2022-02-24 更新
Learning with Free Object Segments for Long-Tailed Instance Segmentation
Authors:Cheng Zhang, Tai-Yu Pan, Tianle Chen, Jike Zhong, Wenjin Fu, Wei-Lun Chao
One fundamental challenge in building an instance segmentation model for a large number of classes in complex scenes is the lack of training examples, especially for rare objects. In this paper, we explore the possibility to increase the training examples without laborious data collection and annotation. We find that an abundance of instance segments can potentially be obtained freely from object-centric im-ages, according to two insights: (i) an object-centric image usually contains one salient object in a simple background; (ii) objects from the same class often share similar appearances or similar contrasts to the background. Motivated by these insights, we propose a simple and scalable framework FreeSeg for extracting and leveraging these “free” object foreground segments to facilitate model training in long-tailed instance segmentation. Concretely, we employ off-the-shelf object foreground extraction techniques (e.g., image co-segmentation) to generate instance mask candidates, followed by segments refinement and ranking. The resulting high-quality object segments can be used to augment the existing long-tailed dataset, e.g., by copying and pasting the segments onto the original training images. On the LVIS benchmark, we show that FreeSeg yields substantial improvements on top of strong baselines and achieves state-of-the-art accuracy for segmenting rare object categories.
PDF
论文截图
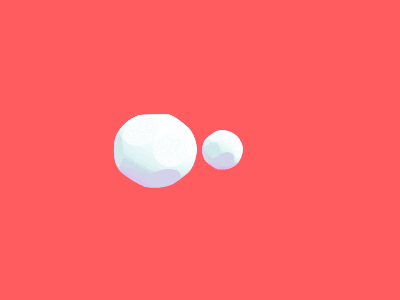
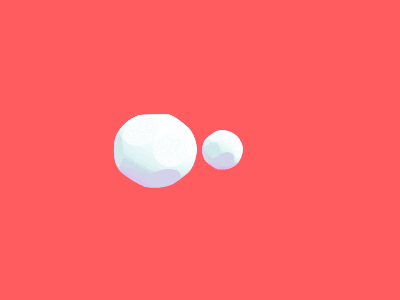
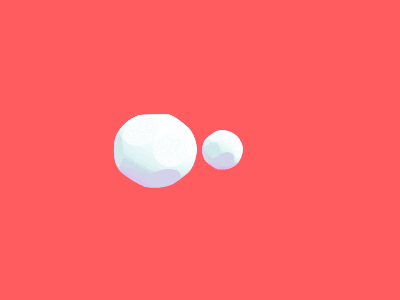
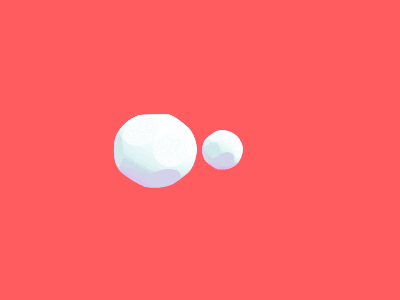
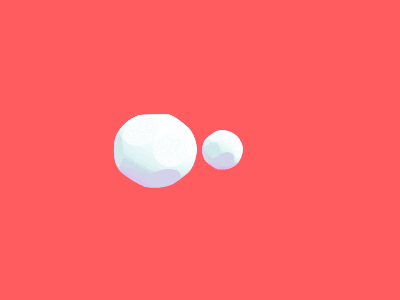
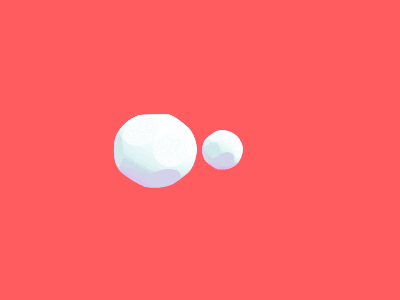
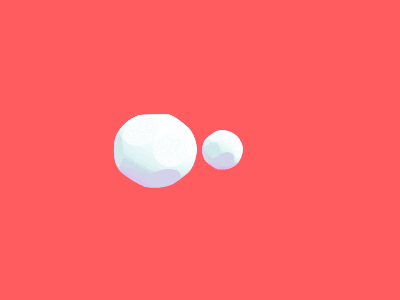
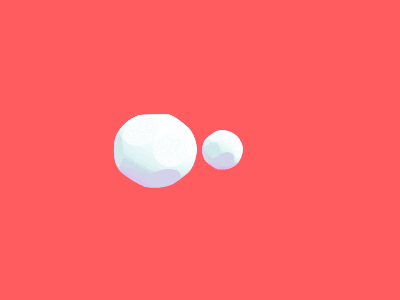
An End-to-End Cascaded Image Deraining and Object Detection Neural Network
Authors:Kaige Wang, Tianming Wang, Jianchuang Qu, Huatao Jiang, Qing Li, Lin Chang
While the deep learning-based image deraining methods have made great progress in recent years, there are two major shortcomings in their application in real-world situations. Firstly, the gap between the low-level vision task represented by rain removal and the high-level vision task represented by object detection is significant, and the low-level vision task can hardly contribute to the high-level vision task. Secondly, the quality of the deraining dataset needs to be improved. In fact, the rain lines in many baselines have a large gap with the real rain lines, and the resolution of the deraining dataset images is generally not ideally. Meanwhile, there are few common datasets for both the low-level vision task and the high-level vision task. In this paper, we explore the combination of the low-level vision task with the high-level vision task. Specifically, we propose an end-to-end object detection network for reducing the impact of rainfall, which consists of two cascaded networks, an improved image deraining network and an object detection network, respectively. We also design the components of the loss function to accommodate the characteristics of the different sub-networks. We then propose a dataset based on the KITTI dataset for rainfall removal and object detection, on which our network surpasses the state-of-the-art with a significant improvement in metrics. Besides, our proposed network is measured on driving videos collected by self-driving vehicles and shows positive results for rain removal and object detection.
PDF
论文截图
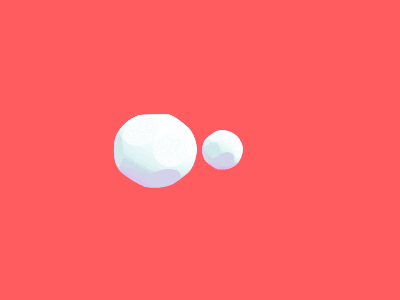
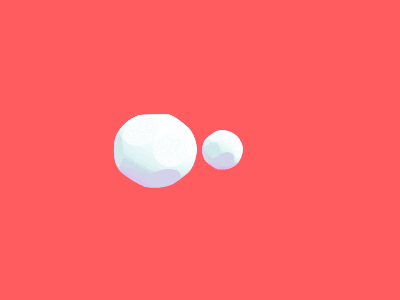
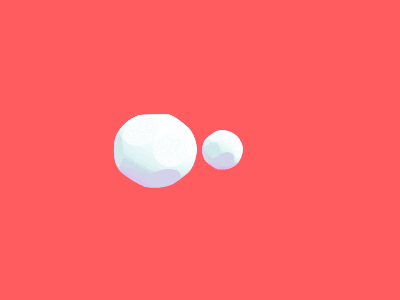
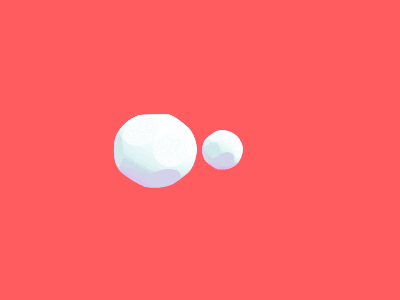
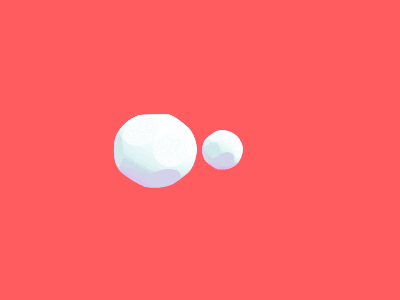
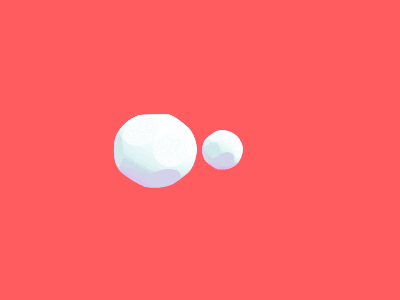
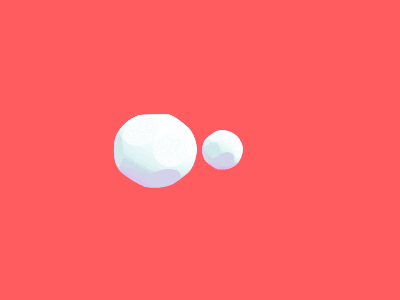
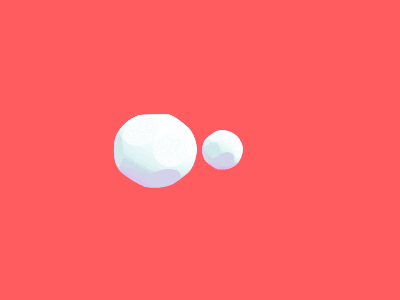