2022-02-21 更新
Iterative Learning for Instance Segmentation
Authors:Tuomas Sormunen, Arttu Lämsä, Miguel Bordallo Lopez
Instance segmentation is a computer vision task where separate objects in an image are detected and segmented. State-of-the-art deep neural network models require large amounts of labeled data in order to perform well in this task. Making these annotations is time-consuming. We propose for the first time, an iterative learning and annotation method that is able to detect, segment and annotate instances in datasets composed of multiple similar objects. The approach requires minimal human intervention and needs only a bootstrapping set containing very few annotations. Experiments on two different datasets show the validity of the approach in different applications related to visual inspection.
PDF 5 pages, 6 figures
论文截图
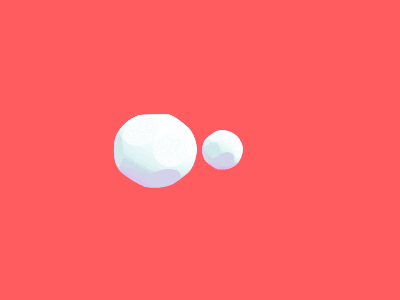
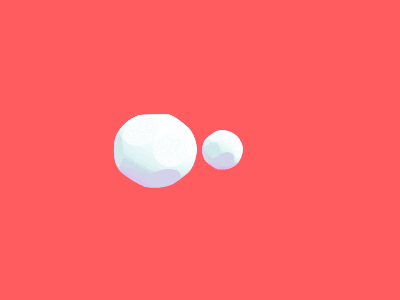
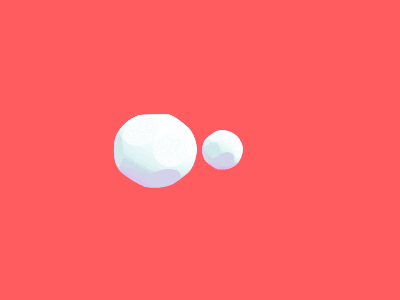
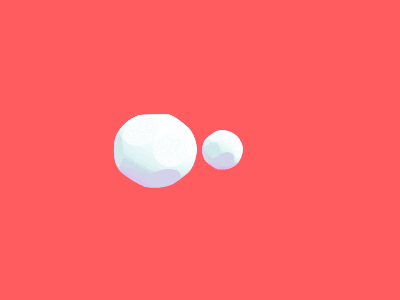
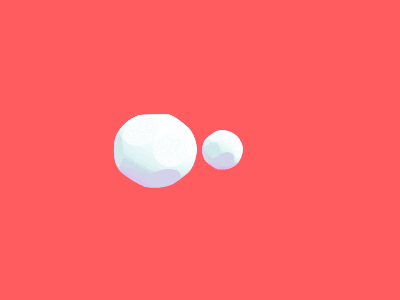
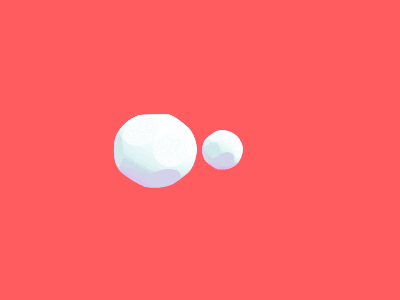
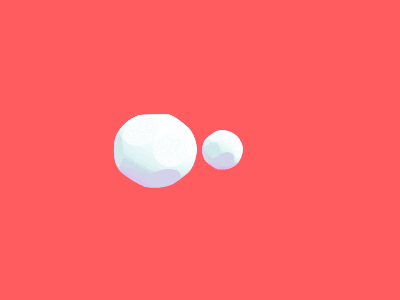
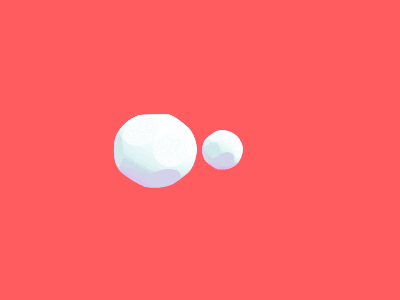
Decoupled Adaptation for Cross-Domain Object Detection
Authors:Junguang Jiang, Baixu Chen, Jianmin Wang, Mingsheng Long
Cross-domain object detection is more challenging than object classification since multiple objects exist in an image and the location of each object is unknown in the unlabeled target domain. As a result, when we adapt features of different objects to enhance the transferability of the detector, the features of the foreground and the background are easy to be confused, which may hurt the discriminability of the detector. Besides, previous methods focused on category adaptation but ignored another important part for object detection, i.e., the adaptation on bounding box regression. To this end, we propose D-adapt, namely Decoupled Adaptation, to decouple the adversarial adaptation and the training of the detector. Besides, we fill the blank of regression domain adaptation in object detection by introducing a bounding box adaptor. Experiments show that D-adapt achieves state-of-the-art results on four cross-domain object detection tasks and yields 17% and 21% relative improvement on benchmark datasets Clipart1k and Comic2k in particular.
PDF
论文截图
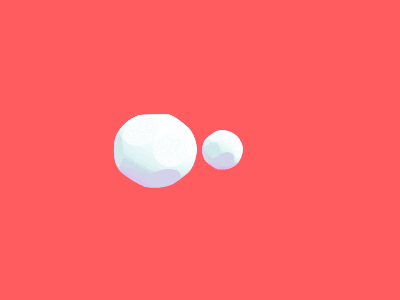
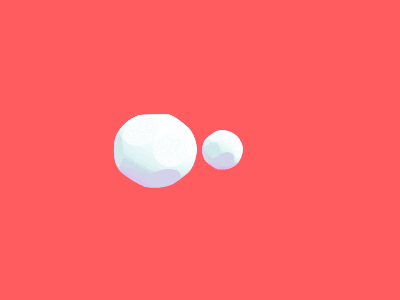
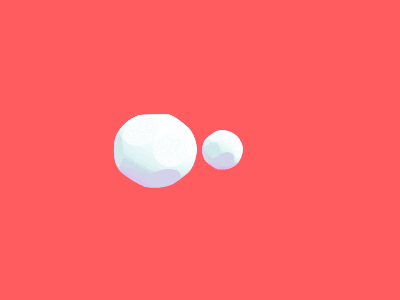
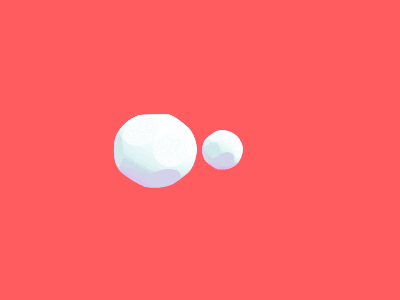
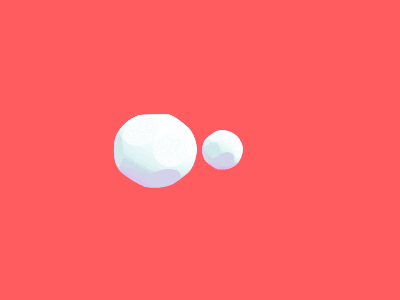
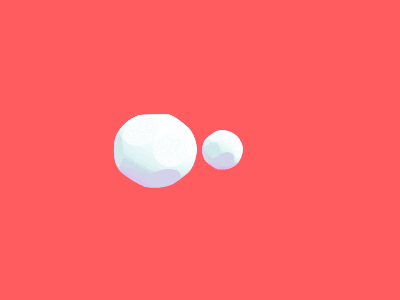
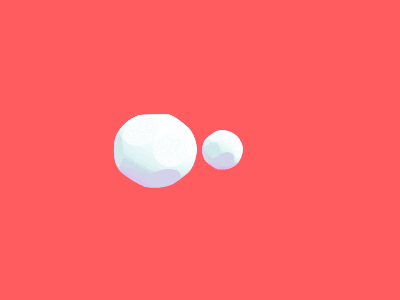
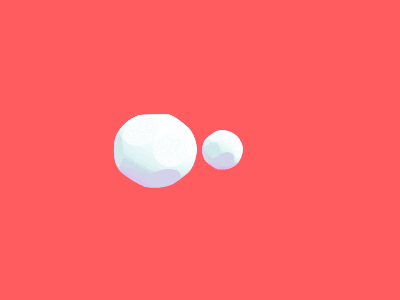