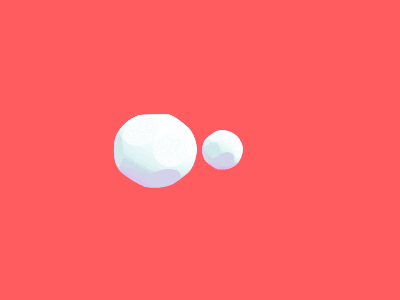
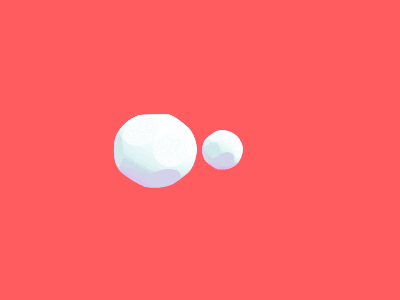
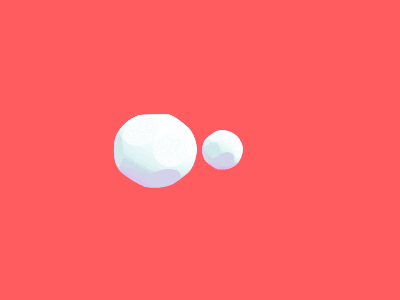
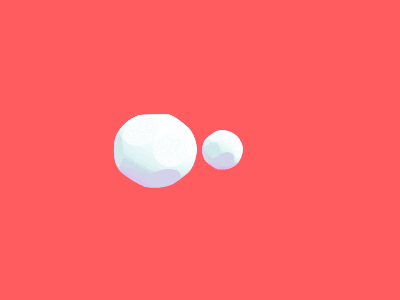
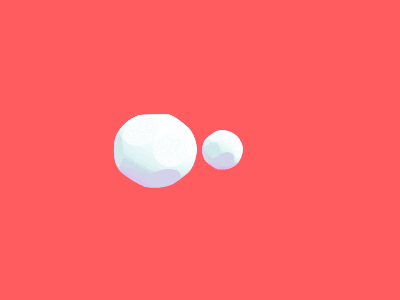