2022-03-03 更新
CrossPoint: Self-Supervised Cross-Modal Contrastive Learning for 3D Point Cloud Understanding
Authors:Mohamed Afham, Isuru Dissanayake, Dinithi Dissanayake, Amaya Dharmasiri, Kanchana Thilakarathna, Ranga Rodrigo
Manual annotation of large-scale point cloud dataset for varying tasks such as 3D object classification, segmentation and detection is often laborious owing to the irregular structure of point clouds. Self-supervised learning, which operates without any human labeling, is a promising approach to address this issue. We observe in the real world that humans are capable of mapping the visual concepts learnt from 2D images to understand the 3D world. Encouraged by this insight, we propose CrossPoint, a simple cross-modal contrastive learning approach to learn transferable 3D point cloud representations. It enables a 3D-2D correspondence of objects by maximizing agreement between point clouds and the corresponding rendered 2D image in the invariant space, while encouraging invariance to transformations in the point cloud modality. Our joint training objective combines the feature correspondences within and across modalities, thus ensembles a rich learning signal from both 3D point cloud and 2D image modalities in a self-supervised fashion. Experimental results show that our approach outperforms the previous unsupervised learning methods on a diverse range of downstream tasks including 3D object classification and segmentation. Further, the ablation studies validate the potency of our approach for a better point cloud understanding. Code and pretrained models are available at http://github.com/MohamedAfham/CrossPoint.
PDF Accepted at CVPR 2022
论文截图
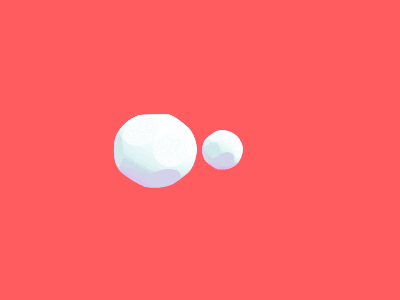
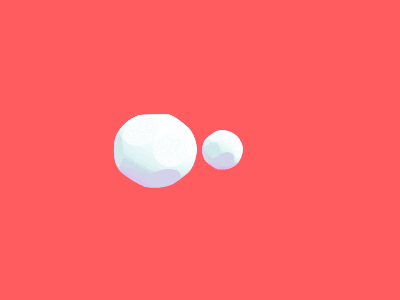
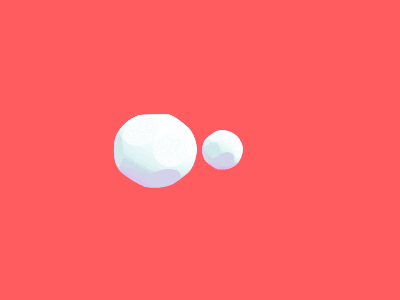
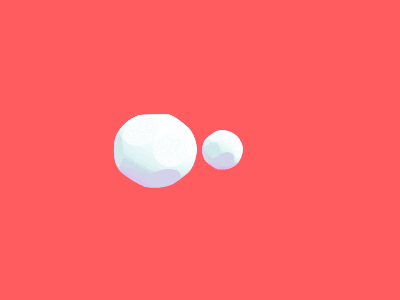
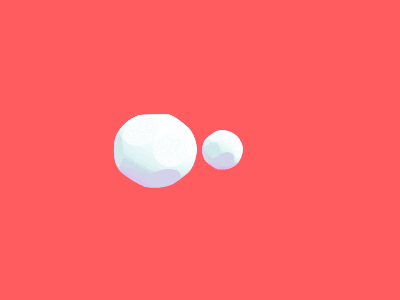
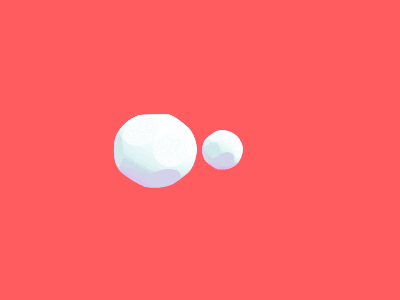
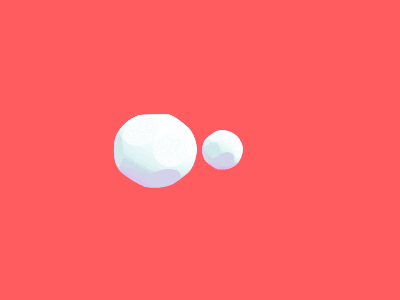
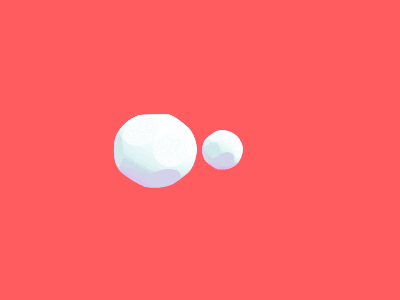
Vision-Language Pre-Training with Triple Contrastive Learning
Authors:Jinyu Yang, Jiali Duan, Son Tran, Yi Xu, Sampath Chanda, Liqun Chen, Belinda Zeng, Trishul Chilimbi, Junzhou Huang
Vision-language representation learning largely benefits from image-text alignment through contrastive losses (e.g., InfoNCE loss). The success of this alignment strategy is attributed to its capability in maximizing the mutual information (MI) between an image and its matched text. However, simply performing cross-modal alignment (CMA) ignores data potential within each modality, which may result in degraded representations. For instance, although CMA-based models are able to map image-text pairs close together in the embedding space, they fail to ensure that similar inputs from the same modality stay close by. This problem can get even worse when the pre-training data is noisy. In this paper, we propose triple contrastive learning (TCL) for vision-language pre-training by leveraging both cross-modal and intra-modal self-supervision. Besides CMA, TCL introduces an intra-modal contrastive objective to provide complementary benefits in representation learning. To take advantage of localized and structural information from image and text input, TCL further maximizes the average MI between local regions of image/text and their global summary. To the best of our knowledge, ours is the first work that takes into account local structure information for multi-modality representation learning. Experimental evaluations show that our approach is competitive and achieve the new state of the art on various common down-stream vision-language tasks such as image-text retrieval and visual question answering.
PDF code: https://github.com/uta-smile/TCL; accepted by CVPR 2022
论文截图
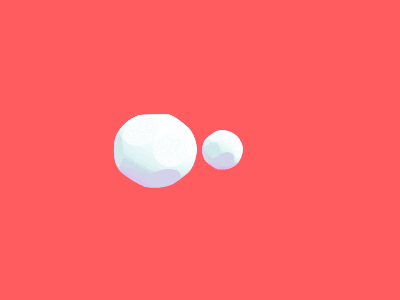
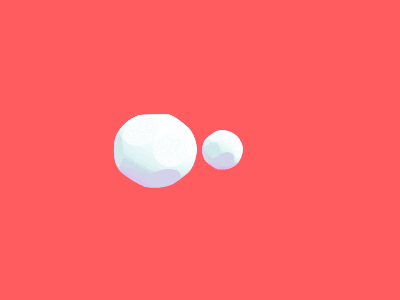
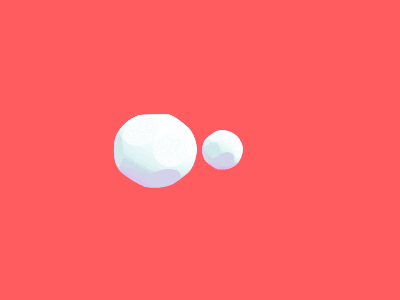
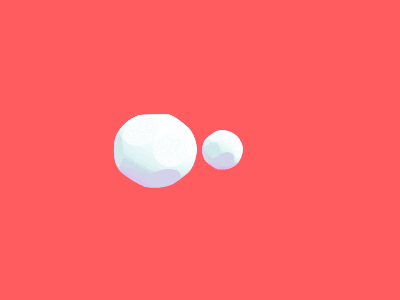
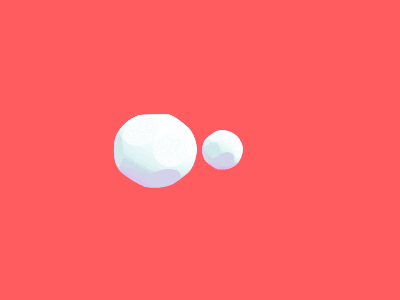
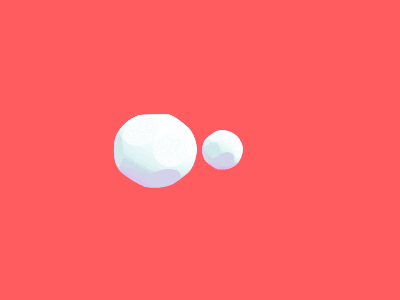
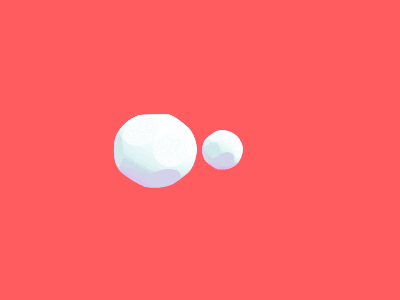
ACTIVE:Augmentation-Free Graph Contrastive Learning for Partial Multi-View Clustering
Authors:Yiming Wang, Dongxia Chang, Zhiqiang Fu, Jie Wen, Yao Zhao
In this paper, we propose an augmentation-free graph contrastive learning framework, namely ACTIVE, to solve the problem of partial multi-view clustering. Notably, we suppose that the representations of similar samples (i.e., belonging to the same cluster) and their multiply views features should be similar. This is distinct from the general unsupervised contrastive learning that assumes an image and its augmentations share a similar representation. Specifically, relation graphs are constructed using the nearest neighbours to identify existing similar samples, then the constructed inter-instance relation graphs are transferred to the missing views to build graphs on the corresponding missing data. Subsequently, two main components, within-view graph contrastive learning (WGC) and cross-view graph consistency learning (CGC), are devised to maximize the mutual information of different views within a cluster. The proposed approach elevates instance-level contrastive learning and missing data inference to the cluster-level, effectively mitigating the impact of individual missing data on clustering. Experiments on several challenging datasets demonstrate the superiority of our proposed methods.
PDF
论文截图
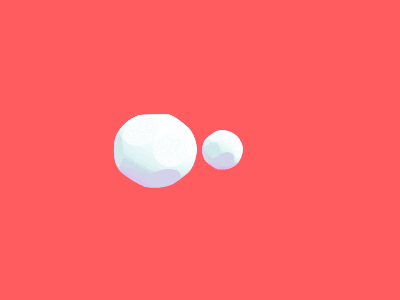
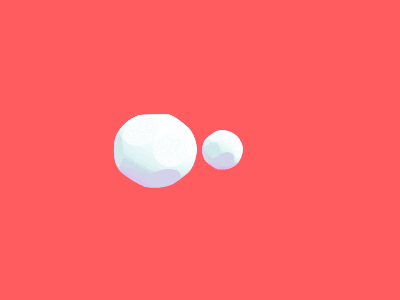
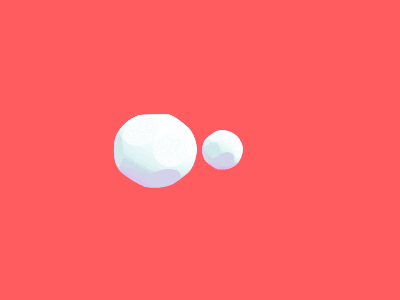
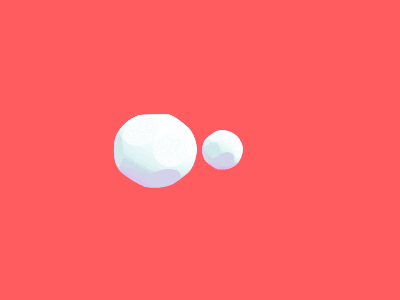
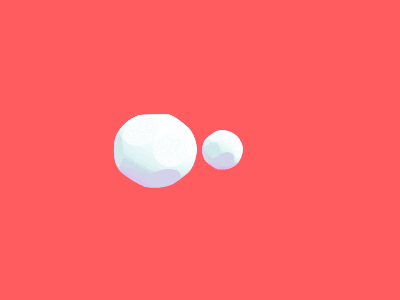
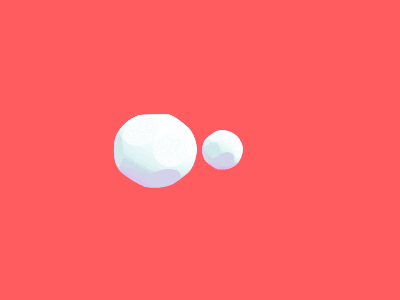
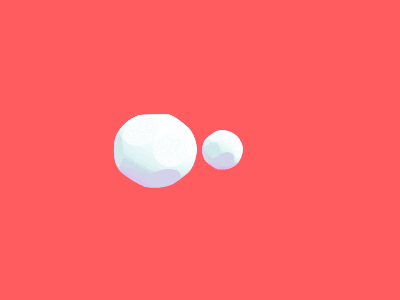