2023-03-20 更新
Configurable EBEN: Extreme Bandwidth Extension Network to enhance body-conducted speech capture
Authors:Julien Hauret, Thomas Joubaud, Véronique Zimpfer, Éric Bavu
This paper presents a configurable version of Extreme Bandwidth Extension Network (EBEN), a Generative Adversarial Network (GAN) designed to improve audio captured with body-conduction microphones. We show that these microphones significantly reduce environmental noise. However, this insensitivity to ambient noise is at the expense of the bandwidth of the voice signal acquired from the wearer of the devices. The obtained captured signals therefore require the use of signal enhancement techniques to recover the full-bandwidth speech. EBEN leverages a configurable multiband decomposition of the raw captured signal. This decomposition allows the data time domain dimensions to be reduced and the full band signal to be better controlled. The multiband representation of the captured signal is processed through a U-Net-like model, which combines feature and adversarial losses to generate an enhanced speech signal. We also benefit from this original representation in the proposed configurable discriminator architecture. The configurable EBEN approach can achieve state-of-the-art enhancement results on synthetic data with a lightweight generator that allows real-time processing.
PDF 12 pages, 10 figures, 3 tables, submitted to IEEE TASLP
点此查看论文截图
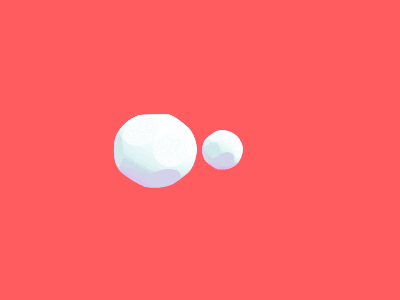
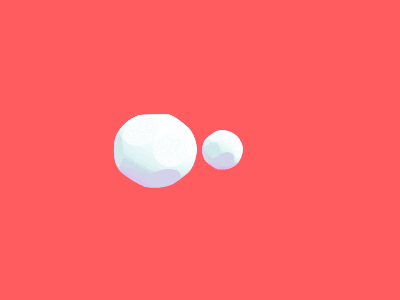
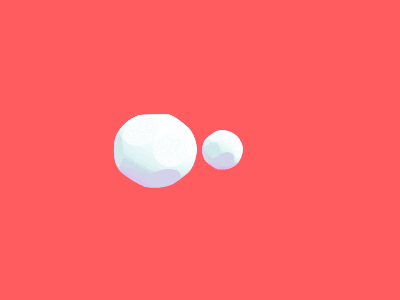
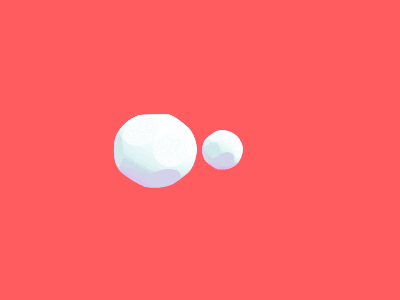
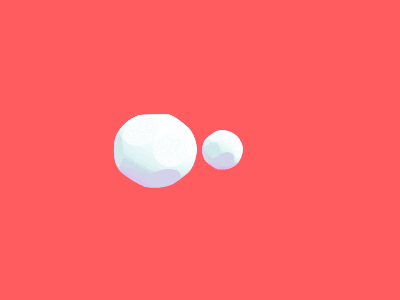
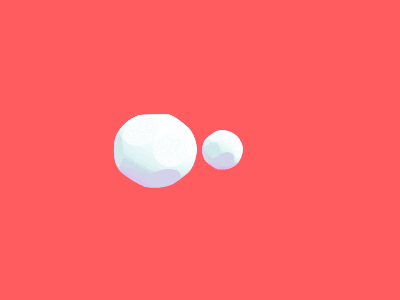
Visual Information Matters for ASR Error Correction
Authors:Vanya Bannihatti Kumar, Shanbo Cheng, Ningxin Peng, Yuchen Zhang
Aiming to improve the Automatic Speech Recognition (ASR) outputs with a post-processing step, ASR error correction (EC) techniques have been widely developed due to their efficiency in using parallel text data. Previous works mainly focus on using text or/ and speech data, which hinders the performance gain when not only text and speech information, but other modalities, such as visual information are critical for EC. The challenges are mainly two folds: one is that previous work fails to emphasize visual information, thus rare exploration has been studied. The other is that the community lacks a high-quality benchmark where visual information matters for the EC models. Therefore, this paper provides 1) simple yet effective methods, namely gated fusion and image captions as prompts to incorporate visual information to help EC; 2) large-scale benchmark datasets, namely Visual-ASR-EC, where each item in the training data consists of visual, speech, and text information, and the test data are carefully selected by human annotators to ensure that even humans could make mistakes when visual information is missing. Experimental results show that using captions as prompts could effectively use the visual information and surpass state-of-the-art methods by upto 1.2% in Word Error Rate(WER), which also indicates that visual information is critical in our proposed Visual-ASR-EC dataset
PDF Accepted at ICASSP 2023
点此查看论文截图
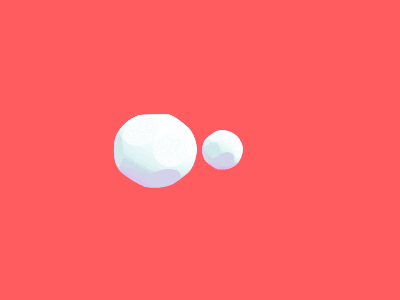
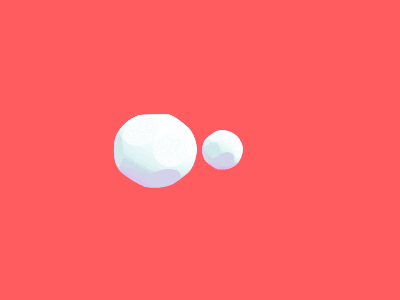
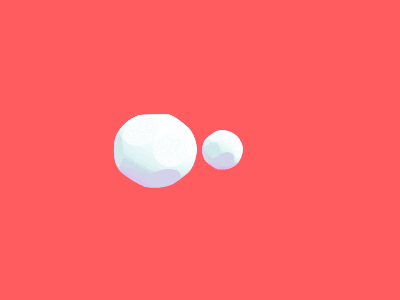
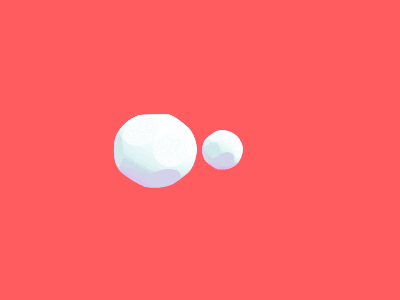
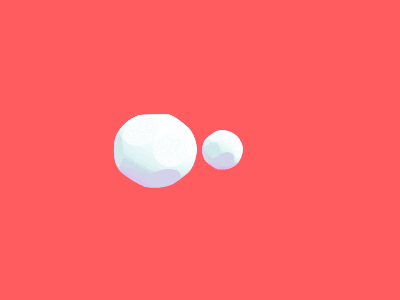
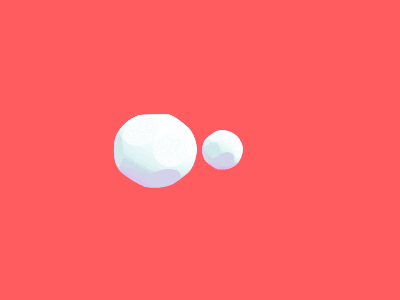