2022-03-01 更新
DCL: Differential Contrastive Learning for Geometry-Aware Depth Synthesis
Authors:Yuefan Shen, Yanchao Yang, Youyi Zheng, C. Karen Liu, Leonidas Guibas
We describe a method for unpaired realistic depth synthesis that learns diverse variations from the real-world depth scans and ensures geometric consistency between the synthetic and synthesized depth. The synthesized realistic depth can then be used to train task-specific networks facilitating label transfer from the synthetic domain. Unlike existing image synthesis pipelines, where geometries are mostly ignored, we treat geometries carried by the depth scans based on their own existence. We propose differential contrastive learning that explicitly enforces the underlying geometric properties to be invariant regarding the real variations been learned. The resulting depth synthesis method is task-agnostic, and we demonstrate the effectiveness of the proposed synthesis method by extensive evaluations on real-world geometric reasoning tasks. The networks trained with the depth synthesized by our method consistently achieve better performance across a wide range of tasks than state of the art, and can even surpass the networks supervised with full real-world annotations when slightly fine-tuned, showing good transferability.
PDF Accepted by International Conference on Robotics and Automation (ICRA) 2022 and RA-L 2022
论文截图
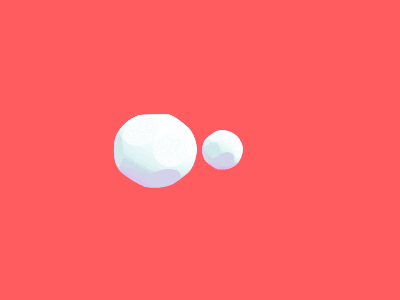
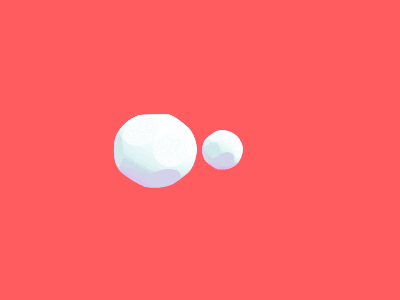
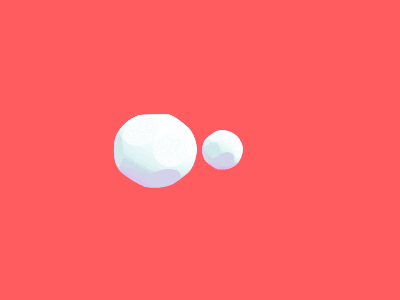
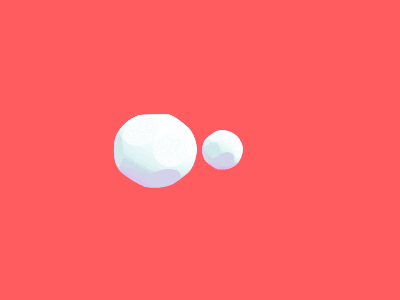
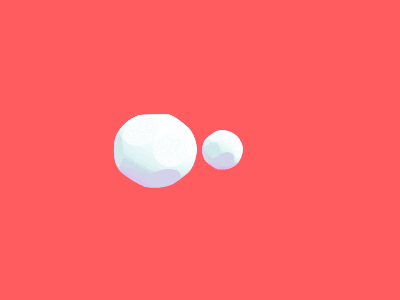
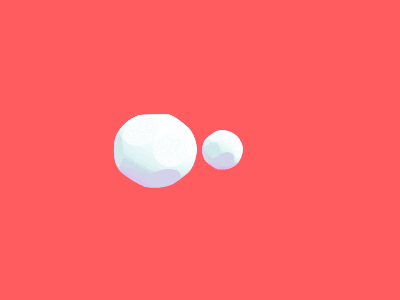
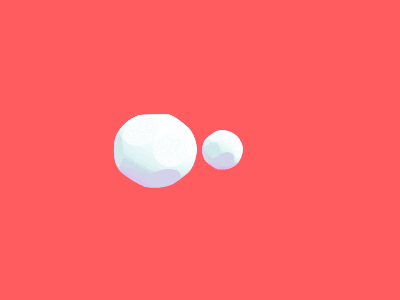
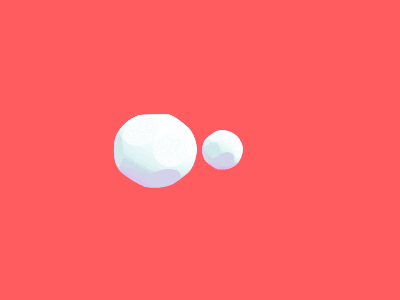
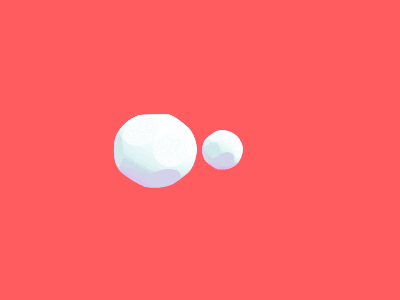
Incremental False Negative Detection for Contrastive Learning
Authors:Tsai-Shien Chen, Wei-Chih Hung, Hung-Yu Tseng, Shao-Yi Chien, Ming-Hsuan Yang
Self-supervised learning has recently shown great potential in vision tasks through contrastive learning, which aims to discriminate each image, or instance, in the dataset. However, such instance-level learning ignores the semantic relationship among instances and sometimes undesirably repels the anchor from the semantically similar samples, termed as “false negatives”. In this work, we show that the unfavorable effect from false negatives is more significant for the large-scale datasets with more semantic concepts. To address the issue, we propose a novel self-supervised contrastive learning framework that incrementally detects and explicitly removes the false negative samples. Specifically, following the training process, our method dynamically detects increasing high-quality false negatives considering that the encoder gradually improves and the embedding space becomes more semantically structural. Next, we discuss two strategies to explicitly remove the detected false negatives during contrastive learning. Extensive experiments show that our framework outperforms other self-supervised contrastive learning methods on multiple benchmarks in a limited resource setup.
PDF
论文截图
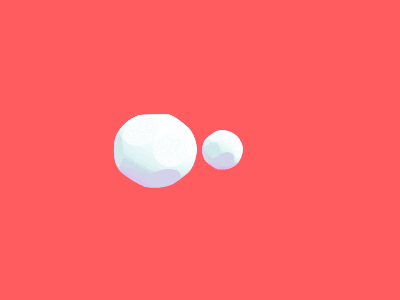
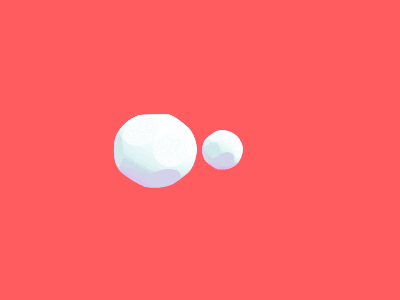
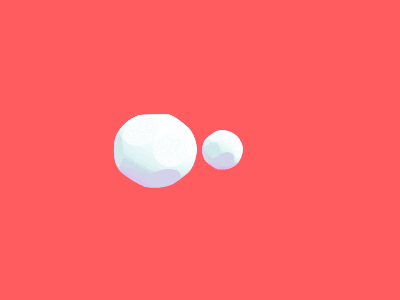
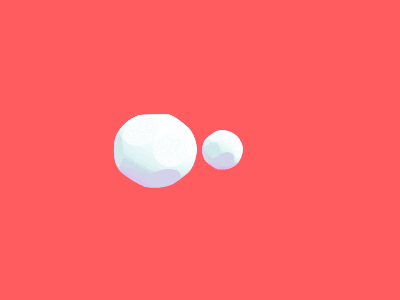
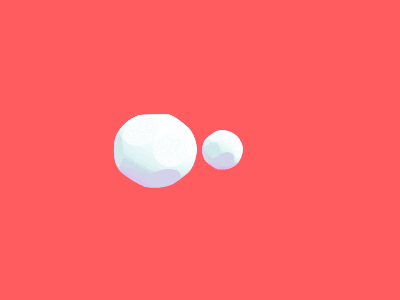
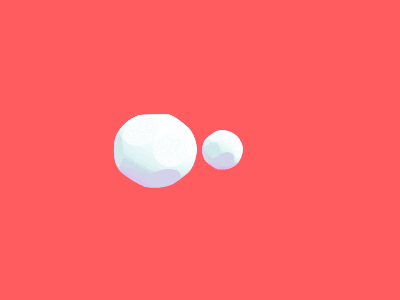
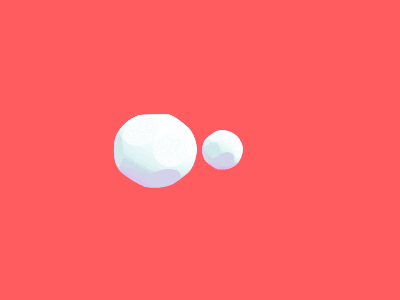