2022-03-09 更新
Unsupervised Domain Adaptation with Contrastive Learning for OCT Segmentation
Authors:Alvaro Gomariz, Huanxiang Lu, Yun Yvonna Li, Thomas Albrecht, Andreas Maunz, Fethallah Benmansour, Alessandra M. Valcarcel, Jennifer Luu, Daniela Ferrara, Orcun Goksel
Accurate segmentation of retinal fluids in 3D Optical Coherence Tomography images is key for diagnosis and personalized treatment of eye diseases. While deep learning has been successful at this task, trained supervised models often fail for images that do not resemble labeled examples, e.g. for images acquired using different devices. We hereby propose a novel semi-supervised learning framework for segmentation of volumetric images from new unlabeled domains. We jointly use supervised and contrastive learning, also introducing a contrastive pairing scheme that leverages similarity between nearby slices in 3D. In addition, we propose channel-wise aggregation as an alternative to conventional spatial-pooling aggregation for contrastive feature map projection. We evaluate our methods for domain adaptation from a (labeled) source domain to an (unlabeled) target domain, each containing images acquired with different acquisition devices. In the target domain, our method achieves a Dice coefficient 13.8% higher than SimCLR (a state-of-the-art contrastive framework), and leads to results comparable to an upper bound with supervised training in that domain. In the source domain, our model also improves the results by 5.4% Dice, by successfully leveraging information from many unlabeled images.
PDF Main: 10 pages, 4 figures, 1 table. Supplementary: 2 pages, 1 figure, 4 tables
论文截图
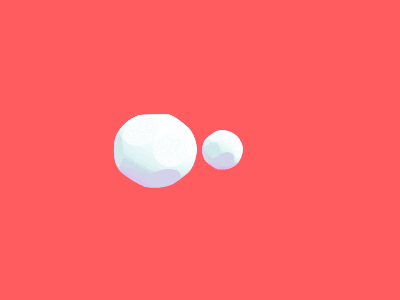
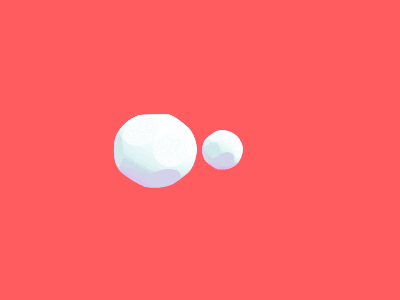
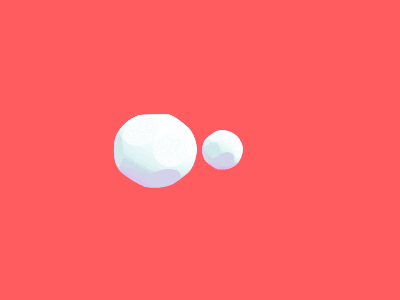
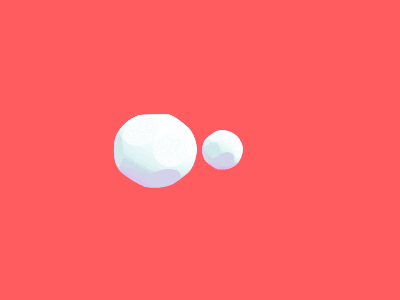
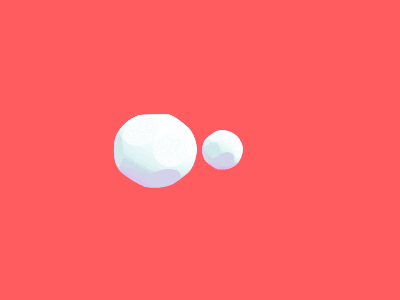
Seeing BDD100K in dark: Single-Stage Night-time Object Detection via Continual Fourier Contrastive Learning
Authors:Ujjal Kr Dutta
In this paper, we study the lesser explored avenue of object detection at night-time. An object detector trained on abundant labeled daytime images often fails to perform well on night images, due to domain gap. As collecting more labeled data from night-time is expensive, unpaired generative image translation techniques seek to synthesize night-time images. However, unrealistic artifacts often arise on the synthetic images. Illuminating night-time inference images also does not work well in practice, as shown in our paper. To address these issues, we suggest a novel technique for enhancing the object detector via Contrastive Learning, which tries to group together embeddings of similar images. To provide anchor-positive image pairs for Contrastive Learning, we leverage Fourier Transformation, which is naturally good at preserving the semantics of an image. For practical benefits in real-time applications, we choose the recently proposed YOLOF single-stage detector, which provides a simple and clean encoder-decoder segregation of the detector network. However, merely trying to teach the encoder to perform well on the auxiliary Contrastive Learning task may lead to catastrophic forgetting of the knowledge essential for object detection. Hence, we train the encoder in a Continual Learning fashion. Our novel method by an elegant training framework achieves state-of-the-art performance on the large scale BDD100K dataset, in an uniform setting, chosen, to the best of our knowledge, for the first time.
PDF
论文截图
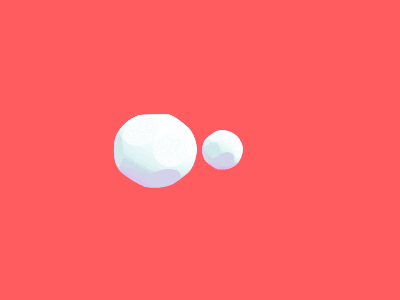
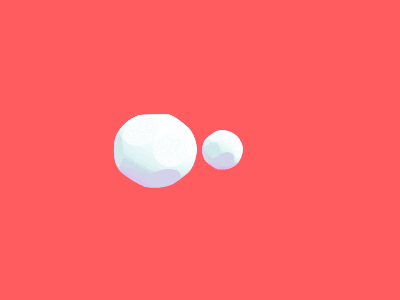
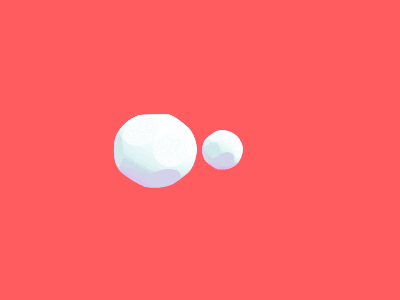
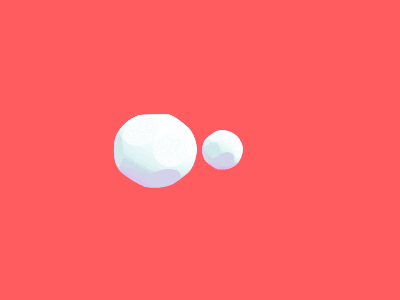
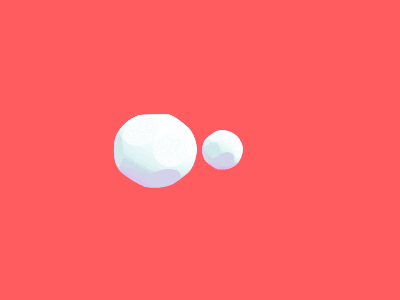
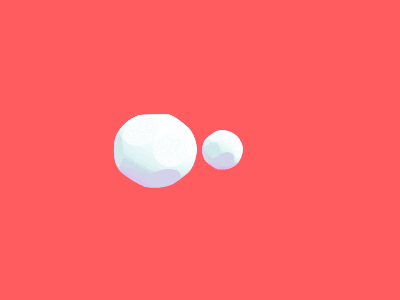